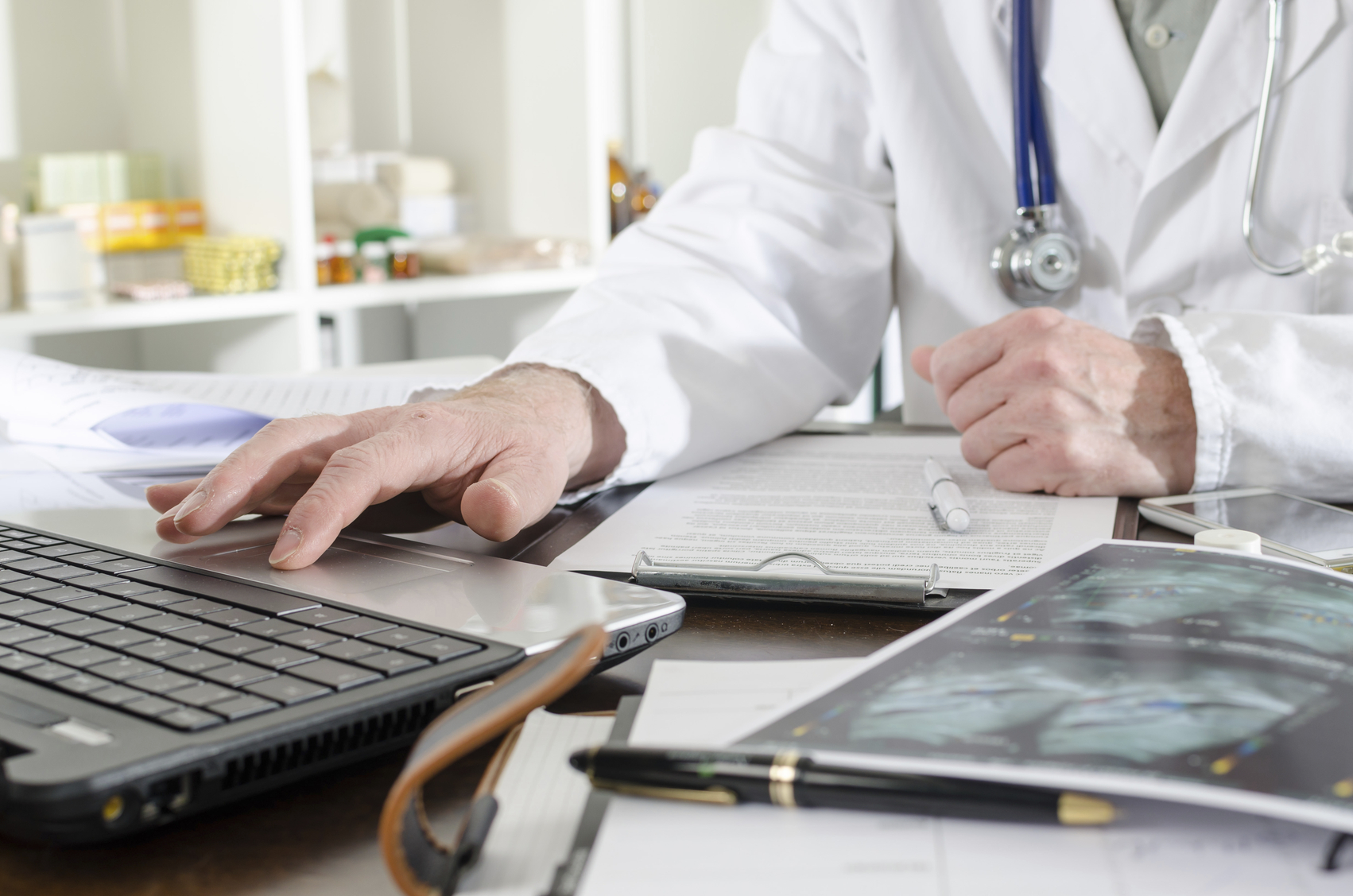
Asthma is one of the most common diseases in the world, but given the number of symptoms it shares with other conditions it is often misdiagnosed.
Recently, however, a team of Japanese researchers achieved 98 percent diagnostic accuracy using an AI-aided "Deep Neural Network" (DNN) tool that had been equipped with all patient information relevant to diagnosing adult asthma.
This topped the performance of two other AI-aided models using similarly thorough patient information, logistic analysis, with 94 percent accuracy, and support vector machine learning (SVM), with 82 percent accuracy.
The team also tested the three techniques using only physical symptoms presented by the 566 patients who made up their study cohort. Here the deep neural network still exceeded the other techniques, achieving 68 percent accuracy vs. 65 percent for logistic analysis and 60 percent for SVM learning.
According to the report, “the application of deep learning techniques currently has a very large advantage over SVM approaches in both accuracy and data handling. SVM approaches are an extremely popular and well-researched class of supervised learning models that do not use the computational power of deep learning.”
The full complement of patient information included not only physical symptoms but also biochemical findings, lung function test results and results from a bronchial challenge test.
For the study, the definitive diagnosis against which the models were checked came from asthma specialists working with all relevant patient data.
In their discussion, the researchers note that their study is probably the first in which computers performed nearly as well as human experts at diagnosing adult asthma.
“Our simple approach using deep neural networks is more accurate than conventional machine learning methods and can be employed to implement practical personal assistant services,” they wrote. “The next step [is] to persuade more hospitals to use the technology and investigate transferring the AI-based [approach] to primary care.”