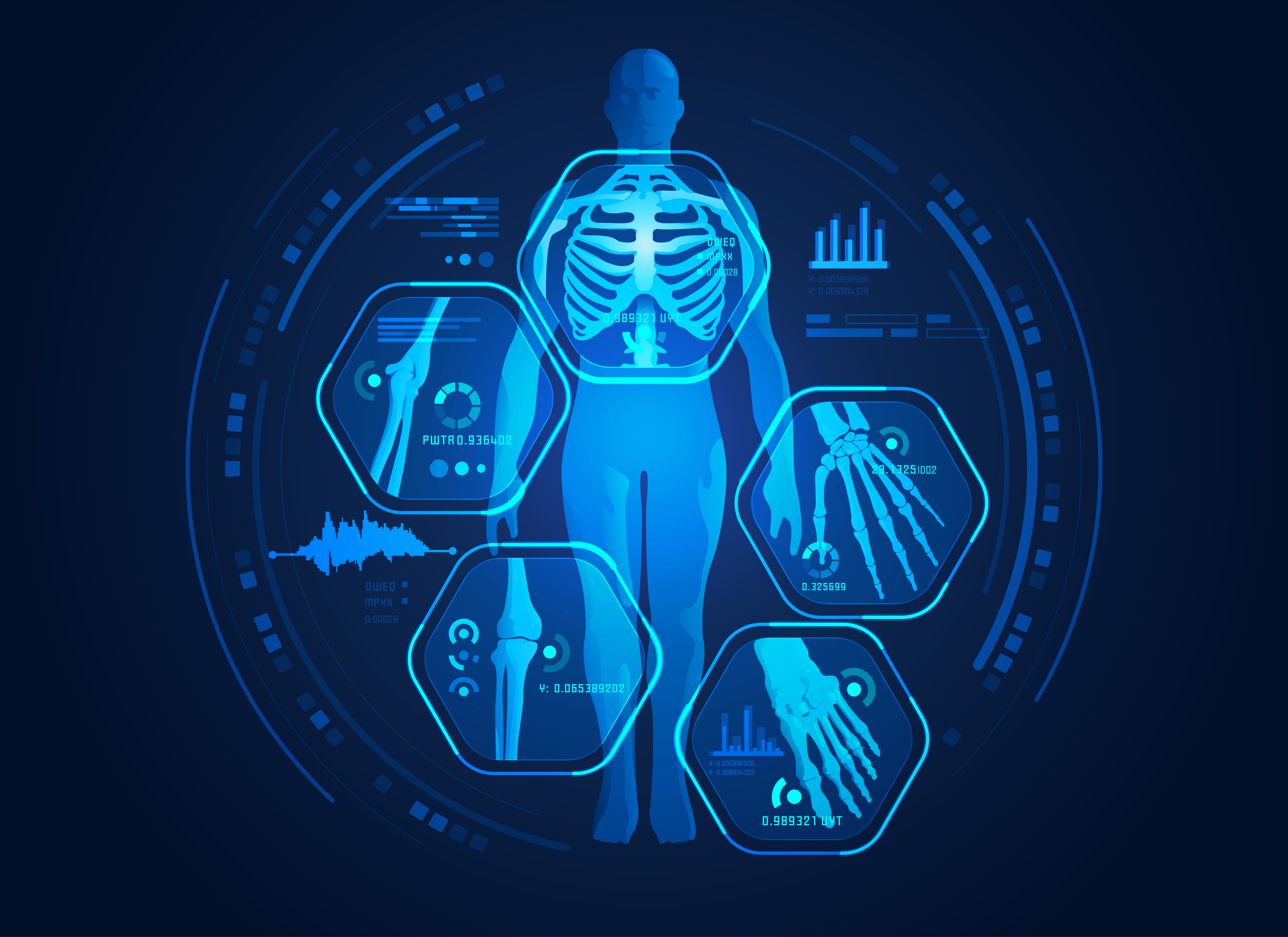
Damage in the joints of people with rheumatoid arthritis (RA) is currently measured by visual inspection and detailed scoring on radiographic images of small joints in the hands, wrists, and feet, but AI may be about to make the process easier and more accurate.
At the recent American College of Rheumatology (ACR) annual meeting, researchers lead by an investigator from New York’s Hospital for Special Surgery (HSS) rolled out the results of a crowdsourced effort to develop machine learning tools to qualify joint damage in individuals with RA. According to the researchers, the current scoring system requires specially trained experts and can be time-consuming and expensive, so finding an automated way to measure joint damage is important for both clinical research and for care of patients.
“If a machine-learning approach could provide a quick, accurate quantitative score estimating the degree of joint damage in hands and feet, it would greatly help clinical research,” the study’s senior author S. Louis Bridges, Jr., MD, PhD, said in a statement. “For example, researchers could analyze data from electronic health records and from genetic and other research assays to find biomarkers associated with progressive damage. Having to score all the images by visual inspection ourselves would be tedious, and outsourcing it is cost-prohibitive.”
Bridges added that the new machine learning method could also assist rheumatologists by quickly assessing damage progression over time and providing insight into treatment options. “This is really important in geographic areas where expert musculoskeletal radiologists are not available,” Bridges noted.
For the challenge, Bridges and his team partnered with Sage Bionetworks, a nonprofit organization that helps researchers develop DREAM (Dialogue on Reverse Engineering Assessment and Methods) Challenges, which focus on creating AI tools in the life sciences.
According to the team’s statement, “For the first part of the challenge, one set of images was provided to the teams, along with known scores that had been visually generated. These were used to train the algorithms. Additional sets of images were then provided so the competitors could test and refine the tools they had developed. In the final round, a third set of images was given without scores, and competitors estimated the amount of joint space narrowing and erosions. Submissions were judged according to which most closely replicated the gold-standard visually generated scores.”
Competitors were given 674 sets of images from 562 different RA patients who had participated in a previous National Institutes of Health research study.
Photo by Jackie Niam/Getty Images