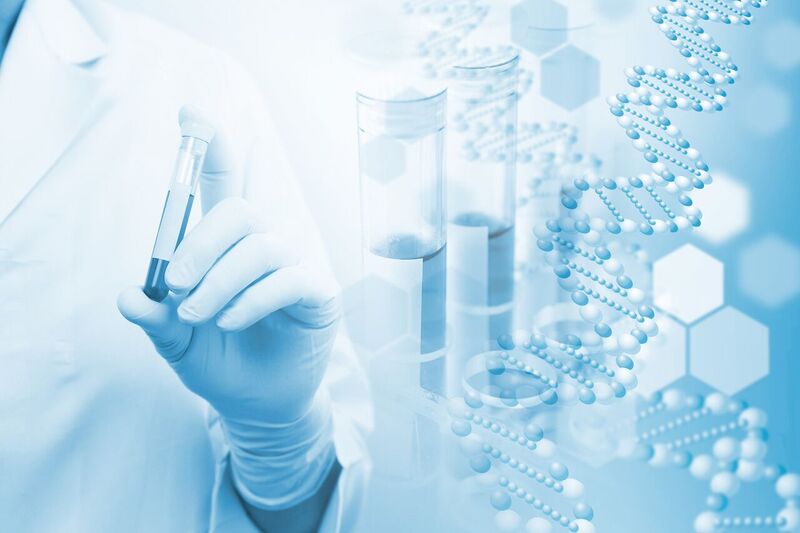
When examining biopsied tissue samples, time is often of the essence as work to make rapid, accurate diagnoses and determine appropriate treatments. To facilitate that process, engineers at UCLA recently tapped AI to develop a system that virtually re-stains tissue samples, reducing by hours the time needed to complete the process when performed by humans.
Under a microscope, pathologists examine the tissue samples from biopsies that have been stained with special dyes to enhance the color and contrast. While hematoxylin and eosin (H&E) are the most used stains, often in clinical cases additional special stains are necessary to increase contrast and color to different tissue components, allowing pathologists to get a clearer diagnostic picture.
The special stains provide a better diagnostic picture, but they can require much longer tissue preparations, increased monitoring, and a more expensive price tag. According to the researchers, the new, AI-driven process takes less than one minute per tissue sample, as opposed to the several hours when performed by human experts, thus allowing for faster preliminary diagnoses and, ideally, leading to quicker development of treatment options.
“We developed a deep learning-based technique that eliminates the need for special stains to be performed by histotechnologists,” said research lead Aydogan Ozcan, UCLA’s Volgenau Professor for Engineering Innovation at the Electrical and Computer Engineering Department of the Samueli School of Engineering and an associate director of the California NanoSystems Institute (CNSI), in a press release. “The enhanced speed and accuracy are particularly important when diagnosing medical conditions such as organ transplant rejection cases, where a fast and accurate diagnosis enables rapid treatment, which may lead to greatly improved clinical outcomes.”
Ozcan and team demonstrated the AI-based technique by generating a full panel of special stains used for kidney tissue. By using specialized deep neural networks trained on images of H&E-stained tissue biopsies, the research team virtually generated the special stains on several clinical samples, covering a broad range of kidney diseases.
“A multi-institution team of board-certified renal pathologists then performed a clinical evaluation to ascertain the efficacy of the virtual, stain-to-stain transformation technique. They found a statistically significant improvement in the diagnoses achieved by using the virtually generated special stains over the use of only the H&E-stained images of biopsies,” the team stated in their release.
Moreover, they noted, since the technique is applied to existing H&E-stained images it would be easy to adopt, as it does not require any changes to the current tissue processing workflow used in pathology labs.
A full report has been published at Nature Communications.
Photo by Ca-ssis/Getty Images