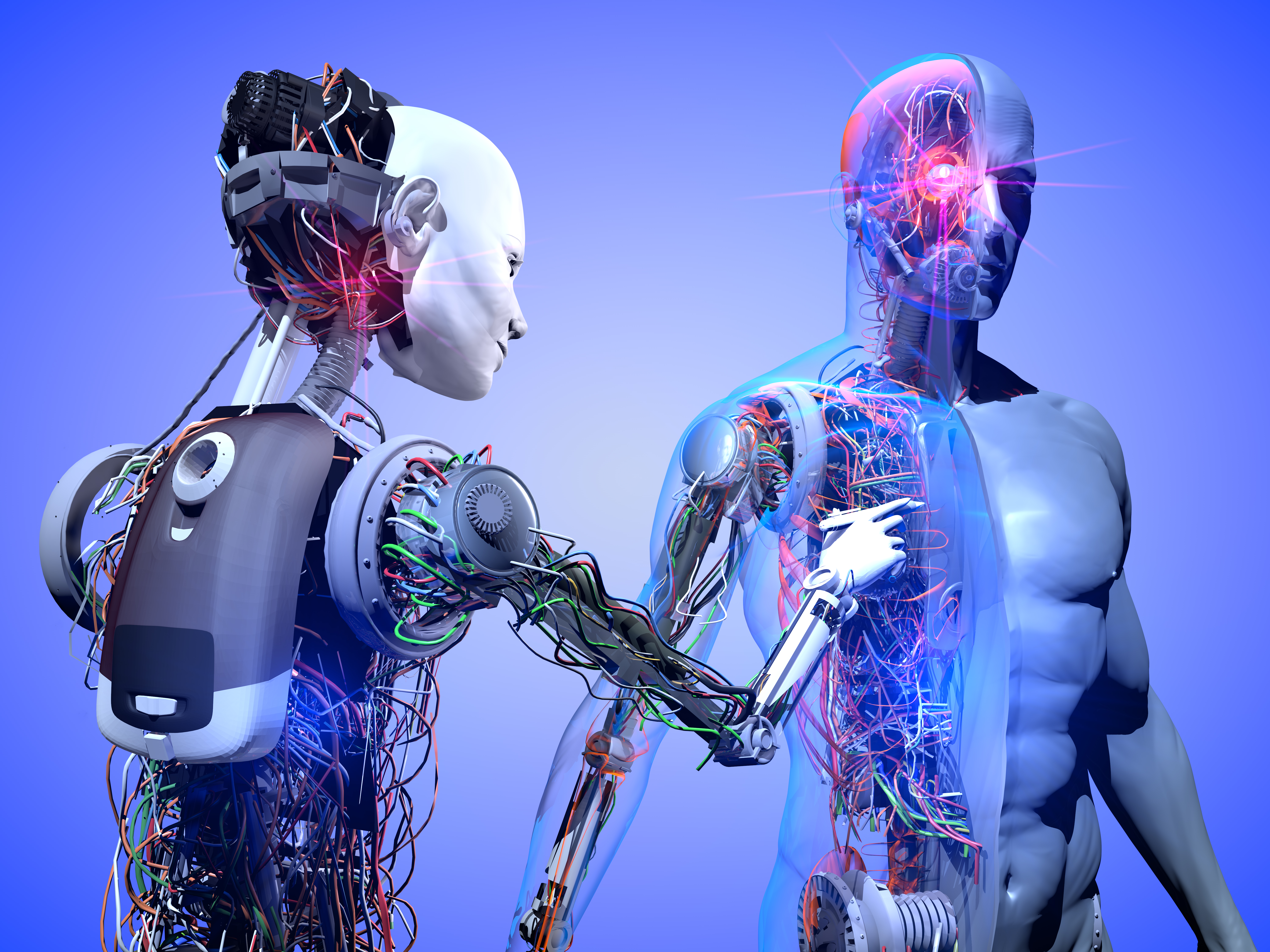
While prosthetics have undoubtedly been a boon for countless users, uneven or variable terrain has long been a challenge. But engineers at North Carolina State University have come up with a potential solution.
According to a release from the University, researchers have developed new software that can be integrated with existing hardware to enable people using robotic prosthetics or exoskeletons to walk in a safer, more natural manner on different types of terrain. The new framework incorporates computer vision into prosthetic leg control, and includes robust AI algorithms that allow the software to better account for uncertainty
Currently, lower limb robotic prosthetics will act differently depending on the terrain, but when uncertainties are encountered, the robotic limbs will often default into ‘safe mode’.
As Edgar Lobaton, co-author of a paper on the work and an associate professor of electrical and computer engineering at North Carolina State University, summed it up, “Lower-limb robotic prosthetics need to execute different behaviors based on the terrain users are walking on. The framework we’ve created allows the AI in robotic prostheses to predict the type of terrain users will be stepping on, quantify the uncertainties associated with that prediction, and then incorporate that uncertainty into its decision-making.”
The outcome is safer walking for robotic limb users.
“We came up with a better way to teach deep-learning systems how to evaluate and quantify uncertainty in a way that allows the system to incorporate uncertainty into its decision making,” Lobaton explained. “This is certainly relevant for robotic prosthetics, but our work here could be applied to any type of deep-learning system. We found that the model can be appropriately transferred so the system can operate with subjects from different populations. That means that the AI worked well even though it was trained by one group of people and used by somebody different."
The researchers trained the AI by attaching cameras to able-bodied people who walked on different terrains. This was followed with an evaluation of a person with lower-limb amputation wearing the cameras whilst walking across the same environments. Incorporating a camera onto the limb and a set of glasses allowed the AI to utilize ‘computer vision’ data from both cameras.
The researchers focused on distinguishing between six different terrains that require adjustments in a robotic prosthetic’s behavior: tile, brick, concrete, grass, “upstairs” and “downstairs.”
“If the degree of uncertainty is too high, the AI isn’t forced to make a questionable decision,” noted Boxuan Zhong, lead author of the paper and a recent Ph.D. graduate from NC State. “It could instead notify the user that it doesn’t have enough confidence in its prediction to act, or it could default to a ‘safe’ mode.”
The paper, “Environmental Context Prediction for Lower Limb Prostheses with Uncertainty Quantification,” is published in IEEE Transactions on Automation Science and Engineering. The paper was co-authored by Rafael da Silva, a Ph.D. student at NC State; and Minhan Li, a Ph.D. student in the Joint Department of Biomedical Engineering.