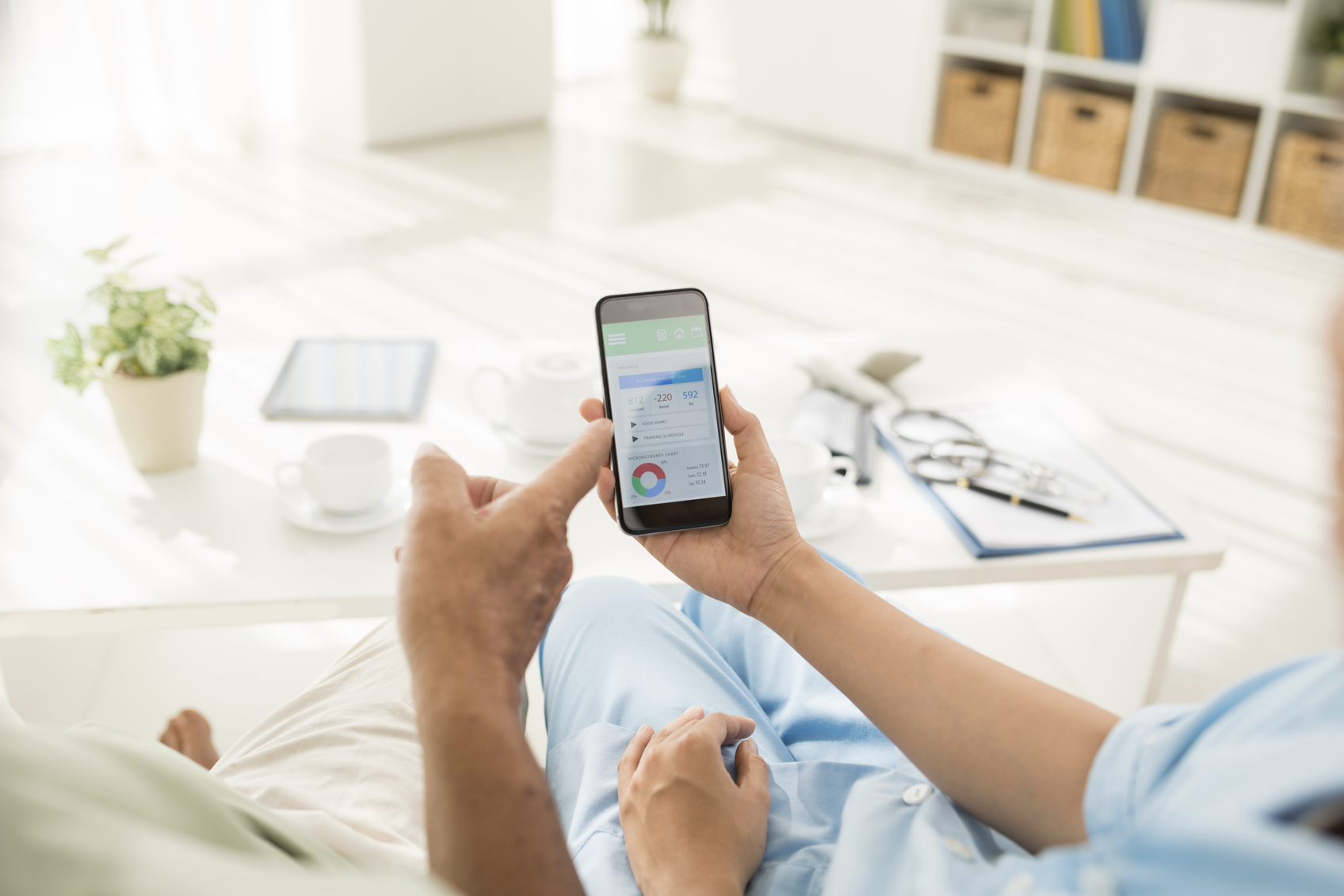
How will a chronic disease progress as a patient ages?
That's the question researchers at the University of Buffalo have recently been asking, and they've tapped AI to help them find answer.
As described in a recent report in the Journal of Pharmacokinetics and Pharmacodynamics, the team develop a model that assesses metabolic and cardiovascular biomarkers – measurable biological processes such as cholesterol levels, body mass index, glucose and blood pressure – to calculate health status and disease risks across a patient’s lifespan.
“There is an unmet need for scalable approaches that can provide guidance for pharmaceutical care across the lifespan in the presence of aging and chronic co-morbidities,” said lead author Murali Ramanathan, PhD, professor of pharmaceutical sciences in the UB School of Pharmacy and Pharmaceutical Sciences, in a statement accompanying the release of the report. “This knowledge gap may be potentially bridged by innovative disease progression modeling.”
According to the team, the findings are important given the increased risk of developing metabolic and cardiovascular diseases with aging, a process that has adverse effects on cellular, psychological and behavioral processes.
Using artificial intelligence, a team of University at Buffalo researchers has developed a novel system that models the progression of chronic diseases as patients age.
For the study, the team examined data from three case studies within the third National Health and Nutrition Examination Survey (NHANES) that assessed the metabolic and cardiovascular biomarkers of nearly 40,000 people in the United States. Including measurements such as temperature, body weight and height, biomarkers are used to diagnose, treat and monitor overall health and numerous diseases.
The researchers examined seven metabolic biomarkers: body mass index, waist-to-hip ratio, total cholesterol, high-density lipoprotein cholesterol, triglycerides, glucose and glycohemoglobin. The cardiovascular biomarkers examined include systolic and diastolic blood pressure, pulse rate and homocysteine. By analyzing changes in metabolic and cardiovascular biomarkers, the model “learns” how aging affects these measurements. Via machine learning, the system used a memory of previous biomarker levels to predict future measurements, which ultimately reveal how metabolic and cardiovascular diseases progress over time.
Prof. Ramanathan said the model could facilitate the assessment of long-term chronic drug therapies, and help clinicians monitor treatment responses for conditions such as diabetes, high cholesterol and high blood pressure, which become more frequent with age.
Photo by DragonImages/Getty Photo