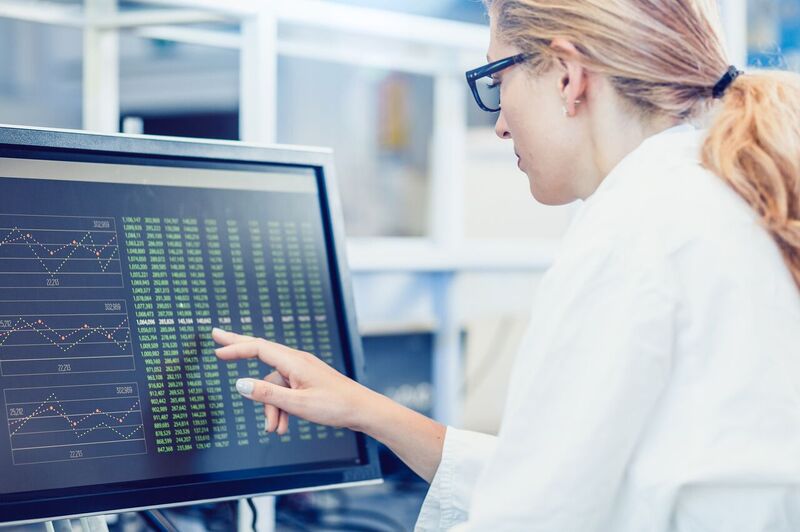
While the American Medical Informatics Association (AMIA) supports the steps taken thus far by the Food and Drug Administration (FDA) to develop effective guidelines for regulating AI-driven medical devices, the organizations wants the federal agency to continue to improve its overall conceptual approach to the new technology.
In comments sent recently to the FDA, AMIA recommended improvements in four areas: continuously learning versus “locked” algorithms; new data inputs’ impact on algorithms’ outputs; cybersecurity in the context of AI/ML-based SaMD; and evolving knowledge about algorithm-driven bias.
“Properly regulating AI and machine learning-based SaMD will require ongoing dialogue between FDA and stakeholders,” said AMIA President and CEO Douglas Fridsma, MD. “This draft framework is only the beginning of a vital conversation to improve both patient safety and innovation. We certainly look forward to continuing it.”
The FDA sees tremendous potential in healthcare for AI algorithms that continually evolve—dubbed “adaptive” or “continuously learning” algorithms—and don’t need manual modification to incorporate learning or updates.
But when it comes to learning versus locked algorithms, AMIA told the FDA that “while the framework acknowledges the two different kinds of algorithms” it is concerned that the framework is “rooted in a concept that both locked and continuously learning SaMD provides opportunity for periodic, intentional updates.”
Moreover, the group said it is “concerned that a user of SaMD in practice would not have a practical way to know whether the device reasonably applied to their population, and therefore, whether adapting to data on their population would be likely to cause a change based on the SaMD’s learning.”
In addition, AMIA claimed that the framework “fails to discuss how modifications to SaMD algorithms may be the result of breaches of cybersecurity and the need to make this a component of periodic evaluation” and that the FDA should “consider how cybersecurity risks, such as hacking or data manipulation that may influence the algorithm’s output, may be addressed in a future version of the framework.”
A further critical question, the AMIA letter noted, concerns “the extent to which an AI-based SaMD should be able to furnish explanatory reasoning for any decision it provides or supports. In the classical form of AI, where existing expertise has been encoded, it is possible to have a chain of reasoning back to principles or data. Machine learning algorithms, however, may function in a ‘black box’ mode, with inputs modifying the implicit circuitry with no clear traceability. It is thus vital to consider under what circumstances an AI-based SaMD should provide explanation of any decision it offers.”
Finally, the group recommended that the agency develop guidance about how and how often developers of SaMD-based products test their products for algorithm-driven biases.