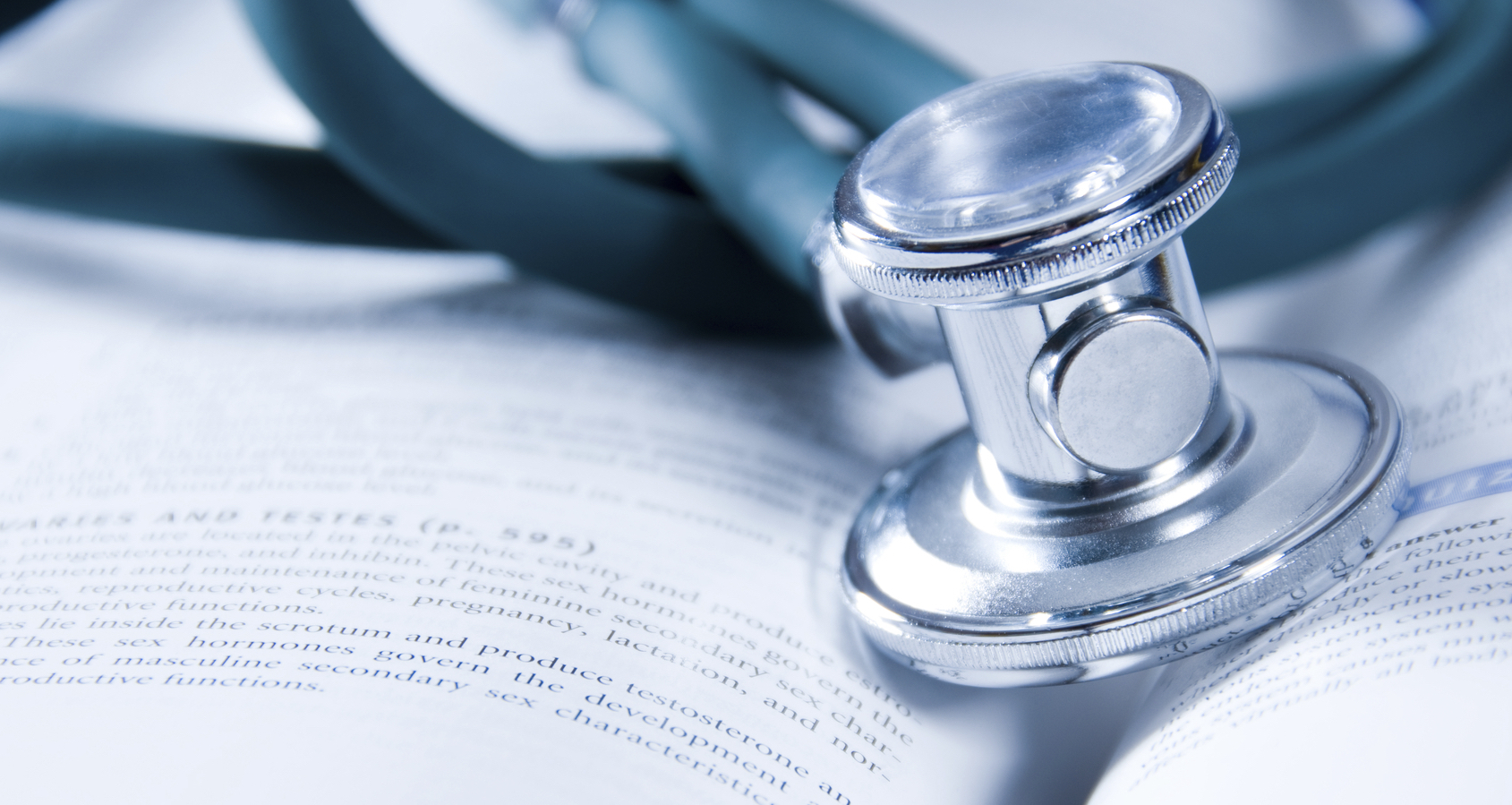
As it has across the healthcare sector, “equity” has been a top concern among developers and promoters of AI.
In a recent commentary, for example, the Pew Charitable Trusts’ Liz Richardson, director the organization’s health care products project, urged the FDA to consider health equity more explicitly in its regulatory approval process.
“The agency could do so under its current authorities and has a range of regulatory tools to help ensure AI-enabled products do not reproduce or amplify existing inequalities within the healthcare system,” she argues.
While Richardson concedes that many health-focused AI tools fall outside the FDA’s authority, she notes that “since 2015 the agency has approved or cleared more than 100 medical devices that rely on AI, including a handful used to screen COVID-19 patients. The potential algorithmic biases posed by such products can affect health equity, a principle suggesting that disparities in health outcomes caused by factors such as race, income, or geography should be addressed and prevented.”
After describing a number of ways bias can inadvertently end up in approved AI, Richardson argues that “(d)uring premarket review, FDA can help mitigate the risks of bias by routinely analyzing the data submitted by AI software developers by demographic subgroup, including sex, age, race, and ethnicity. This would help gauge how the product performed in those populations and whether there were differences in effectiveness or safety based on these characteristics. The agency also could choose to reject a product’s application if it determined, based on the subgroup analysis, that the risks of approval outweighed the benefits.”
Another step FDA could take, according to Richardson, is by requiring “clear labeling about potential disparities in product performance” based on potential bias in the AI’s development. “For example, if an applicant seeks FDA approval for an AI-enabled tool to detect skin cancer by analyzing patient images, but has not tested it on a racially diverse group of images, the agency could require the developer to note this omission in the labeling. This would alert potential users that the product could be inaccurate for some patient populations and may lead to disparities in care or outcomes. Providers can then take steps to mitigate that risk or avoid using the product.”
The good news, says Richardson, is that ‘AI can help patients from historically underserved populations by lowering costs and increasing efficiency in an overburdened health system. But the potential for bias must be considered when developing and reviewing these devices to ensure that the opposite does not occur. By analyzing subpopulation-specific data, calling out potential disparities on product labels, and pushing internally for the prioritization of equity in its review process, FDA can prevent potentially biased products from entering the market and help ensure that all patients receive the high-quality care they deserve.”
Photo by YanC/Getty Images