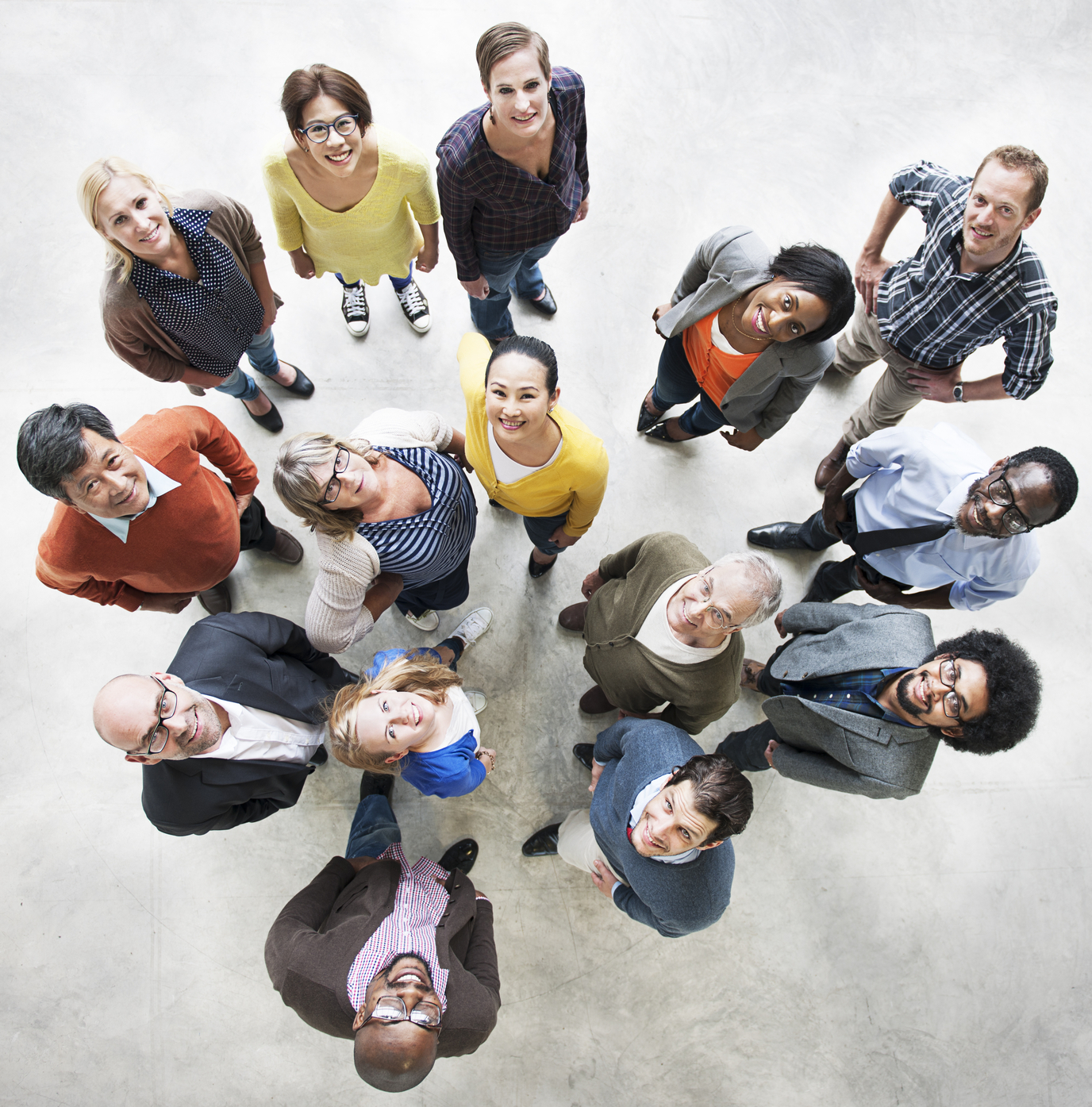
While AI is being incorporated into the battle against a range of diseases and conditions, it has yet to be adequately enrolled in either research or the development of new therapies needed to meet the growing global demand for hearing care.
That’s according to a team of experts from the UK and US in a recent commentary published in the journal Nature Machine Intelligence.
Hearing was once at the forefront of technological innovation, the team noted, “but innovation has stalled, and hearing healthcare is struggling to meet a growing global burden; the vast majority of those with hearing loss do not receive treatment, and those who do often receive only limited benefit.”
In their view the disconnect between AI and hearing has deep roots. “In contrast to modern machine vision, which began with the explicit goal of mimicking the visual cortex and continues to draw inspiration from the visual system, work in modern machine hearing has never prioritized biological links,” they explained.
While the team said the recent incorporation of deep neural networks (DNNs) into machine hearing systems has further improved their performance in specific tasks, it has not brought machine hearing any closer to the auditory system in a mechanistic sense. “Biological replication is not necessarily a requirement: many of the important clinical challenges in hearing can be addressed using models with no relation to the auditory system . . . But for the full potential of AI in hearing to be realized, new machine hearing systems that match both the function of the auditory system and key elements of its structure are needed.”
In their paper, the scientists first explain the scope of the need for improved hearing healthcare. “Hearing disorders are a leading cause of disability, affecting approximately 500 million people worldwide and costing nearly US$750 billion annually. The current care model, which is heavily reliant on specialized equipment and labour-intensive clinician services, is failing to cope: approximately 80% of those who need treatment are not receiving it.”
That said, “many of the most pressing problems in hearing healthcare can be framed as classification or regression problems that can be solved by training existing AI technologies on the appropriate clinical datasets.”
For example, they note, “the most promising use of AI in hearing devices is in replicating or enhancing functions that are normally performed by the auditory system. By using DNNs to transform incoming sounds, AI could dramatically improve the signal processing in hearing devices. This approach is particularly well suited to address the most common problem reported by device users: difficulty understanding speech in a setting with multiple talkers or substantial background noise (the so-called cocktail party problem). Recent work has already demonstrated that DNNs can improve the understanding of speech in noise for device users.”
In short, they argue, “(o)ngoing collaboration between AI researchers and hearing researchers would create a win–win situation for both communities and also help to ensure that new technologies are well matched to the needs of users. The computational strategies implemented by the ear and brain evolved over many millennia under strong pressure to be highly effective and efficient. Thus, new AI tools modelled after the auditory system have the potential to be transformative not only for hearing but also for other domains in which efficient and adaptive multi-scale, multi-modality and multi-task capabilities are critical.”
Photo by Rawpixel/Getty Images