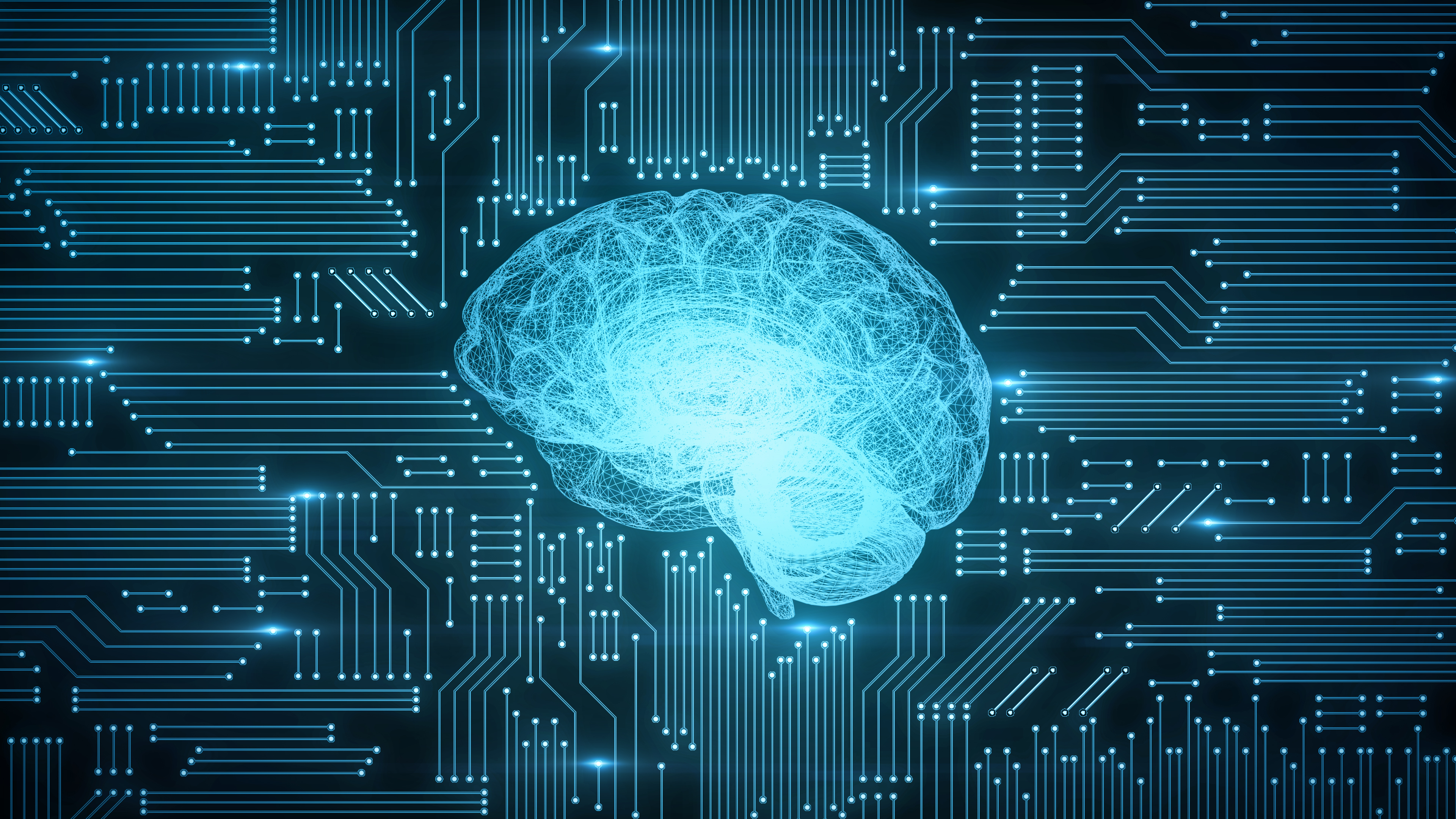
Schizophrenia is a disease that is at once devastating and difficult to explain, say experts, but researchers at Baylor College of Medicine have recently announced a new AI-enabled test that may help healthcare providers improve how they identify and treat the condition that affects roughly 1% of the world’s population.
According to their press release, the researchers have developed a machine learning algorithm called SPLS-DA to look for epigenetic markers for schizophrenia. By analyzing DNA from blood samples, the team was able to identify the epigenetic markers – epigenetics being a system for DNA molecular marking that “instructs” different body cells which genes to turn on or off – that differed between people diagnosed with schizophrenia and those without. The team then developed a model that could use predictive analytics to determine the probability of an individual having schizophrenia.
“Although genetic and environmental components seem to be involved in the condition, current evidence only explains a small portion of cases, suggesting that other factors, such as epigenetic, also could be important,” explained corresponding author Dr. Robert A. Waterland in the release.
Adding to the complexity is the fact that epigenetic markers can vary between different normal tissues within an individual, making it difficult for researchers to determine if epigenetic changes contribute to diseases involving the brain.
To address this obstacle, Waterland and his colleagues referenced previous work that had identified specific genomic regions in which DNA methylation, a common epigenetic marker, differs between people but is consistent across different tissues in one person. They called these genomic regions CoRSIVs for correlated regions of systemic interindividual variation. They proposed that studying CoRSIVs is a novel way to uncover epigenetic causes of disease.
“Because methylation patterns in CoRSIVs are the same in all the tissues of one individual, we can analyze them in a blood sample to infer epigenetic regulation on other parts of the body that are difficult to assess, such as the brain,” said Waterland, who is also a professor of pediatrics – nutrition at the USDA/ARS Children’s Nutrition Research Center at Baylor and of molecular and human genetics.
“Our study is innovative in various ways,” said first author Dr. Chathura J. Gunasekara, a computer scientist in the Waterland lab. “We focused on CoRSIVs and also applied for the first time the SPLS-DA machine learning algorithm to analyze DNA methylation. As a scientist interested in applying machine learning to medicine, our findings are very exciting. They not only suggest the possibility of predicting risk of schizophrenia early in life, but also outline a new approach that may be applicable to other diseases.”
Photo by wigglestick/Getty Images