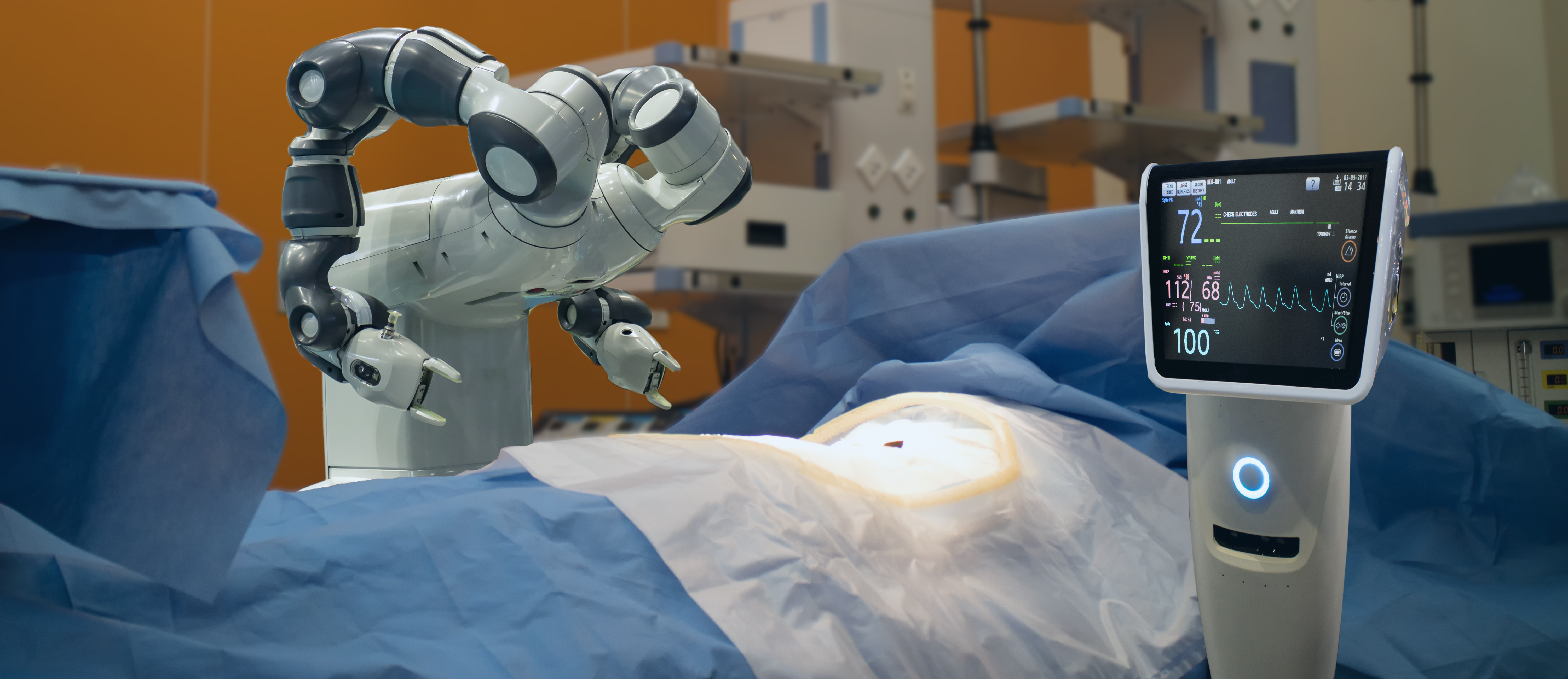
While anesthesiologists have traditionally relied on patient metrics such as heart and respiratory rate to determine when surgery patients are at the desired level of unconsciousness, new machine learning algorithms can evaluate unconsciousness in patients based on brain activity.
That’s according to a new study published in Plos One by a team of researchers from MIT and Massachusetts General Hospital (MGH).
“One of the things that is foremost in the minds of anesthesiologists is 'Do I have somebody who is lying in front of me who may be conscious and I don't realize it?'” said senior author Emery N. Brown, Edward Hood Taplin Professor in The Picower Institute for Learning and Memory and the Institute for Medical Engineering and Science at MIT, and an anesthesiologist at MGH, in an article describing the study. “Being able to reliably maintain unconsciousness in a patient during surgery is fundamental to what we do. This is an important step forward.”
The new algorithms may also offer anesthesiologists the opportunity to maintain unconsciousness at the desired level while using less drug than they might administer otherwise, a development which could improve patients’ post-operative outcomes such as delirium.
“We may always have to be a little bit 'overboard',” said Brown, who is also a professor at Harvard Medical School. “But can we do it with sufficient accuracy so that we are not dosing people more than is needed?”
For the study, researchers trained machine learning algorithms on a dataset collected in 2013 in ten healthy volunteers underwent anesthesia with a commonly used drug, propofol. As the dose was methodically raised using computer-controlled delivery, researchers asked volunteers to respond to a simple request until they couldn’t anymore.
When they were brought back to consciousness as the dose was later lessened, they were able to respond again. As the doses were increasing and decreasing, the team recorded neural rhythms reflecting their brain activity using electroencephalogram (EEG) electrodes. This provided a direct, real-time link between measured brain activity and exhibited unconsciousness.
For the new study, the team trained versions of their AI algorithms on more than 33,000 two-second-long snippets of EEG recordings from seven of the volunteers, thus enabling the algorithms to learn the difference between EEG readings predictive of consciousness and unconsciousness under anesthesia.
The group then used the algorithms to analyze EEG recorded from 27 real surgery patients who received propofol for general anesthesia. The algorithms were able to distinguish unconsciousness with a higher level of accuracy than other studies have shown.
The team is working to refine the algorithms, as well as to expand testing to more cases for confirmation of their findings.