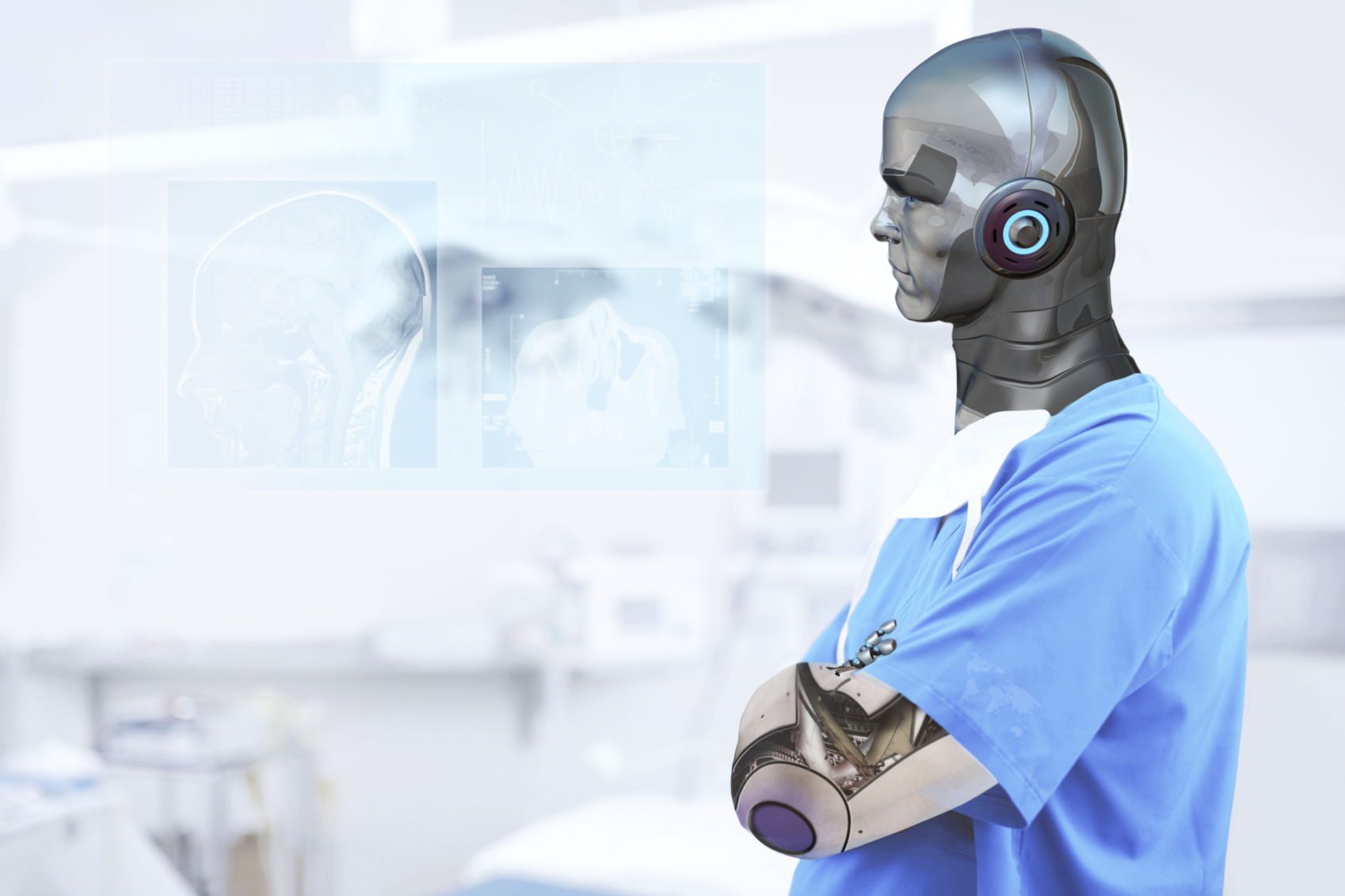
AI has been a welcome introduction to healthcare for, among many other reasons, its capacity to analyze huge troves of data in a fraction of the time required by human researchers. But one of the ongoing concerns has been the inability of researchers to consistently determine how AI algorithms reached specific decisions.
In what is being hailed as a breakthrough on that front, researchers from Charité University Hospital in Berlin, the Berlin Institute of Technology and the University of Oslo have unveiled a breast cancer diagnosis system that could help clinicians understand the rationale behind AI decisions.
According to a report the researchers have published in Nature Machine Intelligence, the system uses heatmaps to show what visual information influenced the AI decision process as well as to what extent, thus enabling doctors to understand and assess the plausibility of the results. In addition, for the first time, morphological, molecular and histological data are integrated in a single analysis.
As explained by Prof. Frederick Klauschen, M.D., of Charité's Institute of Pathology, cancer treatment is increasingly concerned with the molecular characterization of tumor tissue samples. But while microscopic techniques enable biological processes to be studied with high spatial detail, they only permit a limited measurement of molecular markers. As a result, spatial detail is not possible and the relationship between these markers and the microscopic structures is typically unclear.
"We know that in the case of breast cancer, the number of immigrated immune cells, known as lymphocytes, in tumor tissue has an influence on the patient's prognosis. There are also discussions as to whether this number has a predictive value - in other words if it enables us to say how effective a particular therapy is," said Dr. Klauschen.
Added fellow researcher Prof. Klaus-Robert Müller, M.D., professor of machine learning at TU Berlin, ”The problem we have is the following: We have good and reliable molecular data and we have good histological data with high spatial detail. What we don't have as yet is the decisive link between imaging data and high-dimensional molecular data.”
Ideally, the approach the researchers describe in the report will facilitate the development of that link.
"Our system facilitates the detection of pathological alterations in microscopic images. Parallel to this, we are able to provide precise heatmap visualizations showing which pixel in the microscopic image contributed to the diagnostic algorithm and to what extent," explained Müller.
The research team has also succeeded in significantly further developing this process: "Our analysis system has been trained using machine learning processes so that it can also predict various molecular characteristics, including the condition of the DNA, the gene expression as well as the protein expression in specific areas of the tissue, on the basis of the histological images.