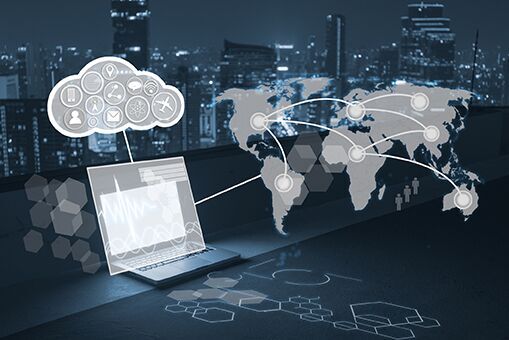
While AI is increasingly used to sharpen the cutting edge of healthcare diagnosis and delivery in the wealthier countries of the world, in lower income countries it’s doing all that plus helping doctors do more with limited resources while also spreading the range of delivery of care.
Writing recently in the Financial Times, Darlington Akogo, founder and executive director of minoHealth AI Labs, a Ghana-based AI for health start-up, outlined his company’s efforts to automate radiology through the use of deep learning.
“Using an algorithm called a convolutional neural network — a form of deep learning designed to emulate the way vision works in animals — we have been able to develop image recognition tools to help diagnose conditions including breast cancer, pneumonia, fibrosis and hernia,” he explained. We are exploring additional applications in neuroscience, optometry, oncology, epidemiology and nutrition. We have developed systems to study and identify differences between populations with high and low immunity to malaria, which could help develop more effective vaccines. We are researching digital diagnostics to speed up the detection of infectious diseases.”
To realize the full potential of AI in lower and middle income countries, however, Akogo points to five barriers that need to be overcome.
First, proper digital infrastructure. Too often, he says, “patient records are poorly managed, with medical (centers) often handing over test results to patients or other facilities without keeping a copy. This limits the data available to help develop and assess AI systems.”
Next, he points to the need for a “data culture” in which health facilities value information and make the tools necessary for data use available to clinicians. To that end, “(g)overnments and health agencies should encourage health facilities and providers to adopt suitable clinical information systems — which are comprehensive and compatible — and support training for data-driven healthcare,” and, third, they also need to step up efforts to develop appropriate regulations and standards for AI and data science.
The final two efforts Akogo urges include the development of local standards and, not surprisingly, more funding.
Concerning the former, he notes that while many countries rely on the use of western digital health technologies, “(l)ocal developers are better able to understand and find appropriate solutions while fostering self-reliance and helping to nurture the local ecosystem.”
As for funding, Akogo points to the need for more targeted investment for AI health start-ups in Africa, and he says “(i)nternational development agencies should redirect funding from public universities to start-ups, helping lower the risk for private investors to follow. Donors must not simply impose solutions, but instead encourage proposals to meet broad gaps and needs.”
And the results that await? Predicts Akogo, “by bringing together local, regional and global physicians, researchers, academics, entrepreneurs and regulators, societies with poor and expensive healthcare systems alike could see a democratization of quality healthcare.”