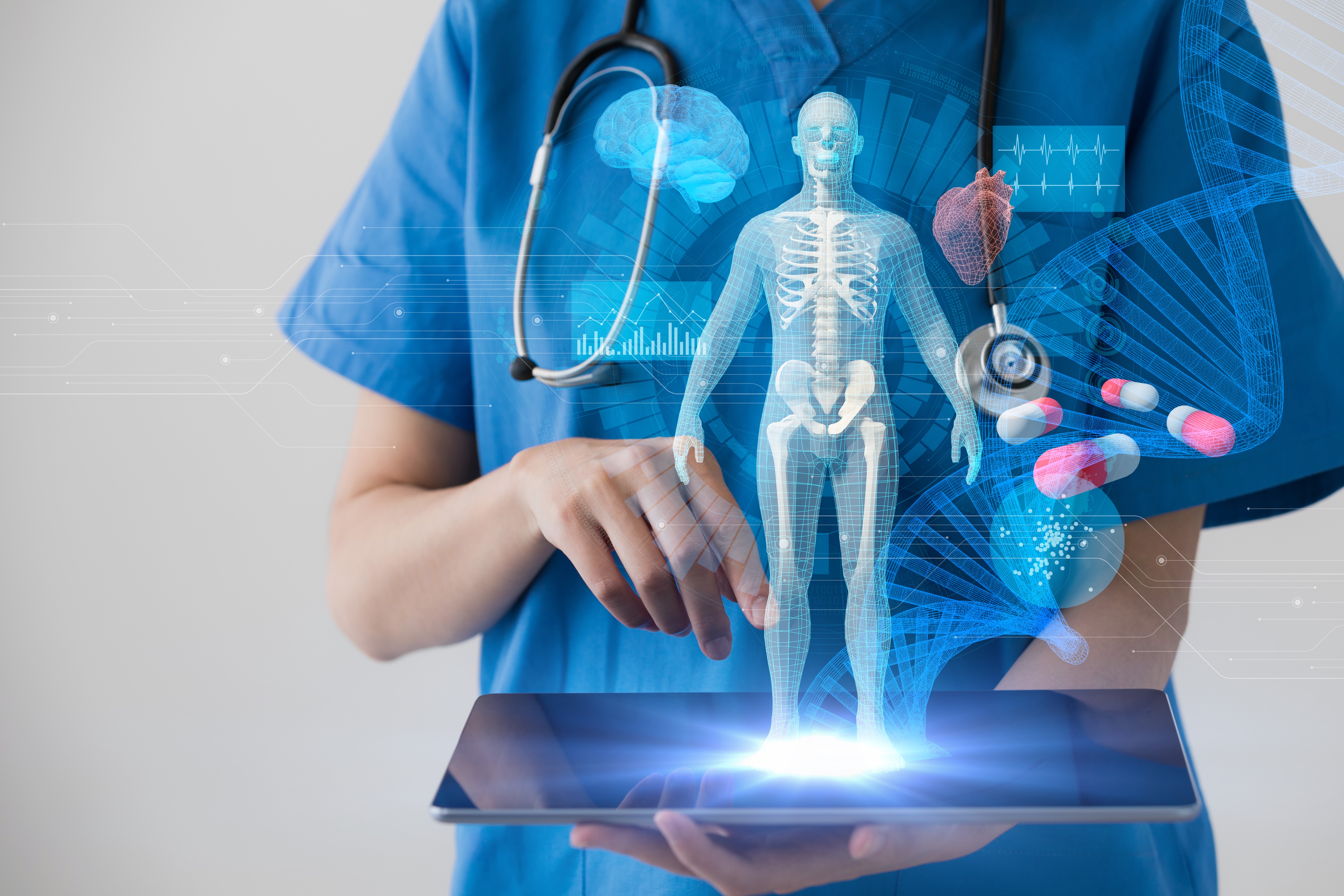
How do AI algorithms discriminate, and what can be done about it?
Those are two questions increasingly on the minds of healthcare stakeholders as AI assumes a steadily more prominent position as a tool in the struggles against the coronavirus and other pressing healthcare challenges.
In a recent commentary, Sharona Hoffman, professor of health law and bioethics at Case Western Reserve University, cited some examples of how algorithms can end up “discriminating” by making assumptions based on data that don’t necessarily support them.
For example, she described a 2019 study that found that an algorithm used to refer chronically ill patients to programs that care for high-risk patients favored whites over sicker African Americans because it “used past medical expenditures as a proxy for medical needs.”
The problem is, she noted, “(p)overty and difficulty accessing health care often prevent African Americans from spending as much money on health care as others. The algorithm misinterpreted their low spending as indicating they were healthy and deprived them of critically needed support.
Similarly, she observed, “doctors often diagnose angina and heart attacks based on symptoms that men experience more commonly than women. Women are consequently underdiagnosed for heart disease.”
So what to do about these and myriad other instances where de-facto discrimination occurs as a result of data that have been skewed repeatedly one way or another.
For Hoffman, the keys to addressing algorithmic bias are litigation, regulation, legislation and best practices.
For litigation, Hoffman is referring specifically to “disparate impact litigation,” which she notes is available to plaintiffs in the fields of employment and housing but not healthcare. “In the AI era,” she says, “this approach makes little sense.”
Under regulation, she calls on the FDA to become more mindful of potential bias problems before it approves new AI systems, while for legislation she promotes the move to make companies study their algorithms more thoroughly in order to root it out before releasing a new algorithm.
Perhaps the most sweeping but also most effective solution Hoffman suggests is for developers to focus on making fairer AI. “Medical AI developers and users can prioritize algorithmic fairness,” she says. “It should be a key element in designing, validating and implementing medical AI systems, and healthcare providers should keep it in mind when choosing and using these systems.”
The bottom line, says Hoffman, is that AI is only going to become more prominent in healthcare, so given the potential damage bias can do a growing number of patients, the time to address and eliminate the problem is now.