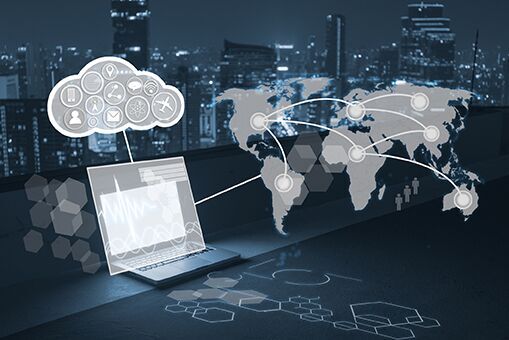
If necessity is the mother of invention, and there’s no doubt the past year’s pandemic has created a great deal of necessity in healthcare systems around the world, then it’s not at all surprising that stakeholders have turned at warp speed to an array of innovative technology solutions.
As John Langton, PhD Director of Applied Data Science, Wolters Kluwer, Health, recently explained in a commentary at HITConsultant, “healthcare organizations were quick to launch telehealth solutions and advance digital health to maintain critical patient relationships and ensure continuity of care. Behind the scenes, hospitals and health systems have been equally adept at advancing technology solutions to support and enhance clinical care delivery.”
In particular, says Langton, healthcare organizations have turned to AI-powered clinical surveillance systems that use real-time and historical patient data to identify emerging clinical patterns, allowing clinicians to intervene in a timely, effective manner. Among other advantages, those systems have enabled many providers to quickly incorporate COVID-19 updates into their clinical surveillance activities, thus providing them with a centralized, global view of COVID-19 cases.
In addition, Langton explains, “by refining the use of AI for clinical surveillance, we can proactively identify an expanding range of acute and chronic health conditions with greater speed and accuracy. This has tremendous implications in the clinical setting beyond the current pandemic. AI-powered clinical surveillance can save lives and reduce costs for conditions that have previously proven resistant to prevention.”
In particular, AI can help help healthcare systems grapple with the healthcare-associated infections (HAIs) that cost the US healthcare system up to $45 billion a year. According to Langton, “AI can analyze millions of data points to predict patients at-risk for HAIs, enabling clinicians to respond more quickly to treat patients before their infection progresses, as well as prevent spread among hospitalized patients.”
One problem he points to, however, is the continued lack of trust “among clinicians and patients around the efficacy of AI. Many clinicians remain concerned over the validity of the data, as well as uncertainty over the impact of the use of AI on their workflow. Patients, in turn, express concerns over AI’s ability to address their unique needs, while also maintaining patient privacy.”
The key, he says, is for hospitals and health systems to be proactive in building that critical trust.
First, Langton argues, systems need to ensure their AI-enabled tools are transparent when it comes to explaining clinical recommendations. Clinicians need “a visual picture of how and why the AI-enabled tool reached its prediction, as well as evidence that the AI solution is effective. AI surveillance solutions are intended to support clinical decision making, not serve as a replacement.”
Next is the need for expanded data access. “The ability to optimize emerging tools depends on comprehensive data access throughout the healthcare ecosystem,” Langton says.
Finally, clinicians and data scientists must collaborate in developing AI tools. Langton notes, “In isolation, data scientists don’t have the context for interpreting variables they should be considering or excluding in a solution. Conversely, doctors working alone may bias AI by telling it what patterns to look for. The whole point of AI is how great it is at finding patterns we may not even consider.”
As Langton sees the future, “AI-enabled clinical surveillance has the potential to deliver next-generation decision-support tools that combine the powerful technology, the prevention focus of public health, and the diagnosis and treatment expertise of clinicians.”
The first critical step, however, is to make sure that people are ready to trust it.