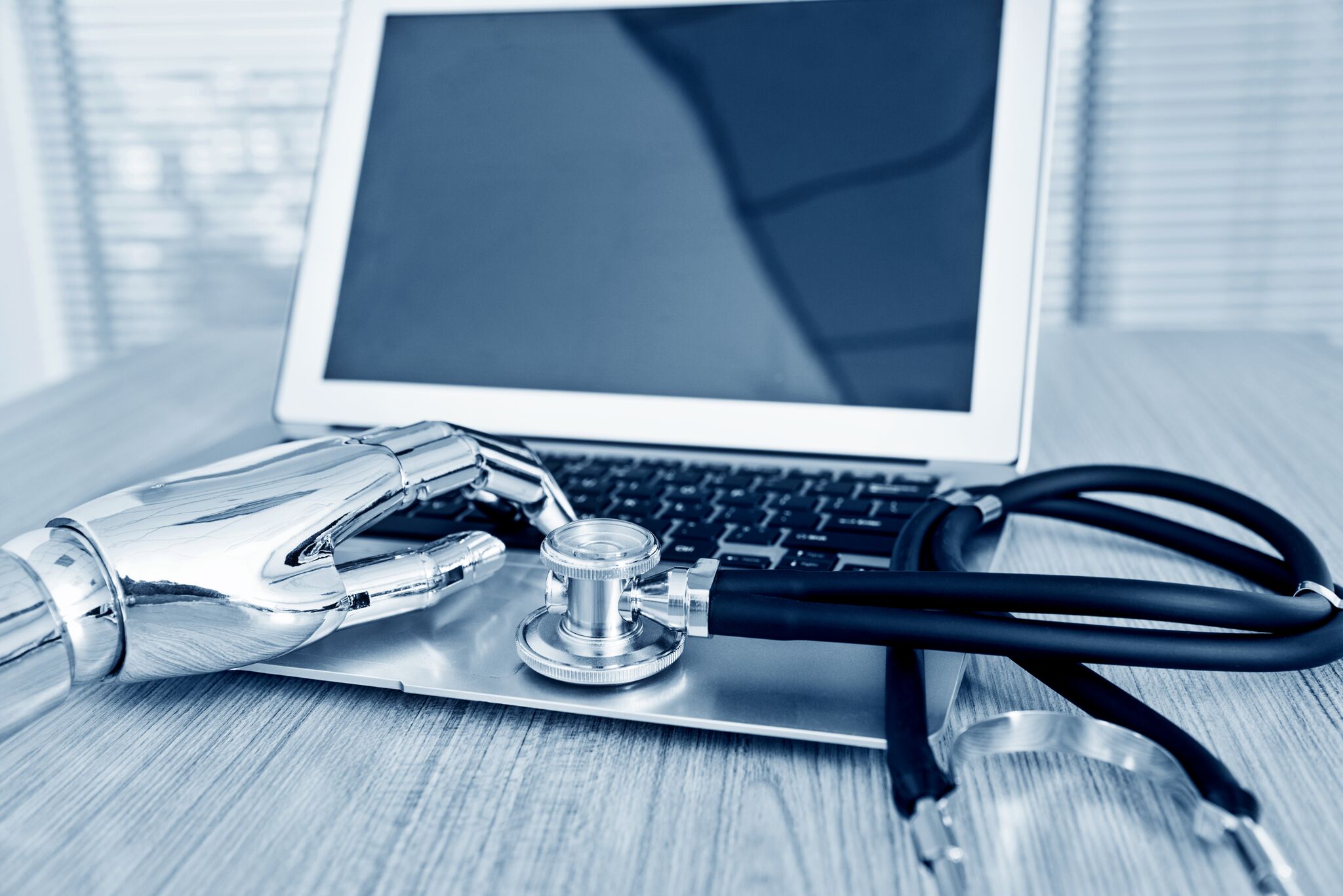
"Despite the global challenges with the COVID-19 public health emergency ... the patient's voice won't be stopped. And if anything, there is even more reason for it to be heard."
So said Dr. Jeff Shuren, Director of the U.S. Food and Drug Administration’s Center for Devices and Radiological Health (CDRH), recently, at a meeting of the FDA’s Patient Engagement Advisory Committee (PEAC) to discuss AI and machine learning in medical devices.
Noting that over 500 medical devices have already been granted emergency use authorization by the FDA in response to the COVID-19 crisis, Shuren said that as more AI and ML-enabled devices are introduced it remains critical for patient needs to be considered as part of the creation process.
"We continue to encourage all members of the healthcare ecosystem to strive to understand patients' perspective and proactively incorporate them into medical device development, modification and evaluation," said Shuren. "Patients are truly the inspiration for all the work we do.”
With that said, Pat Baird, regulatory head of global software standards at Philips, reminded attendees that facilitating patient trust also means acknowledging the importance of robust and accurate data sets.
"To help support our patients, we need to become more familiar with them, their medical conditions, their environment, and their needs and wants to be able to better understand the potentially confounding factors that drive some of the trends in the collected data," said Baird.
"An algorithm trained on one subset of the population might not be relevant for a different subset.”
As an example, Baird said if a hospital needed a device that would serve its population of seniors at a Florida retirement community, an algorithm trained on recognizing healthcare needs of teens in Maine would not be effective.
"This bias in the data is not intentional, but can be hard to identify," he explained, adding, “We need to use our collective intelligence to help produce better artificial intelligence populations.”
He encouraged the development of a taxonomy of bias types that would be made publicly available, noting that, ultimately, people won't use what they don't trust.
The committee also examined how informed consent might play a role in algorithmic training.
"If I give my consent to be treated by an AI/ML device, I have the right to know whether there were patients like me ... in the data set," said Bennet Dunlap, a health communications consultant.
The committee called on the FDA to take a strong role in addressing algorithmic bias in artificial intelligence and machine learning.
"At the end of the day, diversity validation and unconscious bias … all these things can be addressed if there's strong leadership from the start," said Dr. Paul Conway, PEAC chair and chair of policy and global affairs of the American Association of Kidney Patients.