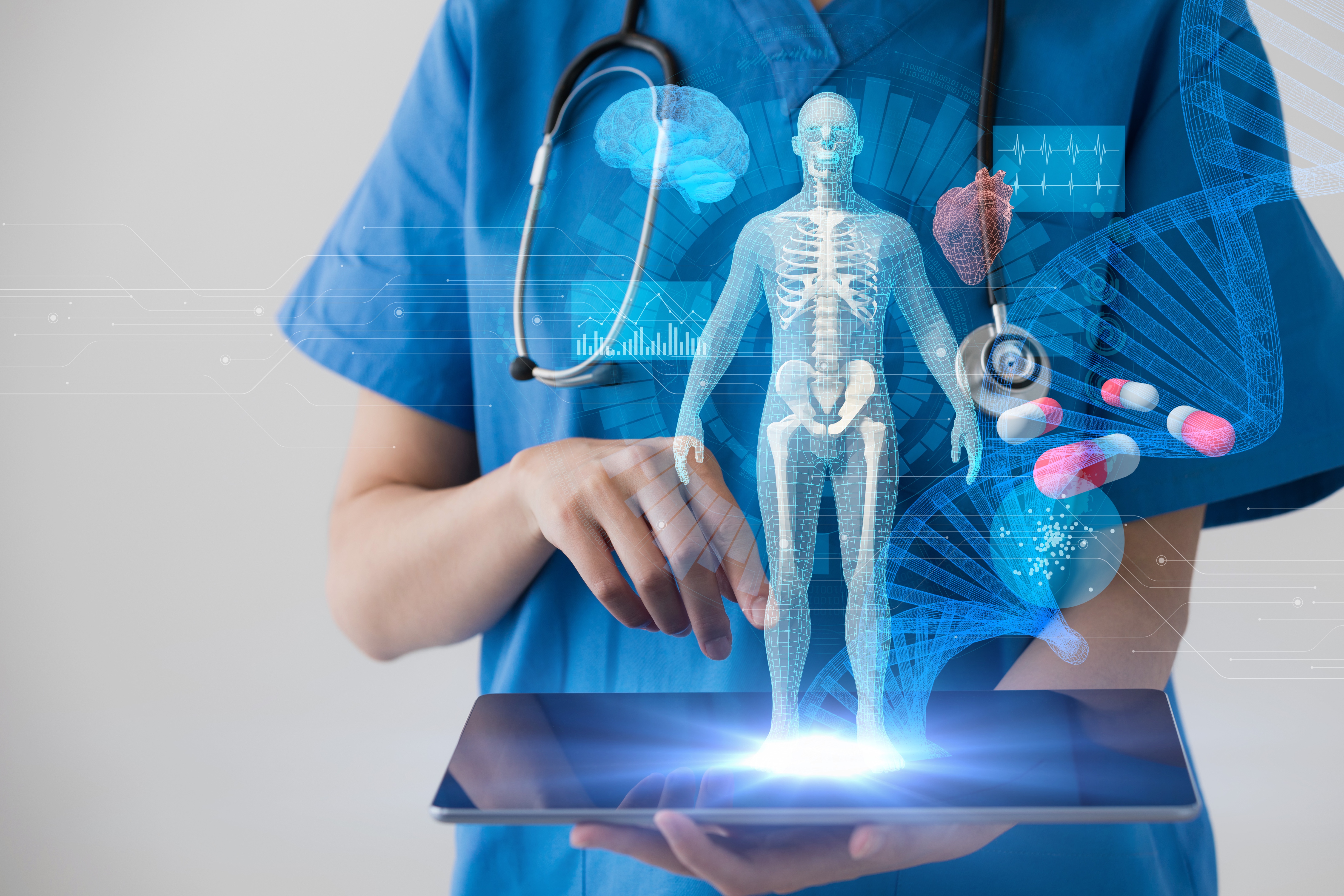
AI has the potential to revolutionize medicine, but AI needs copious amounts of good data on which to be trained, and those resources are hard to come by due to privacy regulations and the absence of standardized EHRs.
That’s according to a recent article at Nature written by a team of academics from the University of Munich and the Imperial College London.
To be sure, the writers note, AI has already had an impact, particularly in the domain of medical imaging. But “(t)o allow medical imaging AI applications to offer clinical decision support suitable for precision medicine implementations, even larger amounts of imaging and clinical data will be required.”
Currently, they say, the streams of data available for purposes of training machine learning algorithms are random and largely voluntarily offered. “(S)uch datasets often stem from relatively few institutions, geographic regions or patient demographics, and might therefore contain unquantifiable bias due to their incompleteness with respect to co-variables such as co-morbidities, ethnicity, gender and so on. . . . (C)onsidering that the sum of the world’s patient databases probably contains enough data to answer many significant questions, it becomes clear that the inability to access and leverage this data poses a significant barrier to AI applications in this field.
“The lack of standardized, electronic patient records is one reason. Electronic patient data management is expensive, and hospitals in underprivileged regions might be unable to afford participation in studies requiring it, potentially perpetuating the aforementioned issues of bias and fairness.”
Following an in-depth analysis of both the problem and the potential technological solutions, the writers list a number of areas for “multi-disciplinary research and investment” that should facilitate the widespread adoption and utilization of AI.
First and foremost, they argue that “(d)ecentralized data storage and federated learning systems, replacing the current paradigm of data sharing and centralized storage, have the greatest potential to enable privacy-preserving cross-institutional research in a breadth of biomedical disciplines in the near future, with results in medical imaging and genomics recently demonstrated.”
Other suggestions include more research into “the trade-offs between accuracy, interpretability, fairness, bias and privacy (privacy-utility trade-offs)” in order to develop algorithms that are at once more accurate and more secure, as well as “the development of auditable and objectively trustworthy systems (that) will promote the universal acceptance of secure and private AI solutions by individuals and policymakers. need to be researched.”
Finally, they say, “we view both the education of patients, physicians, researchers and policymakers, and the open scientific, public and political discourse about privacy, current risks and technical possibilities as paramount for reinforcing the cultural value of privacy and cultivating a sustainable attitude of trust and value-aligned cooperation both in science and society.”