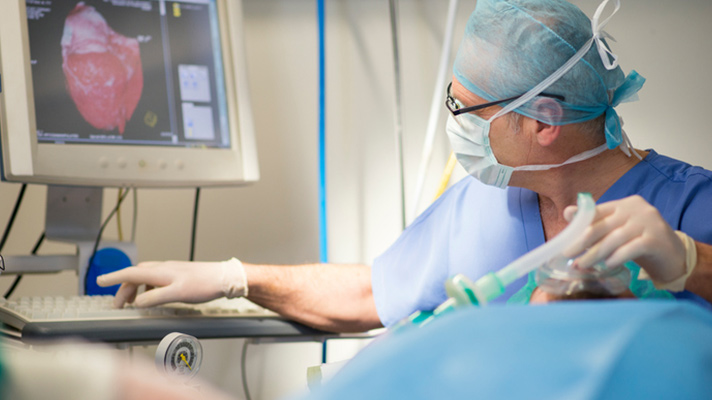
Discussions about artificial intelligence, or AI in radiology, tend to focus on improving patient outcomes through accurate detection and quicker clinical workflows. By integrating and assimilating global multivariate data from disparate sources, AI technology promises to generate insights to support clinical decision-making.
But sometimes what’s lost in this perspective is AI’s positive, and much needed, impact on radiologists themselves.
Radiologists today face increased pressure because of steadily growing volumes and increasingly complex image reviews. “A staggering 90% of all healthcare data comes from imaging technology, yet 97% of it goes unanalyzed or unused,” according to GE Healthcare.[1
The impending shortage of radiologists and ongoing prevalence of burnout will further strain healthcare over the next few years and onward. A survey of 15,000 respondents in the Medscape National Physician Burnout, Depression & Suicide Report 2019 found that an incredible 45% of U.S. radiologists have reported burnout symptoms during the last year. Radiology came in 12th place out of 29 among all specialties.[2]
“When I talked to a lot of imaging leads, they knew burnout was an issue,” said Peter Eggleston, global product marketing director at GE Healthcare. “But they didn’t have any programs in place to specifically do anything about it.”
Paradoxically, computerization itself seems to have exacerbated this workload stress problem.
In the past 20 years, computerization of the radiology practice has led to increased workloads because it has vastly increased the number of scans to evaluate (3D tomography increases the number of scans the clinician must evaluate), and learning new software can be frustrating and time-consuming.
For Eggleston, the situation prompts a caution about deploying new AI tooling. “Implemented incorrectly, this very technology can cause an increase in cognitive workload, thus further contributing to burnout,” he said. If an algorithm that can find a blood clot or a broken vertebra has its own interface, its own way of reporting, “it’s going to cause more cognitive overload, making the burnout problem worse.”
Put another way, integration with existing systems (EHR, PACS) is just as important as the AI algorithm and user interface, or UI. These technologies need to be part of the existing radiology workflow, seamlessly integrated with them, and not part of new applications, UIs, or workflows. “Technology should work with us, not against us,” Eggleston added.
‘Clinical assistant’
Eggleston emphasizes that AI systems integrated in this fashion will assist, rather than replace, radiologists.
This maps to much of the current thinking on the subject. Even a deep learning model, based on 1 million mammographic images that performs on par with expert radiologists in identifying breast cancer using screening mammograms, is shown to be a better diagnostic tool when used in conjunction with a radiologist, not as a substitute for one.[3]
How would this look in practice? What if the AI scored what it found to be a probable site of a brain bleed and used this to order the images to arrange the work list so that the anomaly the algorithm found will be at the top, to be looked at first? Or what if the AI’s assessment could be shown when the doctor reached the right part of the image?
Similarly, a system that prioritizes scans of an accident patient with multiple injuries would be beneficial and perhaps life-saving. AI could also take measurements during the scan and place these into the exam notes, saving the radiologist a step. “If we put information where the clinician is expecting it, then you’ll have one seamless UI that’s consistent across all the AI solutions,” Eggleston said.
This promises to reduce cognitive overload and, more importantly, give radiologists more time to think about what is going on with patients, diagnose more complex cases, and collaborate with patient care teams.
Properly conceptualized and weaved into the workflow, AI systems will offer “a more complete context and picture of the patient, the reason for the exam, and will assist in quickly separating relevant from irrelevant information,” Eggleston proposed.
Less time spent on routine manual work will permit radiologists to focus on what’s important, benefiting themselves and, of course, their patients
[1] “One of the largest AI platforms in healthcare is one you've never heard of, until now.” GE Healthcare. Nov. 24, 2018. http://newsroom.gehealthcare.com/new-apps-smart-devices-launch-healthcar....
[2] Kane L. Medscape National Physician Burnout, Depression & Suicide Report 2019. January 16, 2019.
[3] Jason Phang. “Deep Neural Networks for Breast Cancer Classification.” Medium.com. https://medium.com/@zp489/f06e7daaee5.