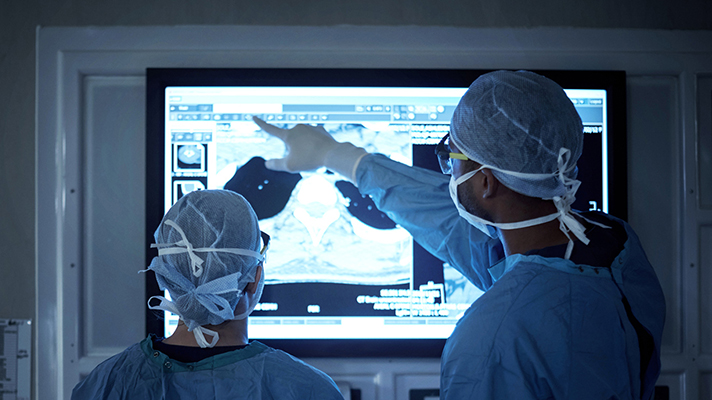
Notwithstanding exciting data science advances fueling artificial intelligence theory, in the real world of hospitals and healthcare systems, AI’s biggest benefits are distinctly practical. They center around improving clinical workflow, productivity, and efficiency.
Tools that help augment, quantify, and stratify voluminous and growing amounts of imaging data are desperately needed. Radiologists today face increased pressure because of steadily growing volumes and increasingly complex image reviews.
A staggering 90% of all healthcare data comes from imaging technology, yet 97% of it goes unanalyzed or unused.[1] Accessing more of this data, along with improving how it is used within a clinical workflow, will give radiologists more time to interpret findings, accurately assess complex cases, and collaborate with care teams. This will help improve individual patient experiences and outcomes, as well as create a platform that supports precision health and population health.
According to Peter Eggleston, global product marketing director at GE Healthcare, intelligent workflows using AI will help radiology along three dimensions:
1. Productivity: Automating and prioritizing tasks and data feeds, making the physician and department more productive.
2. Quantitatively: Providing applications and tools to semi-automatically or automatically extract and quantify information.
3. Precision: Ensuring the right information is available, filtered, and presented to support the diagnosis, as well as guaranteeing the repeatability of any quantification processes.
One immediate opportunity is to use the AI to filter scans “to tell if someone has a life-threatening condition,” Eggleston said. An example is Critical Care Suite on OptimaTM XR240amx from GE Healthcare. The mobile X-ray system is designed to immediately identify and prioritize critical conditions, such as pneumothorax, or collapsed lung, at the point of care. Or, in the case of GE Healthcare’s CentricityTM Universal Viewer Smart Reading Protocols, AI is leveraged to learn the radiologists’ hanging preferences, saving time in setting up future reads.
In another example, AI can be effective for stroke imaging by providing automatic image reformatting in the picture archiving and communication system, or PACS, to save reprocessing time — performing some types of post-processing automatically, for example. Similarly, for brain imaging, AI can provide automatic volumetric visualization and show anatomically relevant views without operator assistance. AI can automatically select organs of interest, viewing parameters and orientation to provide optimal repeatable views, which would save clinicians valuable time.
But beyond emergency medicine, an AI-backed workflow should help clinicians coordinate better with other departments. In addition, such tools will give health systems without radiology specialists (think hospitals in rural areas or developing countries) a way of providing, at least, a first pass and flagging those images it determines should be further inspected.
Precision
There is at least one nonobvious benefit of automation tools: They provide assistance by sorting and filtering images, adding measurements to notations, or prioritizing worklists. Automation tools will help healthcare providers better document the care path and comply with care protocols.
Indeed, while most hospitals have protocols for dealing with deteriorating patients, AI assistance may offer a better way of presenting these policies at the point of care. A National Institutes of Health study found that compliance with an institution’s protocols can be as low as 12% for Level 1 trauma. In general, compliance with protocols might be only around 50%.[2]
Tracked and analyzed in aggregate, existing protocols could be improved or new ones developed. All this should help remove unwanted variation in the care path, which has been shown repeatedly to help improve healthcare outcomes.
Finally, hospital CIOs may find that such systems reduce how much time their staff spends in giving court depositions in trials. “They report that if the AI can just help imaging organizations to find more of the incidentals that might have otherwise been missed, then it would be worth it,” Eggleston said.
Efficiency mandate
Unquestionably, these CIOs are looking for ways to improve the operational efficiency of their healthcare systems.
According to industry leaders interviewed in a 2018 article published in Diagnostic Imaging,[3] approximately $15 billion to $20 billion of radiology’s expenditures are unnecessary and avoidable, caused by shortages in the workflow. Either the right test is conducted at the wrong time, or patients receive an unnecessary repeat exam.
According to an April 2019 survey conducted by HIMSS Media on behalf of GE Healthcare titled Perspectives on Precision Health, a majority of the 100 respondents said they saw an immediate opportunity to bring AI/ML into the clinical workflow, getting information to the right people at the right time, and into the management of chronic health conditions. Further, 4 in 10 said they are making AI and ML a focus of greater strategic activity in the next 12 months.
To understand how AI is currently being used and will be successfully leveraged in the future in imaging and clinical apps, GE Healthcare enlisted MarketVision Research,[4] which conducted a double-blind research study, gathering information from more than 60 imaging leads, including radiology chiefs, radiology directors, managers, and imaging directors from the U.S. and U.K.
The results from that research showed that use of AI in imaging workflows is expected to grow significantly over the next few years. While only 12% of the respondents are using AI today, in two years, 58% expect to have started using AI in imaging workflows. And in five years, 90% expect to have started using AI
[1] “One of the largest AI platforms in healthcare is one you've never heard of, until now.” GE Healthcare. Nov. 24, 2018. http://newsroom.gehealthcare.com/new-apps-smart-devices-launch-healthcar....
[2] Shafi S, Barnes SA, Rayan N, Kudyakov R, Foreman M, Cryer HG, Alam HB, Hoff W, Holcomb J. “Compliance with recommended care at trauma centers: association with patient outcomes.” J Am Coll Surg. 2014 Aug;219(2):189-98. doi: 10.1016/j.jamcollsurg.2014.04.005. Epub 2014 Apr 30. https://www.ncbi.nlm.nih.gov/pubmed/25038959.
[3] Whitney J. Palmer. “How to streamline radiology workflow.” Diagnostic Imaging. MJH Life Sciences. Aug. 27, 2018. https://www.diagnosticimaging.com/article/how-streamline-radiology-workflow.
[4] “Artificial Intelligence in Healthcare: 10 Questions: AI and machine learning technology are the rising stars of healthcare, empowering providers to deliver faster, more precise care.” Gehealthcare.com. Page 3. 2019. https://docplayer.net/156214226-Artificial-intelligence-in-healthcare-10....