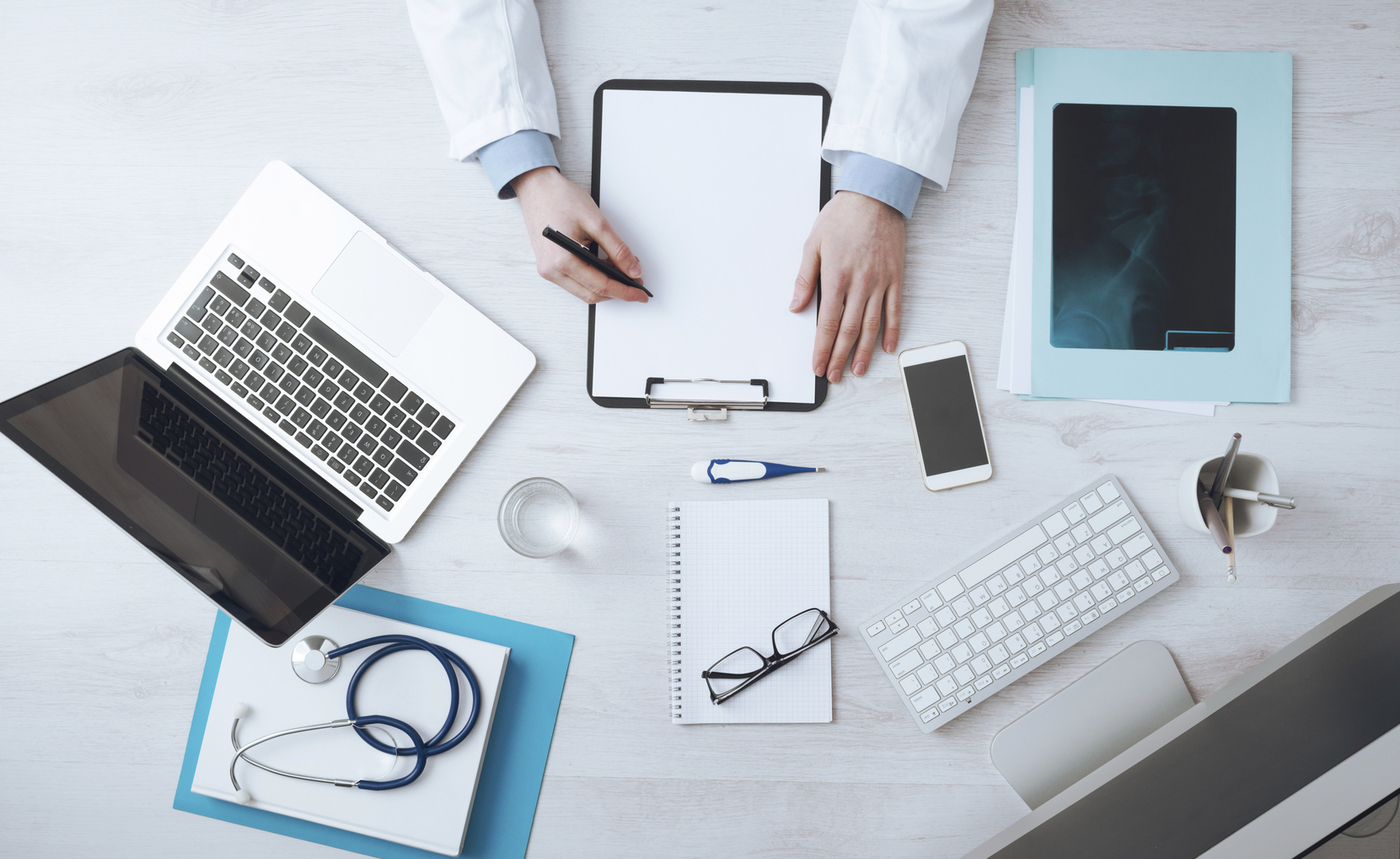
It’s no secret that there’s a hype cycle to most developments in health IT, most likely because due to the increasingly “futuristic” capabilities that new technologies are offering.t
AI and Machine Learning (ML) are far from an exception on this count, but as our colleague Dean Koh recently observed at HealthcareIT News, given the fact that that both AI and ML are data-hungry technologies, “the reality is that the current sources of data are fragmented and unstructured, making it difficult to truly harness the power of data” as a boundless source of insights for AI to explore.
Koh’s observation comes at the beginning of an interview with Dr. Ian Chuang, CMO of Elsevier, and what seems most valuable about Dr. Chuang’s perspective is that he’s more sanguine than enthusiastic about AI/ML, recognizing both the opportunities being presented and the challenges remaining to realize those opportunities.
For example, on that point about the exploding amount of data available, Dr. Chuang points out that “(m)uch of the data that is being gathered today through routine care comes from multiple sources, such as electronic health records and health tracking devices. This data is often inconsistent and unstructured for computing, but is broadly available and reflects realistic care settings and co-morbidities. To turn this into credible, real-world evidence that supports clinical practice, the data first need to be structured, coded and for AI to even begin.”
Of course, says Dr. Chuang, even as the data challenges are being sorted through and increasingly cleaned up, AI developers will need to move with caution when it comes to putting data to work developing new algorithms.
“We will require ways for peer review of the dataset and algorithms associated with machine generated knowledge,” he notes, adding, “This aspect of AI and ML will advance slowly and require judicious analysis and cross validation with existing knowledge that has been time tested.”
One of the more interesting perspectives Dr. Chuang offers concerns the potential of AI to further “democratize” healthcare by putting patients increasingly in charge of their own care.
“We need patients to be engaged in their own health and health maintenance,” he argues. . . . If healthcare is truly a calling and the calling is about serving and providing care for those who seek our care, then clinicians should evolve and align the care experience and the relationship with the patients that help them feel valued as a person: regarding their opinions and fears as important, and their preferences and choices as important considerations to the care decisions.”
In the end, while recognizing the potential utility of AI to deliver better health insights and outcomes for patients, Dr. Chuang points to the need for everyone to be more flexible when it comes to their expectations.
“One important criterion for success will require all stakeholders to start by changing mindsets and attitudes and not be adversarial,” he says. “Payers are not trying to rip off the clinicians and patients, clinicians are not trying to milk the system, and patients are not trying to get services for free. Collectively, we can take strides to define a health care model for the future, leveraging knowledge and technology.”