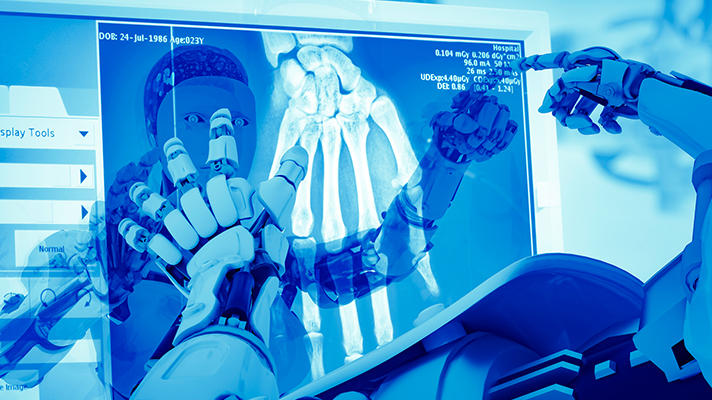
How will clinical workflows change once artificial intelligence and machine learning systems become commonplace?
Clinical workflows have already changed as part of the digital transformation of healthcare, said Joyce Sensmeier, vice president of informatics, technology and innovation at HIMSS. “The challenge we have is making sure that there are expert informaticists and others helping to inform and guide these workflows, and figure out the best technologies to leverage in different situations,” she said.
Current EMRs, of course, implement some level of workflow logic today, such as prompting a nurse to ask certain questions when starting a new episode of care for a patient. The next step in this automation will be clinical recommendations. “That’s the direction in which we absolutely want to head,” Sensmeier said, noting that clinicians have been spending years inputting data into EMRs. “Now it’s time to gain back value from that.”
Certainly, obstacles to full and effective implementation persist. These range from valid concerns about the trustworthiness and security of AI-enabled clinical systems, to still-developing data interoperability and regulatory frameworks.
But participants at the 2019 World Medical Innovation Forum voiced concerns that the healthcare system is not doing enough to prepare for the fundamental changes AI will bring over the next several decades. “We are at a turning point in terms of how we can use data to support improvements to the healthcare system,” CMS Administrator Seema Verma told HealthITAnalytics.com.[1] “The timing is right for true digital transformation.”
One driver of that transformation, which will include AI-assisted systems, is physician burnout.
“Right now, 47% of radiologists are experiencing burnout in their current role and activities,” said Travis Frosch, the global data strategy leader at GE Healthcare. There is an opportunity, therefore, not to replace radiologists but to make their job substantially easier by handling the hundreds of routine scan evaluations they do every day, such as lesion detection, he said.
Even before AI-enabled systems affect hospital-wide workflows, there’s a significant opportunity to optimize critical care processes, Frosch said. “Instead of going through a whole workflow process, you’ve now put the AI on the eyeball, on the actual device itself.” He said that innovation can save “seconds, minutes, hours, and that can make the difference in critical treatments.”
In that subset of scans in which the AI is unsure, the system would flag a human radiologist to review the scan, ideally highlighting an area of interest. “You want to filter the normals and allow the radiologist to focus on the really difficult cases to read,” Frosch said.
At the last meeting of the Radiological Society of North America, GE Healthcare released a solution called AIRxTM. AIRx on MR is designed to use deep learning algorithms to automatically detect and prescribe slices for routine and challenging neurological exams, delivering consistent and quantifiable results.
Another GE Healthcare solution, SonoCNS, does something similar except in the use case of an ultrasound study of the fetal brain. “With SonoCNS, there are 80 percent fewer keystrokes to take the four key measurements [of the fetal central nervous system],” Frosch said, comparing it to a self-parking car.
For AI to augment clinical decision-making is most definitely positive thing, said Sensmeier. “To me, the word that’s essential is ‘augment.’”
Are EMRs ready?
But are hospitalwide EMRs ready to accept input from AI-enabled devices and applications? In other words, can the additional intelligence at the device or department level be absorbed by today’s enterprise EMRs?
“It’s a good question,” said Frosch. “But I would spin it around in a different direction. The thing our teams are doing in developing these solutions is using standard processes and standard healthcare exchange frameworks to move information.”
A GE Healthcare device taking an X-ray, for example, will still acquire an image in a Digital Imaging and Communications in Medicine (DICOM) format, meaning this data can go through known exchanges and information routing. “So, the thing you were doing three years ago is still the thing that happens today,” he said.
That said, the teams are also thinking about how to use the existing pipeline to move smarter assessment of that image to the right place. “So, some teams are placing heat maps on a secondary DICOM and sending that along as well,” he said. “If the radiologist is reading the case, he or she sees the original chest X-ray, plus a notification of the secondary DICOM with a heat map, showing, say, the suspected pneumothorax identified by the AI.”
Frosch allowed that there are user interface issues still to work out and noted that this process hasn’t been delivered at scale, “so we don’t know the potential impacts across vendors.” Indeed, he said many customers are concerned about managing AI solutions from a panoply of vendors. “[These startups] may not own the workflow framework, so for them it’s a little more challenging to embed that into an existing workflow,” he said.
Piggybacking
Frosch makes the point that these AI-enabled insights will need to piggyback on existing UIs and workflows. “The thing that you don’t want to do is disrupt existing healthcare workflows,” he said, recalling the early, difficult days of EMR deployment.
Familiar UIs may be necessary for buy-in, agreed Sensmeier, but she added that clinicians are open to disruption in their existing workflow provided “it takes them in a new and positive direction, saves them time, and adds efficiency.”
Take, for example, devices that automatically transfer data from patient sensors into the EMR. “Clinicians are open to that if they have confidence in the accuracy of the data,” Sensmeier said. “No one is buying into cumbersome workflows just because that’s the way it’s been.”
[1] Jennifer Bresnick, Verma: Artificial Intelligence is Key for Patient Empowerment, Lower Costs, Health IT Analytics, April 10, 2019, https://healthitanalytics.com/news/verma-artificial-intelligence-is-key-for-patient-empowerment-lower-costs.