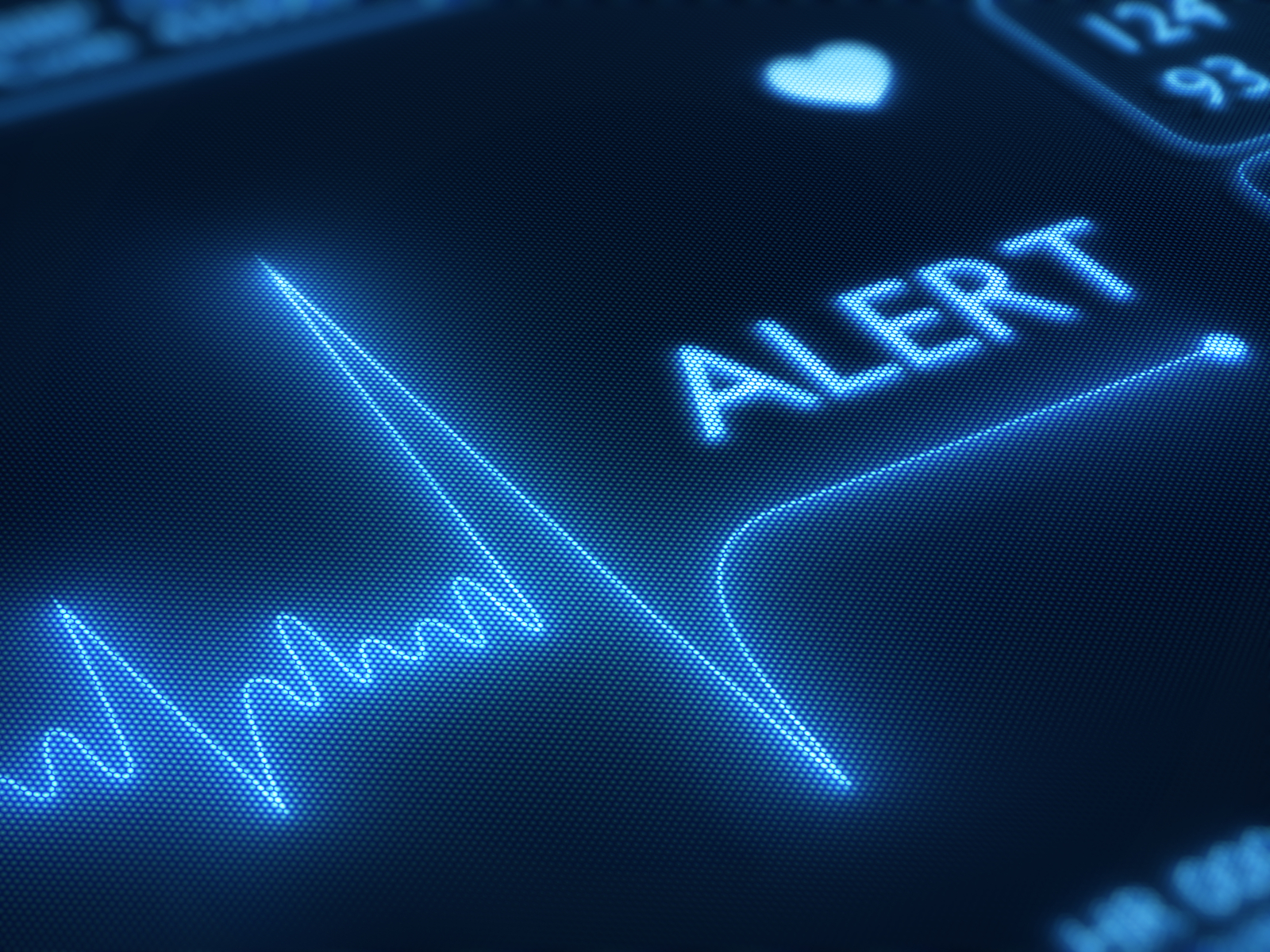
It’s that time of year: flu season. And while the Centers for Disease Control and Prevention monitors influenza-like illnesses (ILI) in the U.S. by gathering information from physicians’ reports about patients with ILI seeking medical attention, the availability of the data reportedly has a lag time of as much as two weeks.
But AI may be able to help shorten that wait, as researchers led by Boston Children’s Hospital recently released a study suggesting that machine learning can produce highly accurate local flu surveillance faster than traditional healthcare-based reports.
In the study published recently in Nature Communications, researchers say they have successfully combined Google search frequencies and electronic health record data with spatio-temporal trends in influenza activity to produce forecasts with higher correlation and lower errors than all other tested models for current ILI activity at the state level.
“We believe that the accuracy of our method involves a balance between responsiveness and robustness,” state the authors. “Real-time data sources such as Google searches and electronic health records provide information about the present, allowing the model to immediately respond to current influenza trends.”
“Timely and reliable methodologies for tracking influenza activity across locations can help public health officials mitigate epidemic outbreaks and may improve communication with the public to raise awareness of potential risks,” says senior author Mauricio Santillana, a faculty member of the Computational Health Informatics Program at Boston Children's Hospital.
The approach, called ARGONet, “exploits the fact that the presence of flu in nearby locations may increase the risk of experiencing a disease outbreak at a given location,” adds Santillana. “The system continuously evaluates the predictive power of each independent method and recalibrates how this information should be used to produce improved flu estimates.”
“We think our models will become more accurate over time as more online search volumes are collected and as more healthcare providers incorporate cloud-based electronic health records,” says first author Fred Lu, an investigator for the Computational Health Informatics Program at Boston Children's Hospital.
In particular, researchers cited the value of electronic health records from athenahealth, which they noted is a cloud-based EHR vendor that is currently used by more than 100,000 providers across all 50 states.
“We anticipate that data sources will improve over time, for example, if athenahealth continues to gain a larger market share over the states or more Google Trends information becomes available,” conclude the authors. “If these conditions hold, or as more web-based data sources or other electronic health record systems become available in real time, the accuracy of our methods may continue to increase.”