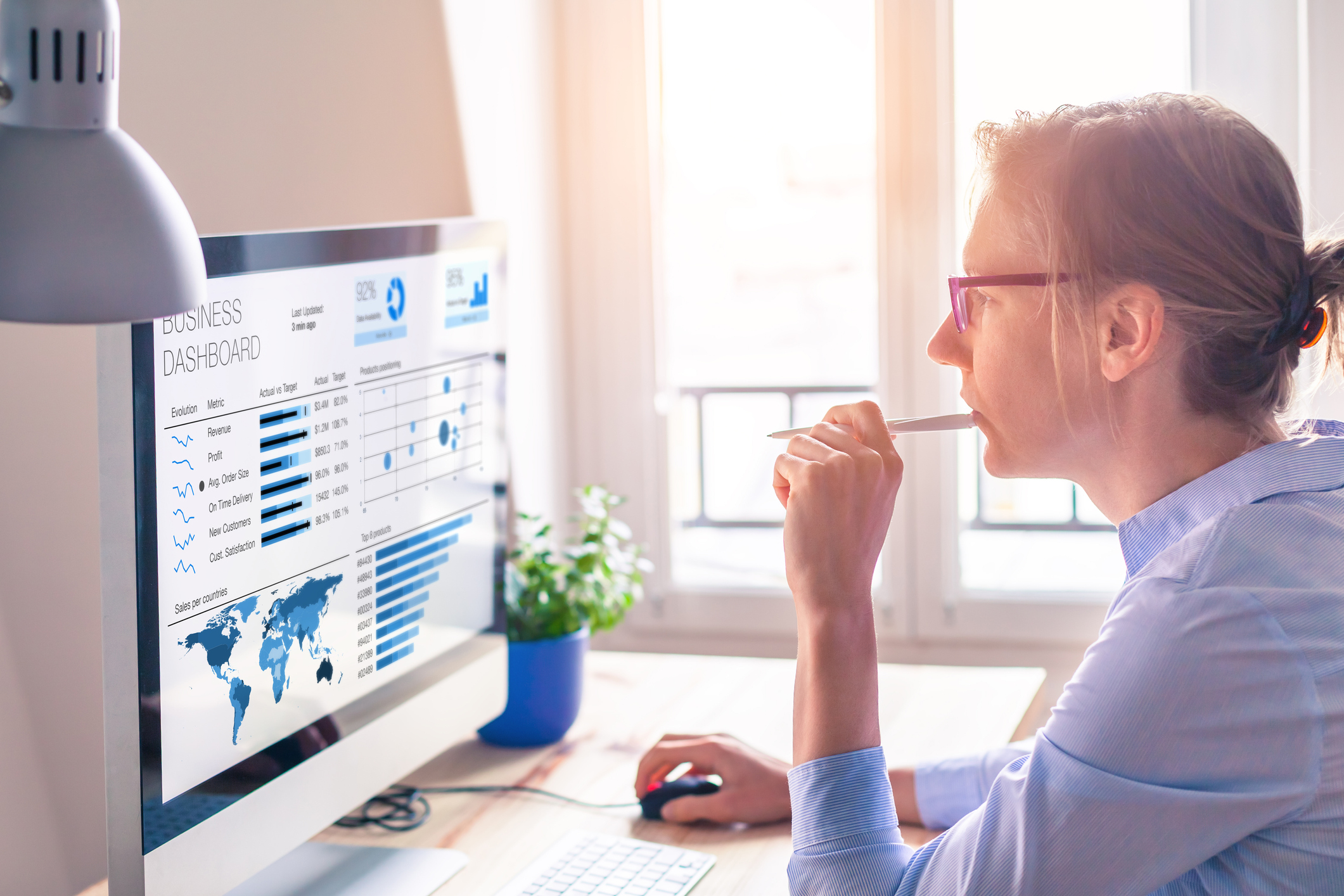
In healthcare, digital dashboards are a key tool providers can use to get a snapshot look at a range of patient data. So it isn’t surprising that providers and other healthcare stakeholders are beginning to consider how AI-enhancement might make dashboards even better.
As Dr. Jean Drouin, CEO of Clarify Health Solutions, an analytics startup, recently put it to MobihealthNews, “Today’s dashboards are a bit static. Yes, they get a daily feed, maybe from the [EHR], or yes, they provide trends. But generally, you are left with the information that’s on the dashboard — it is difficult to click through.”
Enter Artificial Intelligence, although it’s likely that many providers may not know how best to incorporate AI into their dashboards.
According to Drouin, an organization looking to integrate AI into its monitoring dashboard, needs to make sure that any implementation will actively work toward increasing the dashboard user’s awareness and understanding of operations.
“A good dashboard is something that individuals who need to monitor or to act on something can go to to rapidly be able to answer a couple of key questions,” he explained. “I think of it really in terms of [whether] the ML (Machine Learning) and AI help to provide information more quickly. Does it help to provide more precise or trusted information, or does it help to provide more actionable information? It turns out, depending on the metric, ML and AI can help with all three, and I think it’s helpful to first think [where it] it can really be helpful, or what concept can be smoke and mirrors.”
Similarly, Mudit Garg, CEO and founder of management tech platform maker Qventus, sees AI as an opportunity to offload a “tremendous amount of cognitive load” by intelligently automating data interpretation. With that goal in mind, an organization deciding where best to point the technology might want to start by targeting analysis projects a human employee could perform, if given an open schedule.
“If you, as an end user, had completely free time to be looking at this all the time, what are the decisions you would make?” Garg asked. “You don’t want to focus on things you can’t do anything about. It’s a lot more about what is the decision you are trying to make, who makes it, what is the cost and benefit of that decision, and then with all that information you think about what is the best path of operation?”
Drouin recommendation was to look to other industries, for example, how banking has already employed ML and AI to stratify loan risks. In his view, a similar approach could be taken to gauge patient’s risk of readmission or face other complications or to identify and draw insights from the performance of an individual physician.
“That kind of information about the relative performance of a physician can then be delivered into a physician’s monthly dashboard about their performance, or on an administrator’s dashboard [for] the items I need to discuss with my physician when I have my quarterly discussion about his or her performance,” he explained. “Where AI can be useful in this context is rather than have an analyst have to spin through lots and lots of analyses to find which physicians are more variated in their performance, or [among] what types of patients that physician has variance in performance, you can get a computer to very, very quickly go in and get all the outliers.”
As Garg summed it up, “The end goal is making sure the right things are happening reliably and consistently. As a person responsible to make decisions in my department, [rather than] showing me everything that’s going on and relying on my cognitive ability to spot what I think and to figure out what to do with that … draw my attention to the thing I should be paying attention to . . . and make it really straightforward for me to do that.”