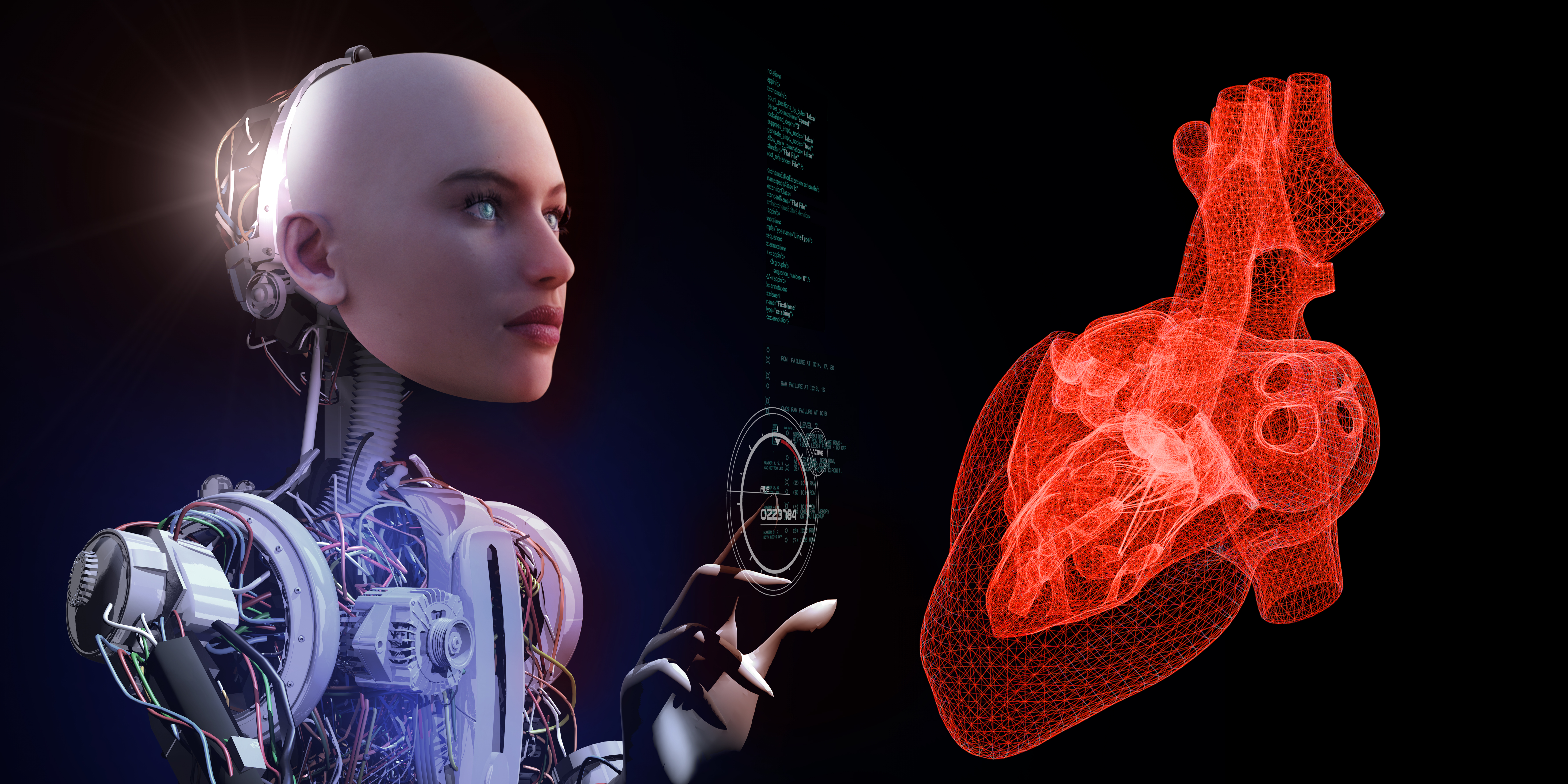
Researchers at the Mount Sinai Clinical Intelligence Center have developed an AI algorithm that can analyze electrocardiograms (ECGs) and identify the subtle changes in heart rhythm that may indicate patient heart failure.
“Heart failure represents a significant health burden, with an estimated 6.2 million people affected in the United States and at least 64 million people worldwide,” the team wrote in a report published in the Journal of the American College of Cardiology: Cardiovascular Imaging.
For years doctors have relied heavily on ECGs to assess whether a patient may be experiencing heart failure, but they can be labor-intensive procedures that are only offered at select hospitals. The goal of the study was to determine whether recent developments in AI may make the procedure more readily available and more insightful.
“We showed that deep-learning algorithms can recognize blood pumping problems on both sides of the heart from ECG waveform data,” said Benjamin S. Glicksberg, PhD, Assistant Professor of Genetics and Genomic Sciences, a member of the Hasso Plattner Institute for Digital Health at Mount Sinai, in a release. “Ordinarily, diagnosing these type of heart conditions requires expensive and time-consuming procedures. We hope that this algorithm will enable quicker diagnosis of heart failure.”
According to the researchers, many studies have shown how a “deep-learning” algorithm can detect weakness in the heart’s left ventricle, which pushes freshly oxygenated blood out to the rest of the body. In this study, the researchers described the development of an algorithm that not only assessed the strength of the left ventricle but also the right ventricle, which takes deoxygenated blood streaming in from the body and pumps it to the lungs.
“Although appealing, traditionally it has been challenging for physicians to use ECGs to diagnose heart failure. This is partly because there is no established diagnostic criteria for these assessments and because some changes in ECG readouts are simply too subtle for the human eye to detect,” said Dr. Nadkarni, Associate Professor of Medicine at the Icahn School of Medicine at Mount Sinai and a senior author of the study. “This study represents an exciting step forward in finding information hidden within the ECG data which can lead to better screening and treatment paradigms using a relatively simple and widely available test.”
For the study, the researchers programmed a computer to read patient ECGs along with data extracted from written reports summarizing the results of corresponding echocardiograms taken from the same patients. The written reports acted as a standard set of data for the computer to compare with the ECG data and learn how to spot weaker hearts. Special neural networks capable of discovering patterns in images were incorporated to help the algorithm learn to recognize pumping strengths.
The computer then read more than 700,000 electrocardiograms and echocardiogram reports obtained from 150,000 Mount Sinai Health System patients from 2003 to 2020. Data from four hospitals was used to train the computer, while data from a fifth one was used to test how the algorithm would perform in a different experimental setting.
“A potential advantage of this study is that it involved one of the largest collections of ECGs from one of the most diverse patient populations in the world,” said Dr. Nadkarni.
Photo by Devrimb/Getty Images