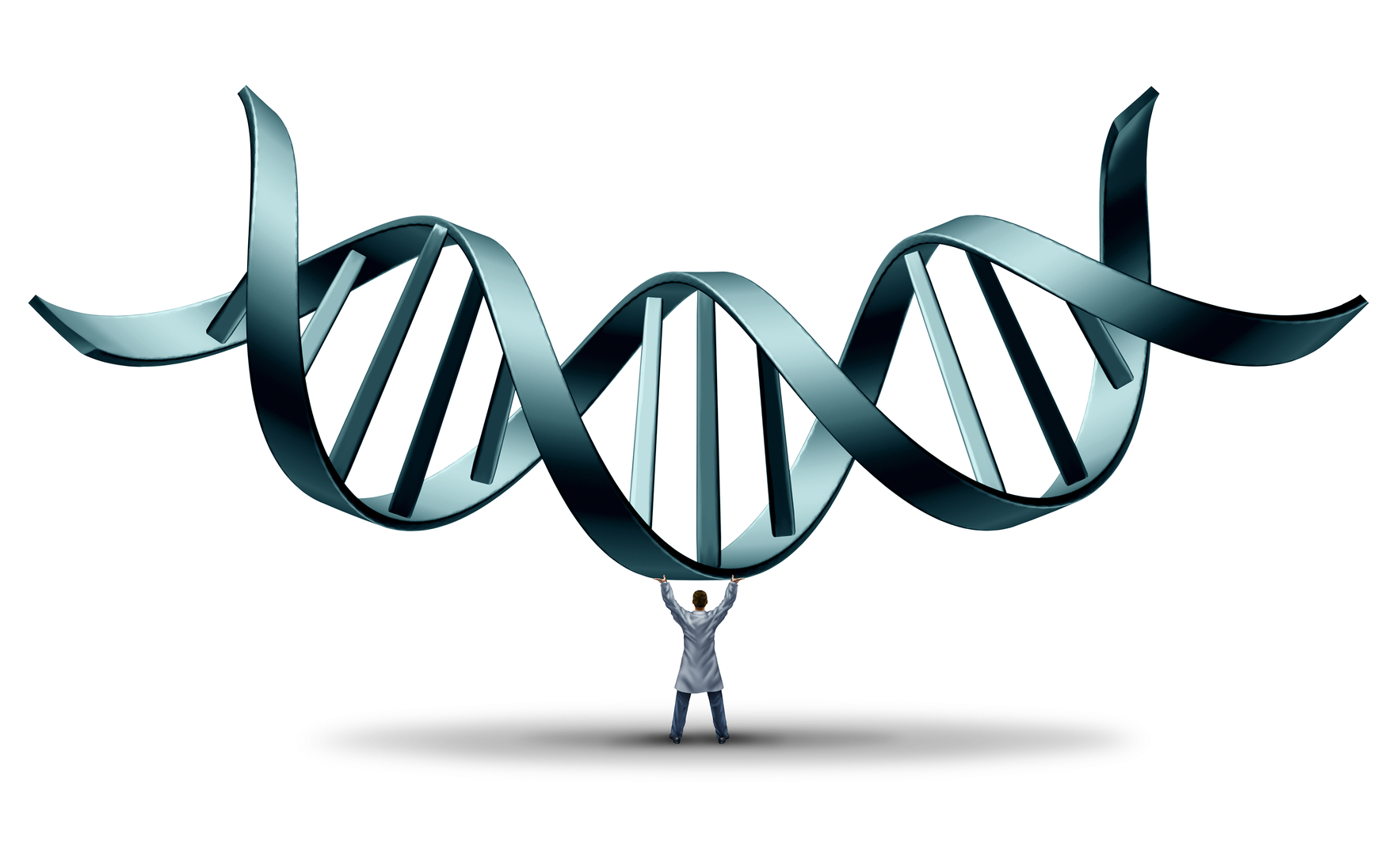
What does using AI to grow crops with less fertilizer have to do with healthcare?
Well, it has to do with researchers using machine learning to pore through vast amounts of genomic data to predict how organisms respond to changes in nutrition, toxins and pathogen exposure. Their findings, according to a new study published in Nature Communications, can help drive crop improvement, but also enhance disease prognosis, epidemiology, and public health.
Specifically, noted a statement released with the report, as a proof-of-concept, the researchers demonstrated that “genes whose responsiveness to nitrogen are evolutionarily conserved between two diverse plant species—Arabidopsis, a small flowering plant widely used as a model organism in plant biology, and varieties of corn, America’s largest crop—significantly improved the ability of machine learning models to predict genes of importance for how efficiently plants use nitrogen.”
In other words, explained Gloria Coruzzi, Carroll & Milton Petrie Professor in NYU’s Department of Biology and Center for Genomics and Systems Biology and the paper’s senior author, “We show that focusing on genes whose expression patterns are evolutionarily conserved across species enhances our ability to learn and predict ‘genes of importance’ to growth performance for staple crops, as well as disease outcomes in animals.”
By focusing on the “genes of importance” to a particular trait, the researchers say they proved that this “evolutionarily informed machine learning approach” can be applied to other traits and species by predicting additional traits in plants, including biomass and yield in both Arabidopsis and corn. They also showed that this approach can predict genes of importance to drought resistance in another staple crop, rice, as well as disease outcomes in animals through studying mouse models.
“Because we showed that our evolutionarily informed pipeline can also be applied in animals, this underlines its potential to uncover genes of importance for any physiological or clinical traits of interest across biology, agriculture, or medicine,” said Coruzzi.
Added study author Ying Li, faculty in the Department of Horticulture and Landscape Architecture at Purdue University, “Many key traits of agronomic or clinical importance are genetically complex and hence it’s difficult to pin down their control and inheritance. Our success proves that big data and systems level thinking can make these notoriously difficult challenges tractable.”
Photo by wildpixel/Getty Images