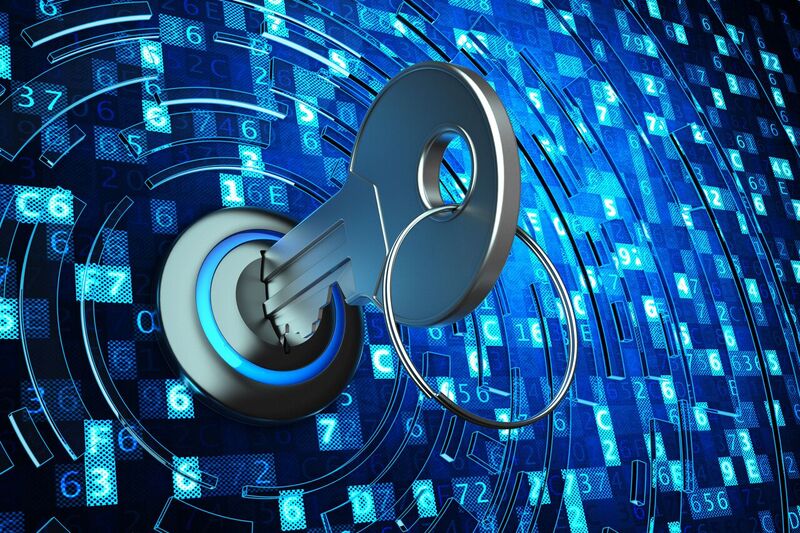
It’s been much noted that the COVID-19 pandemic has lead healthcare organizations to engage in a bit of an old-fashioned gold rush when it comes to calling on new AI to help beat back the virus.
But as the pandemic wanes and stakeholders have a chance to catch their breath, how should they view AI moving forward.
In a recent Forbes commentary, tech entrepeneur Alexander Polyakov says “AI is too powerful a tool to not use for the good,” but he quickly adds the caveat that while AI is bound to be an increasingly prevalent tool, the industry needs to focus on AI security to ensure the longterm benefits outweigh the risks.
“At present, there isn’t a single machine learning model that is completely secure,” he warns ominously. “Researchers all over the world are crafting attacks and safeguards to get ahead of malicious users. Attacks manipulate data, retrain models, embed secret features (backdoors) into models and, most commonly, perturb inputs — all with the intention of making a model produce unexpected results. And, to be clear, there is no model that has not been successfully attacked.”
What that means is that healthcare data is at risk both on the clinical side – meaning, for example, the ease with which data such as medical images can be corrupted – and on the personal side – meaning the risk to patient financial and personal details.
The immediate risk is compounded, Polyakov argues, by the fact that “it is not the direct fallout that poses the most danger. It is the mistrust for AI that would inevitably accumulate with every data leak and misdiagnosis, even if those were less frequent than human errors. The reason behind the double standard is our natural wariness of everything new, in particular the things that we don’t completely understand.”
So what to do?
Polyakov’s intention is primarily to raise awareness, so he doesn’t go into a lot of detail when it comes to solutions.
That said, he does point to “some of the more conventional and well-established security methods,” such as ubiquitous encryption, the use of “AI-specific anomaly detection systems,” and multi-tier security measures including “tests for security vulnerabilities to predict threats, safeguard models to prevent attacks, systems that detect malicious inputs and, lastly, regular reviews of security and defense measures to respond to adversaries.”
At a minimum, he says, when used in combination with state-of-the-art AI attack detectors, these steps could provide what he dubs “reasonable” protections for newly implemented AIs.
“I stress ‘reasonable,’” he concedes, “because as of right now there isn't a machine learning model or a defense that is impervious to attacks. And there is no reason to think there ever will be.”