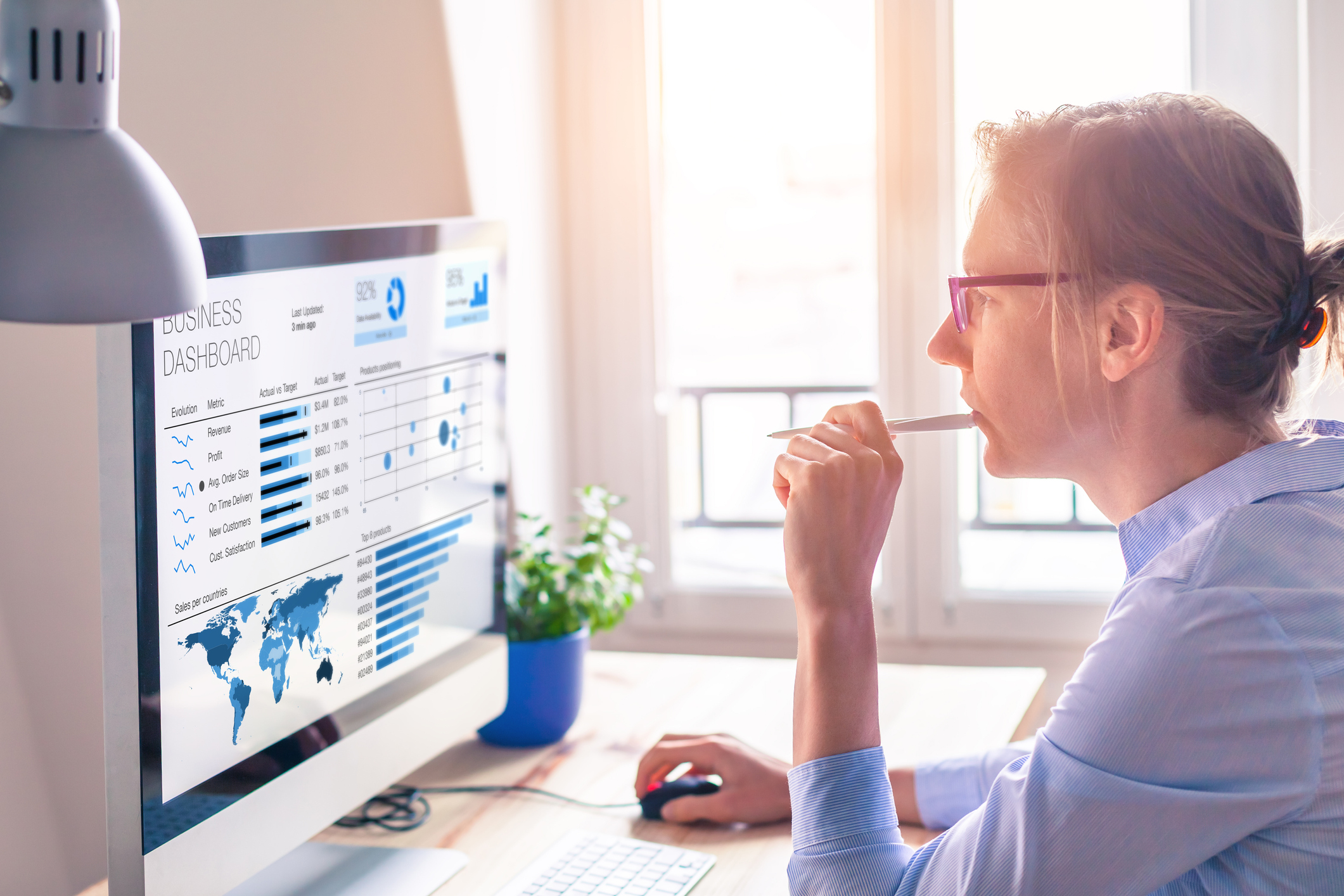
What is the biggest barrier to the adoption of AI in healthcare?
There are perhaps a number of answers to that question, depending on what part of the healthcare sector one is asking about, but according to one stakeholder, surveys show the ironic possibility that what is inhibiting the spread of data-dependent AI is: data.
In a recent commentary, Lauren Maffeo, associate principal analyst at GetApp, an online software review organization, notes that while currently fewer than 4 percent of all physician-patient interactions involve AI by 2023, that percentage will increase to 20, and presumably move up quickly from there.
Indeed, she notes, given the pressure on industry stakeholders to meet the Triple Aim of reduced costs, better outcomes and greater patient satisfaction, the “silver bullet” hype often surrounding AI is certain to increase expectations.
But just as the internal combustion engine doesn’t combust too well with dirty fuel, AI isn’t going to come close to meeting those expectations if the data aren’t up to necessary standards.
In November 2018, Maffeo says, the International Data Corporation (IDC) published a report assessing the “data-readiness” of four industries (including healthcare) on a scale of 1 (Critical) to 5 (Optimis]zed). “IDC ranked the healthcare sector at 2.4, reporting that 60 percent of survey respondents in the healthcare sector lack a data strategy.”
Moreover, her company’s own research echoed IDC’s findings just a few months ago. In April 2019, in response to a GetApp survey exploring how business leaders across five sectors make decisions using data, respondents in the healthcare sector had the highest utilization rate, meaning that they were least likely to have 10 percent or less of their data go unused.
“But,” Maffeo continues, “when we asked respondents which aspect of the data analytics process makes them feel the least confident, 25 percent of respondents in healthcare said they felt the least confident in their ability to collect relevant data. Indeed, respondents in healthcare displayed lower levels of confidence than their peers in accounting/finance, IT/tech, sales, and marketing.
In fact, across all five sectors –including healthcare — data quality was their key concern.
In short, Maffeo says, before the healthcare sector “can adopt AI en masse, it must start by improving the quality and relevancy of the data it would use to train AI systems. Without a high amount of quality data to train such systems, there’s no hope of building AI into clinical workflows.”
And without building AI into clinical workflows, the technology’s greatest promise is likely to go unrealized.