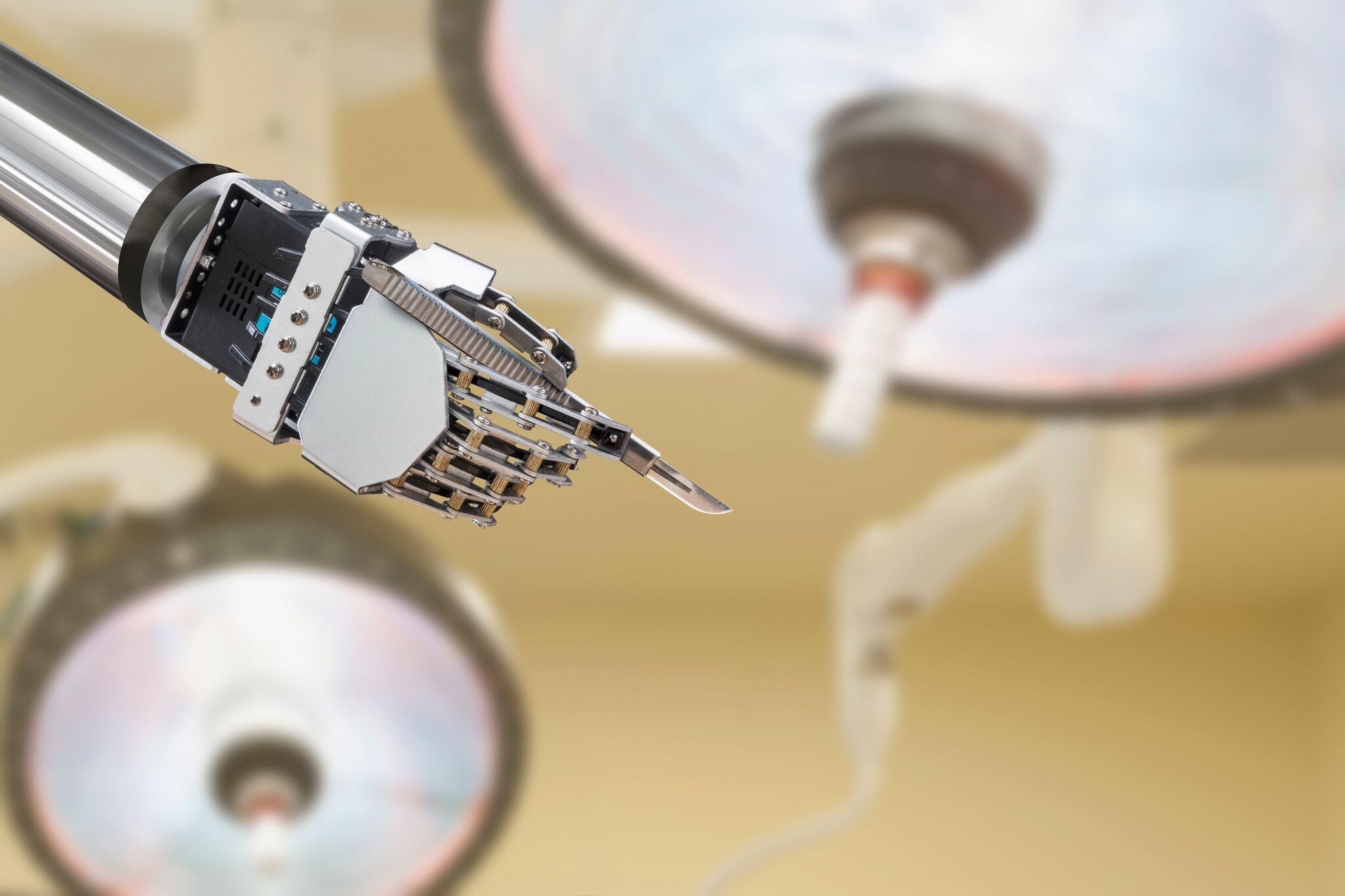
AI is going to have the same holistic impact on medicine that the X-ray did beginning a hundred years ago, only more so. And to guide that impact, hospitals need clinical AI departments as much as radiology departments.
So argues John Frownfelter, MD, an internist and CMIO at Jvion, in a recent commentary at HIT Consultant. In his view, radiology was actually “one of the earliest use cases for AI in medicine today,” and now AI is poised to help once again via machine learning algorithms that “can learn to detect tumors and other malignancies that are, in many cases, too subtle for even a trained radiologist to perceive.”
But AI will do much more than enhance the efforts of radiologists. For example, says Frownwelter, “depending on the data it is trained on, AI can predict a wide range of medical outcomes, from sepsis and heart failure to depression and opioid abuse. As more of patients’ medical data is stored in the EHR, and as these EHR systems become more interconnected across health systems, AI will only become more sensitive and accurate at predicting a patient’s risk of deteriorating.”
The possibilities go even further, according to Frownfelter, and while he lists quite a few of them his real point is that AI won’t realize its full potential over the long run without healthcare organizations taking steps now to provide it with the necessary institutional support in the form of clinical AI departments.
As he sums up the challenge, “for all its promise, it’s important to recognize that AI is as complex a tool as any radiological instrument. Healthcare organizations can’t just install the software and expect results. There are several implementation considerations that, if poorly executed, can doom AI’s success. This is where clinical AI departments can and should play a role.”
First, he says, newly established clinical AI departments need to focus getting and using good data. “Ultimately, the data used to train machine learning models should be relevant and representative of the patient population it serves,” he argues. “Failing to do so can limit AI’s accuracy and usefulness, or worse, introduce bias. Any bias in the training data, including pre-existing disparities in health outcomes, will be reflected in the output of the AI.”
More broadly, Frownwelter notes that uses for clinical AI will vary from hospital to hospital, so they each “will need to deeply consider their patient population and make sure that they have the resources to tailor vendor solutions accordingly. Without the right resources and organizational strategies, clinical AI adoption will come with the same frustration and disillusionment that has come to be associated with EHRs.”
Finally, he argues, “coordination is the bedrock of quality care, and AI is no exception. Clinical AI departments can foster collaboration across departments to action AI insights and treat the whole patient. Doing so can promote a shift from reactive to preventive care, mobilizing ambulatory, and community health resources to prevent avoidable hospitalizations.”
When the pandemic is finally over, says Frownwelter, hospitals will need to take steps to recover financially while reshaping their practices to deliver better care in the future.
And the time to get ready is now.