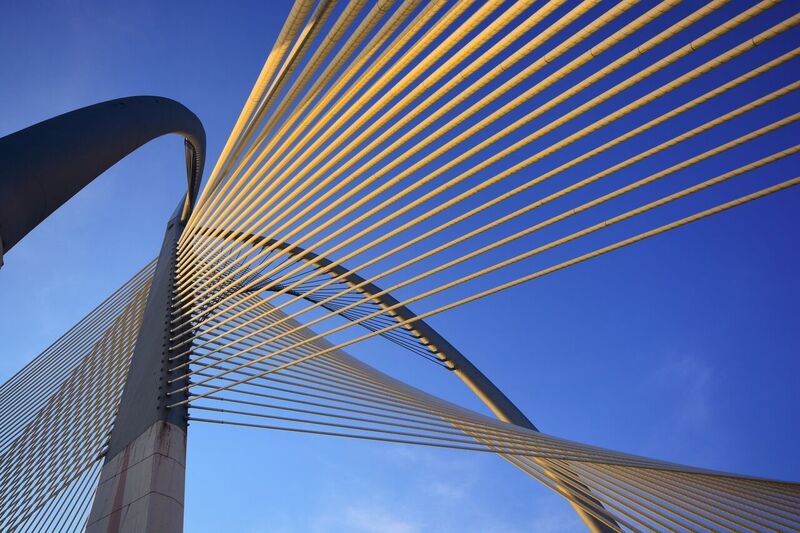
If you want to train your brain – and, by extension, new AI – vary the stimuli.
That’s one admittedly simplified way of summing up a recent study by researchers at Imperial College London in which, by varying neural network cell properties, they determined that the brain learns faster and uses less energy when there is a greater diversity of neurons being stimulated.
“The brain needs to be energy efficient while still being able to excel at solving complex tasks,” explained first author Nicolas Perez, a PhD student at Imperial College London’s Department of Electrical and Electronic Engineering. “Our work suggests that having a diversity of neurons in both brains and AI fulfils both these requirements and could boost learning.”
In their report, published at Nature Communications, the researchers noted that “previous studies have largely used simplified tasks or networks, and it remains unknown whether or not heterogeneity can help animals solve complex information processing tasks in natural environments.”
Their goal, then, was “to investigate the effect of introducing heterogeneity in the time scales of neurons when performing tasks with realistic and complex temporal structure. We found that it improves the overall performance, makes learning more stable and robust, and that the network learns neural parameter distributions that match experimental observations, suggesting that the heterogeneity observed in the brain may be a vital component of its ability to adapt to new environments.”
For the study, the researchers focused on tweaking the “time constant”, or how quickly each cell decides what it wants to do based on what the cells connected to it are doing. Some cells will decide very quickly, looking only at what the connected cells have just done. Other cells will be slower to react, basing their decision on what other cells have been doing for a while.
After varying the cells’ time constants, they tasked the network with performing some benchmark machine learning tasks: classifying images of clothing and handwritten digits, recognizing human gestures, and identifying spoken digits and commands. The results show that by allowing the network to combine slow and fast information, it was better able to solve tasks in more complicated, real-world settings.
Moreover, the researchers noted, when they changed the amount of variability in the simulated networks, they found that the ones that performed best matched the amount of variability seen in the brain, suggesting that the brain may have evolved to have just the right amount of variability for optimal learning.
Said Dr Dan Goodman, also of Imperial’s Department of Electrical and Electronic Engineering, “Evolution has given us incredible brain functions – most of which we are only just beginning to understand. Our research suggests that we can learn vital lessons from our own biology to make AI work better for us.”
Photo by AzriSuratmin/Getty Images