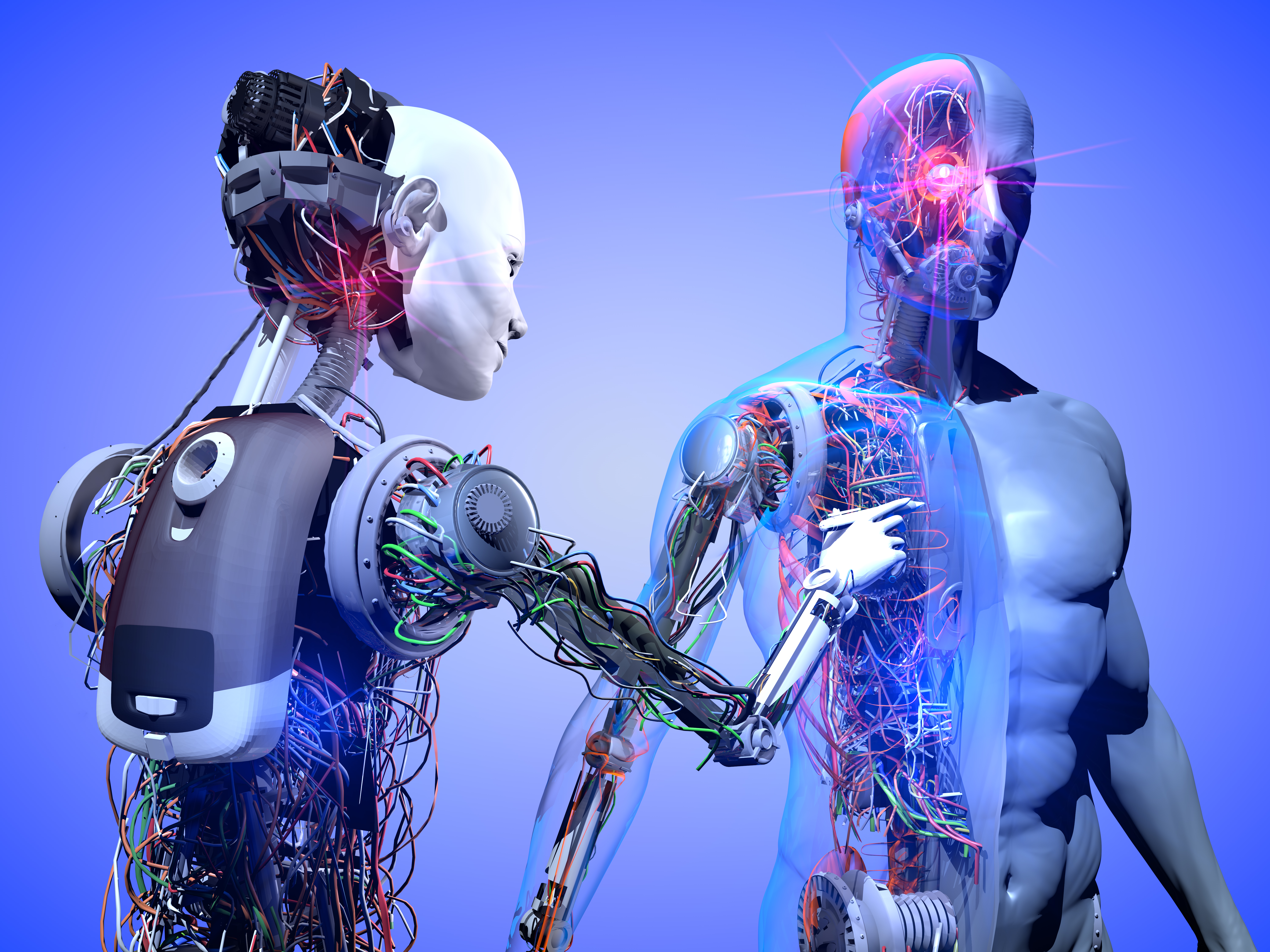
Given the all-hands-on-deck nature of the battle against the coronavirus, over the last year, it’s not surprising that many researchers and providers tried to enlist new AI tools to help them grapple with the virus.
According to a new study, however, machine learning diagnostic tools remain largely inaccurate ways of identifying the disease because of methodological flaws or underlying biases.
For the study, which was published recently in Nature Machine Intelligence, researchers led by the University of Cambridge identified 2,212 studies for potential review. Of those, 415 were included after initial screening and, after quality screening, 62 studies were included in the systematic review.
“The international machine learning community went to enormous efforts to tackle the COVID-19 pandemic using machine learning,” said joint senior author Dr James Rudd, from Cambridge’s Department of Medicine, in a statement. “These early studies show promise, but they suffer from a high prevalence of deficiencies in methodology and reporting, with none of the literature we reviewed reaching the threshold of robustness and reproducibility essential to support use in clinical practice.”
In particular, researchers noted five design flaws in many of the tools reviewed:
- the bias in small datasets;
- the variability of large internationally sourced datasets;
- the poor integration of multistream data, particularly imaging data;
- the difficulty of the task of prognostication;
- the necessity for clinicians and data analysts to work side-by-side to ensure the developed AI algorithms are clinically relevant and implementable into routine clinical care.
“(A)ny machine learning algorithm is only as good as the data it’s trained on,” said first author Dr Michael Roberts from Cambridge’s Department of Applied Mathematics and Theoretical Physics. “Especially for a brand-new disease like COVID-19, it’s vital that the training data is as diverse as possible because, as we’ve seen throughout this pandemic, there are many different factors that affect what the disease looks like and how it behaves.”
Despite the flaws they found in the COVID-19 models, the researchers noted that with some key modifications, machine learning can be a powerful tool in combatting the pandemic. For example, they caution against naive use of public datasets, which can lead to significant risks of bias. In addition, datasets should be diverse and of appropriate size to make the model useful for different demographic group and independent external datasets should be curated.
“The intricate link of any AI algorithm for detection, diagnosis or prognosis of COVID-19 infections to a clear clinical need is essential for successful translation,” the researchers concluded. “As such, complementary computational and clinical expertise, in conjunction with high-quality healthcare data, are required for the development of AI algorithms.”