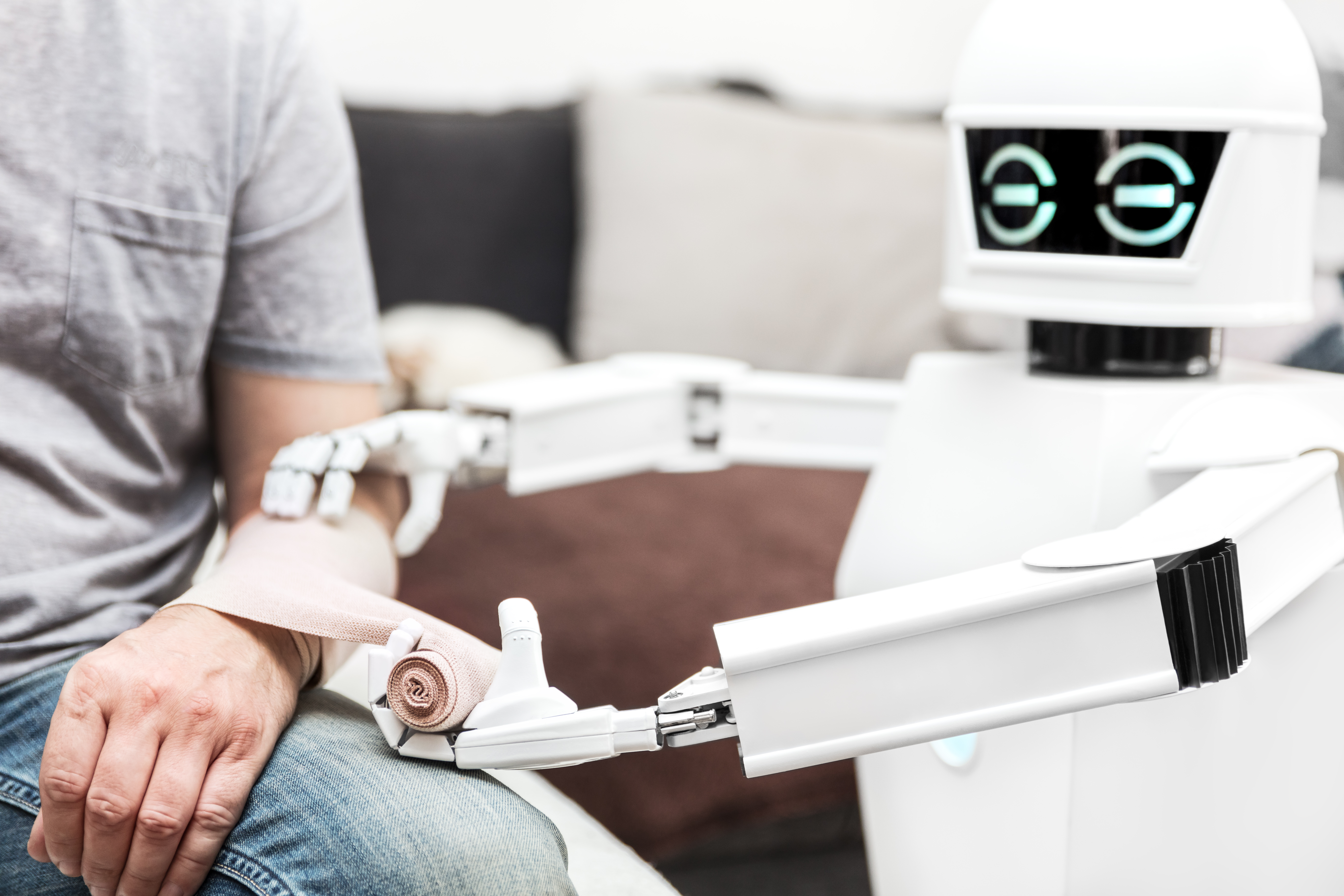
Among the challenges clinicians encounter when monitoring the progression of multiple-sclerosis disease in patients 50 or older is distinguishing between problems, such as gait issues, related to MS and those caused by other age-related issues.
According to a new study published in Institute of Electrical and Electronics Engineers Transactions on Biomedical Engineering, however, machine learning just might help. While MS can present itself in many ways, walking problems are a common symptom and about half of patients need walking assistance within 15 years of disease onset. With that in mind, researchers at the University of Illinois Urbana Champaign decided to evaluate the effectiveness of a gait data-based ML tool for MS prediction.
“We wanted to get a sense of the interactions between aging and concurrent MS disease-related changes, and whether we can also differentiate between the two in older adults with MS,” said Manuel Hernandez, kinesiology and community health professor at University of Illinois.
“Machine learning techniques seem to work particularly well at spotting complex hidden changes in performance. We hypothesized that these analysis techniques might also be useful in predicting sudden gait changes in persons with MS.”
Using an instrumental treadmill, researchers collected gait data that was normalized for body size and demographics from 20 adults with MS, as well as 20 age-, weight-, height-, and gender-matched older adults without MS. Participants walked at a comfortable pace for up to 75 seconds while specialized software captured gait events, corresponding ground reaction forces, and center-of-pressure positions during each walk. The team then extracted each participant’s characteristic spatial, temporal, and kinetic features in their strides to examine variations in gait during each trial.
“Given that subtle and heterogeneous patterns of gait changes may arise in PwMS (Persons with MS) over time,” the team wrote, “a machine learning (ML) approach will be valuable for monitoring MS-related changes in older adults.”
Changes in various gait features enabled the team to identify differences in gait patterns between participants.
“We study the effectiveness of a gait dynamics-based machine-learning framework to classify strides of older persons with MS from healthy controls to generalize across different walking tasks and over new subjects,” said Rachneet Kaur, a graduate student at University of Illinois Urbana Champaign. “This proposed methodology is an advancement toward developing an assessment marker for medical professionals to predict older people with MS who are likely to have a worsening of symptoms in the near term.”
Future studies could provide more thorough examinations to manage the study’s small cohort size, the researchers noted.