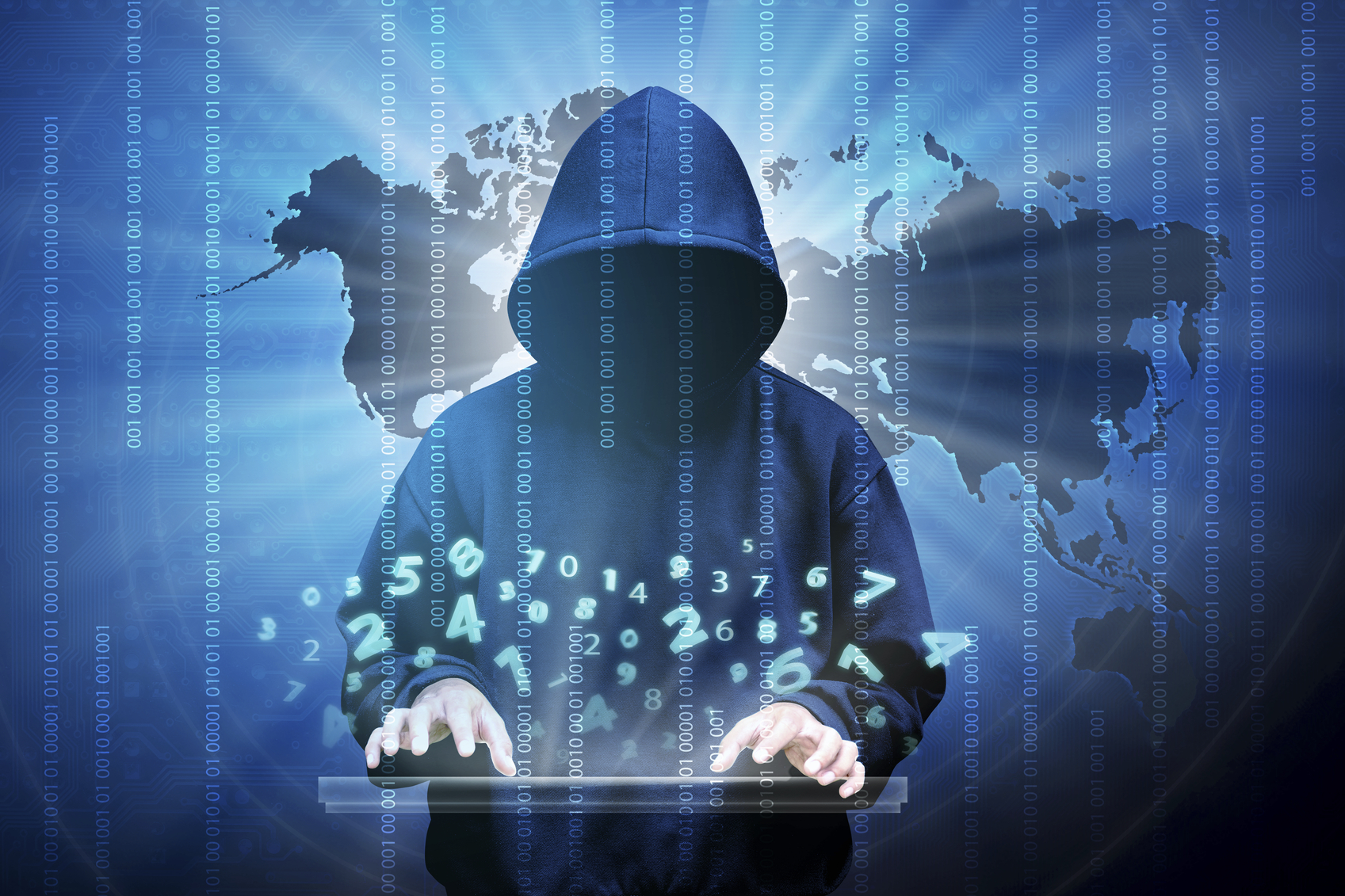
AI and machine learning are widely considered developments that could revolutionize medicine and healthcare, but as with any new technology it all comes with a potential dark side that highlights the importance of caution.
For example, a recent study by a team of Israeli researchers has demonstrated how easy it has become to use deep learning as a way to alter medical images to add realistic-looking cancerous tumors and perhaps often fool even the best radiologists.
In the wake of numerous cyber-attacks on clinics and hospitals, the team undertook to show “how an attacker can use deep-learning to add or remove evidence of medical conditions from volumetric (3D) medical scans.” The team explains how to successfully infiltrate a typical health system’s PACS infrastructure and alter MRI or CT scan images using malware based on a type of machine learning called generative adversarial networks (GANs) to inject fake tumors or remove real cancers from the patient data.
“Since 3D medical scans provide strong evidence of medical conditions, an attacker with access to a scan would have the power to change the outcome of the patient’s diagnosis,” the team explained. “For example, an attacker can add or remove evidence of aneurysms, heart disease, blood clots, infections, arthritis, cartilage problems, torn ligaments or tendons, tumors in the brain, heart, or spine, and other cancers.”
The researchers note that here are numerous motivations for conducting this type of attack. Hackers may wish to influence the outcome of an election or topple a political figure by prompting a serious health diagnosis, or they might alter images and hold the original data for ransom. Individuals could use the strategy to commit insurance fraud or hide a murder; researchers or drug developers could fake their data to confirm a desired result.
For the study, the researchers conducted a simulated attack on a real hospital’s systems using a common sub-$50 computer known as Raspberry Pi. While the participating hospital was fully aware of the team’s activities and consented to the experiment, the realism of the images was such that radiologists had a difficult time recognizing that the images had been altered, even though they knew the images may have been altered.
Moreover, when three experienced clinicians were not told that they were looking at images that included fake lung cancer tumors, they confirmed a cancer diagnosis 99 percent of the time.
“This paper demonstrates how we should be wary of closed world assumptions: both human experts and advanced AI can be fooled if they fully trust their observations,” the team stated. “We hope that this paper, and the supplementary datasets, help both industry and academia mitigate this emerging threat.”