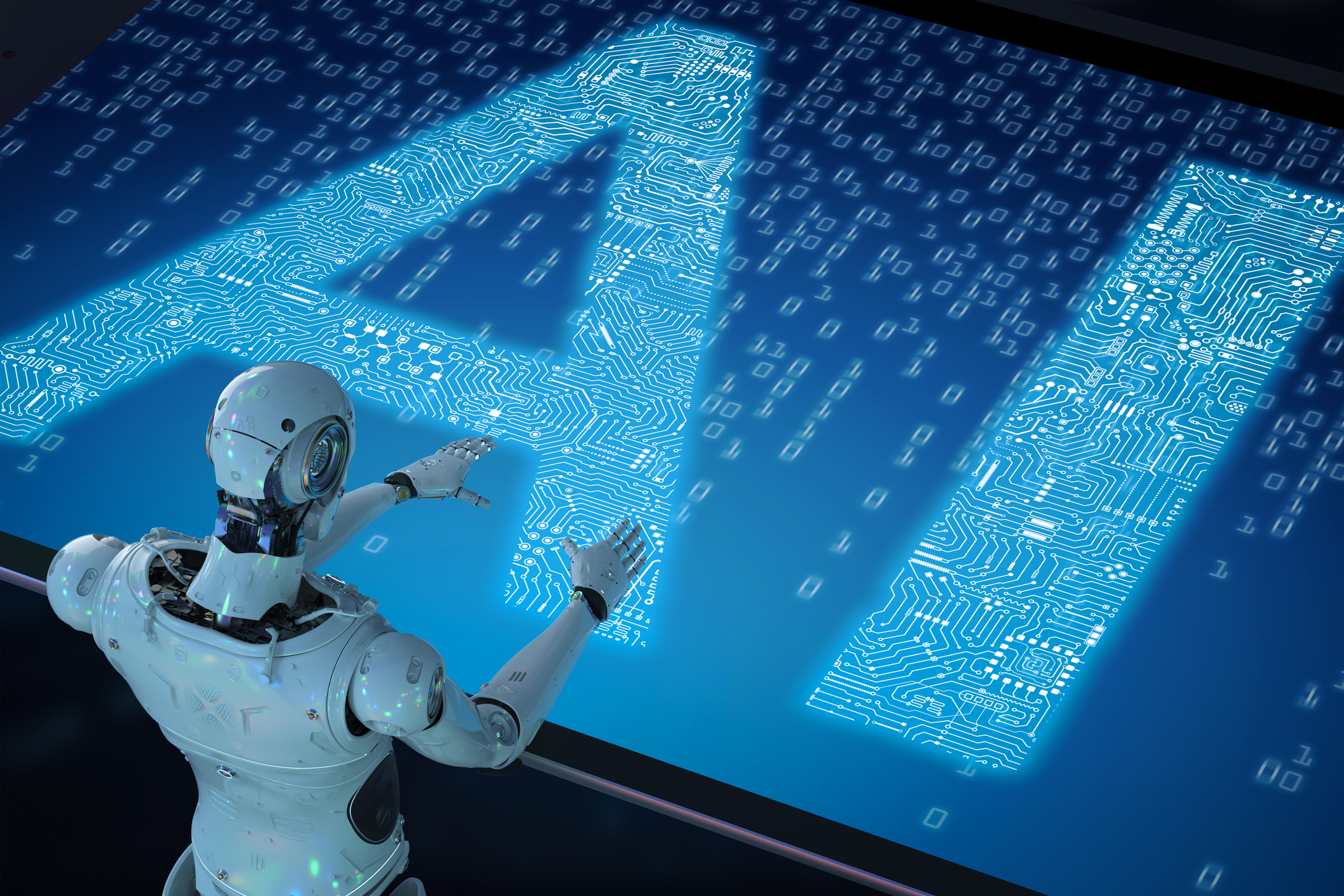
As applications for AI have been adopted in numerous sectors, including healthcare, there’s been growing concern of the potential for inequities to be introduced or perpetuated via the use of data sets which present perspectives that are skewed in a number of ways.
As a result, researchers and developers have focused eliminating or mitigating such bias by a series of adjustments to how AI and machine learning systems are trained. But that, in turn, has led to concerns about the resulting accuracy of the systems.
In an attempt to put these new concerns to rest, a team of researchers from Carnegie Mellon University (CMU) have published a new study by which they tested the assumption of diminished accuracy and, put succinctly, found it lacking.
“You don't have to sacrifice accuracy to build systems that are fair and equitable," Rayid Ghani, a professor in the CMU School of Computer Science's Machine Learning Department (MLD), summed up the team’s findings. "But it does require you to deliberately design systems to be fair and equitable. Off-the-shelf systems won't work.”
For the study, the team looked at situations where in-demand resources are limited, and machine learning systems are used to help allocate those resources. The researchers looked at systems in four areas: prioritizing limited mental health care outreach based on a person's risk of returning to jail to reduce reincarceration; predicting serious safety violations to better deploy a city's limited housing inspectors; modeling the risk of students not graduating from high school in time to identify those most in need of additional support; and helping teachers reach crowdfunding goals for classroom needs.
The upshot?
“In each setting studied,” the team wrote in their report, “explicitly focusing on achieving equity and using our proposed post-hoc disparity mitigation methods, fairness was substantially improved without sacrificing accuracy. This observation was robust across policy contexts studied, scale of resources available for intervention, time, and relative size of the protected groups. . . . Our results suggest that trade-offs between fairness and effectiveness can in fact be negligible in practice, suggesting that improvement in may be equity easier and more practical across a wide range of applications than is often expected.”
The team hopes their research will start to change the minds of fellow researchers and policymakers as they consider the use of machine learning in decision making.
"We want the artificial intelligence, computer science and machine learning communities to stop accepting this assumption of a trade-off between accuracy and fairness and to start intentionally designing systems that maximize both," said Kit Rodolfa, a research scientist in MLD. "We hope policymakers will embrace machine learning as a tool in their decision making to help them achieve equitable outcomes.”
Photo by Phonlamai Photo/Getty Images