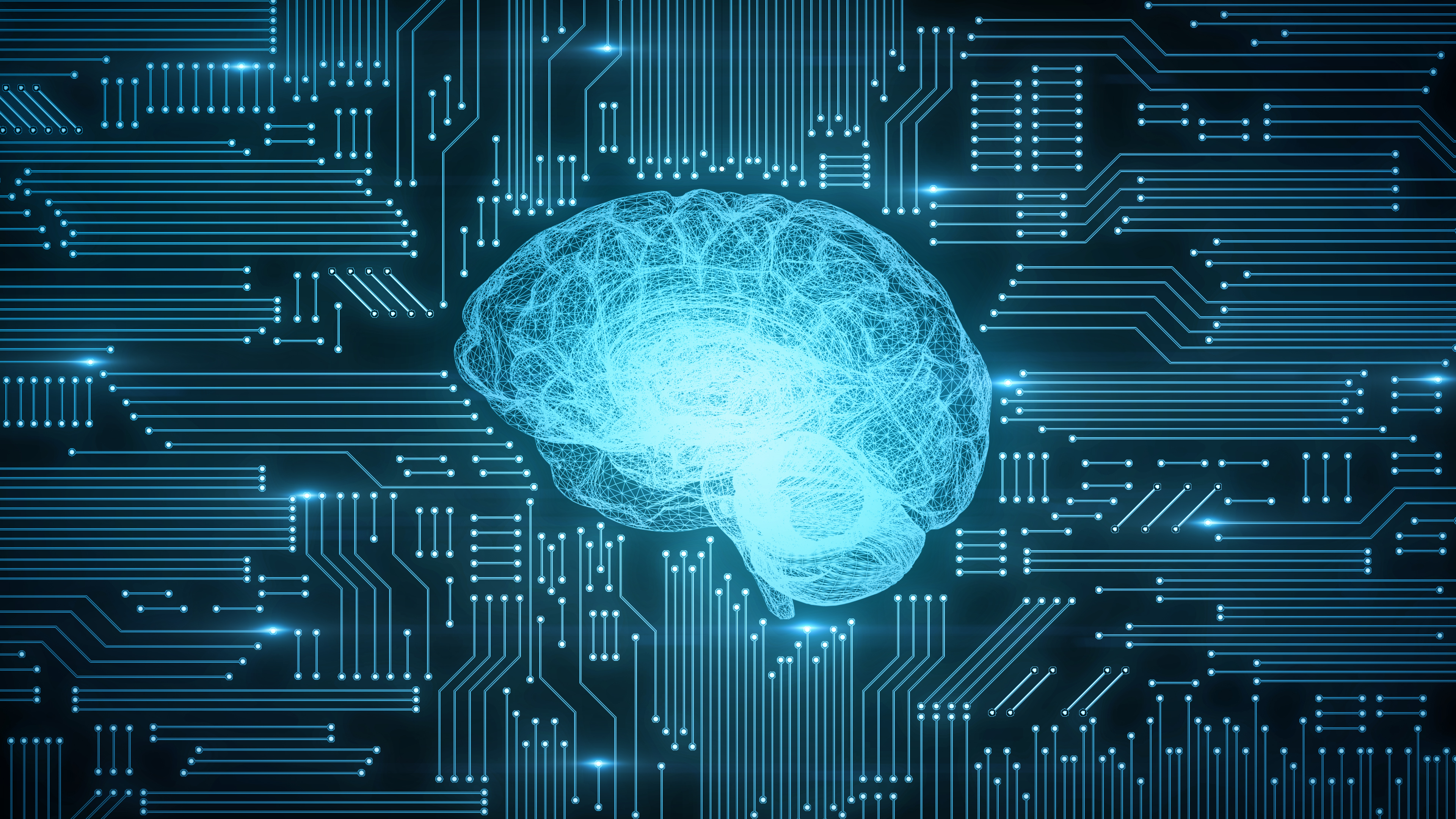
The diagnosis of unruptured brain aneurysms – bulges in blood vessels in the brain that can leak or burst open, potentially leading to stroke, brain damage or death – is arguably among the most time-critical diagnoses a doctor can make, but it often can mean sifting through hundreds of brain scans.
According to a recent study by researchers as Stanford University, a new AI tool should be able to help.
The AI tool, detailed in a paper published June 7 in JAMA Network Open, highlights areas of a brain scan that are likely to contain an aneurysm. Built around an algorithm called HeadXNet, the tool improved clinicians' ability to correctly identify aneurysms at a level equivalent to finding six more aneurysms in 100 scans that contain aneurysms. It also improved consensus among the interpreting clinicians, the researchers announced.
"There's been a lot of concern about how machine learning will actually work within the medical field," said Allison Park, a Stanford graduate student in statistics and co-lead author of the paper, in a statement. "This research is an example of how humans stay involved in the diagnostic process, aided by an artificial intelligence tool.”
Combing brain scans for signs of an aneurysm can mean scrolling through hundreds of images. Aneurysms come in many sizes and shapes and balloon out at tricky angles – some register as no more than a blip within the movie-like succession of images.
Deep learning, however, “has recently shown significant potential in accurately performing diagnostic tasks on medical imaging,” the researchers noted in their report. “Specifically, convolutional neural networks (CNNs) have demonstrated excellent performance on a range of visual tasks, including medical image analysis. Moreover, the ability of deep learning systems to augment clinician workflow remains relatively unexplored.”
"Search for an aneurysm is one of the most labor-intensive and critical tasks radiologists undertake," said Kristen Yeom, associate professor of radiology and co-senior author of the paper. "Given inherent challenges of complex neurovascular anatomy and potential fatal outcome of a missed aneurysm, it prompted me to apply advances in computer science and vision to neuroimaging.”
Yeom brought the idea to the AI for Healthcare Bootcamp run by Stanford's Machine Learning Group.
As the report noted, “given the potential catastrophic outcome of a missed aneurysm at risk of rupture, an automated detection tool that reliably detects and enhances clinicians’ performance is highly desirable. Aneurysm rupture is fatal in 40% of patients and leads to irreversible neurological disability in two-thirds of those who survive; therefore, an accurate and timely detection is of paramount importance. In addition to significantly improving accuracy across clinicians while interpreting CTA examinations, an automated aneurysm detection tool, such as the one presented in this study, could also be used to prioritize workflow so that those examinations more likely to be positive could receive timely expert review, potentially leading to a shorter time to treatment and more favorable outcomes.”
The machine learning methods at the heart of HeadXNet could likely be trained to identify other diseases inside and outside the brain, the team noted, but but they added that a considerable hurdle remains in integrating any artificial intelligence medical tools with daily clinical workflow in radiology across hospitals.