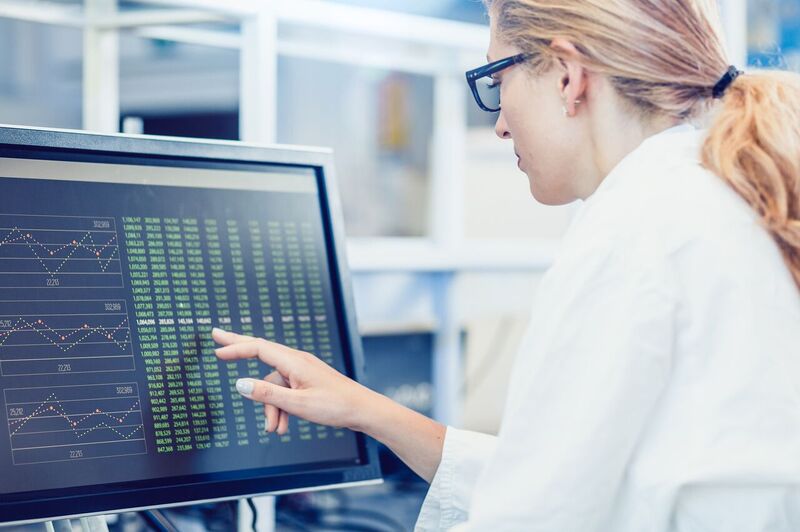
How much progress could we make against cancer if we could grow virtual tumors then study them and figure out how best to treat them?
Scientists in Europe have brought us closer to finding out the answer to that question with a new AI-based platform that allows them to grow tumors then automatically optimize the design of nanoparticles to treat them.
Working under the auspices of the European cooperative project known as EVONANO, the team, led by Dr. Igor Balaz of Serbia’s University of Novi Sad, have published a paper in the Nature journal Computational Materials that describes their efforts to reduce the time and cost of developing therapeutic nanoparticle designs.
“The nanoparticle designs are optimised using machine learning to efficiently find the most effective anti-cancer treatments,” the team wrote. “We demonstrate EVONANO with two examples optimising the properties of nanoparticles and treatment to selectively kill cancer cells over a range of tumour environments. Our platform shows how in silico models that capture both tumour and tissue-scale dynamics can be combined with machine learning to optimise nanomedicine.”
According to the team, drugs incorporated onto nanoparticles, which are tiny vehicles that can be engineered to transport drugs to tumors, have the potential for improved targeting of cancer cells because their design changes their ability to move in the body and correctly target cancer cells. For example, a bioengineer might change the size, charge or material of the nanoparticle, coat the nanoparticles with molecules that make them easy to recognize by cancer cells, or load them with different drugs to kill cancer cells.
“We are still at the early stages of making virtual tumours, given the complex nature of the disease, but the hope is that even these simple digital tumours can help us more efficiently design nanomedicines for cancer,” said Dr. Sabine Hauert, of the UK’s University of Bristol, in a statement. “In the future, creating a digital twin of a patient tumour could enable the design of new nanoparticle treatments specialised for their needs, without the need for extensive trial and error or laboratory work, which is often costly and limited in its ability to quickly iterate on solutions suited for individual patients.”
The strategy identified nanoparticle designs that were known to work in previous research, as well as potential new strategies for nanoparticle design.
“The tool we developed in EVONANO represents a rich platform for testing hypotheses on the efficacy of nanoparticles for various tumour scenarios,” explained Dr. Balaz. “The physiological effect of tweaking nanoparticle parameters can now be simulated at the level of detail that is nearly impossible to achieve experimentally.”
Moving forward, the team aims to use such a platform to bring digital twins closer to reality by using data from individual patients to grow virtual versions of their tumors, and then optimize treatments that are right for them. In the nearer term, the platform will be used to discover new nanoparticle strategies that can be tested in the laboratory.
Photo by sanjeri/Getty Images