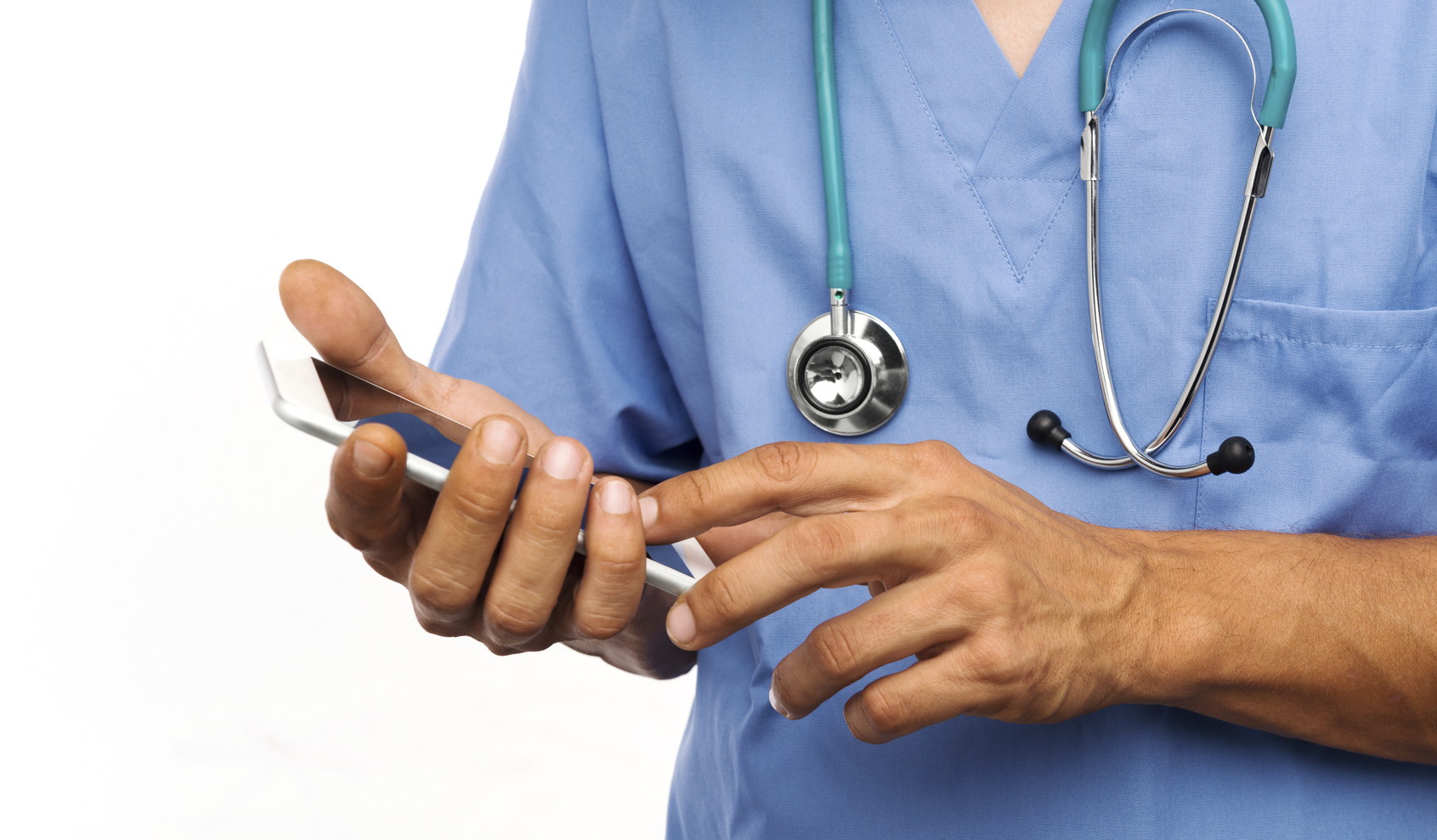
How much oxygen will a patient need while the hospital as they fight to recover from COVID-19?
That’s a critical question hospitals around the world are facing as they struggle to keep up with the pandemic, and researchers on a new study have tapped AI to help providers come up with an answer.
Centered at the UK’s Addenbrooke’s Hospital in Cambridge, the team used the technique known as “federated learning (FL),” which is a method used for training AI models with data from multiple sources, to develop an algorithm that analyzed chest x-rays and electronic health data from hospital patients with Covid symptoms.
Once the algorithm had ‘learned’ from the data, the analysis was brought together to build an AI tool which could predict the oxygen needs of hospital Covid patients anywhere in the world.
Published recently in Nature Medicine, the study, dubbed EXAM, is reportedly one of the largest, most diverse clinical federated learning studies to date.
According to a statement, to check the accuracy of EXAM, the researchers tested it in a number of hospitals across five continents, including Addenbrooke’s Hospital. The results showed it predicted the oxygen needed within 24 hours of a patient’s arrival in the emergency department, with a sensitivity of 95 per cent and a specificity of over 88 per cent.
“Federated learning has transformative power to bring AI innovation to the clinical workflow,” said Professor Fiona Gilbert, who led the study in Cambridge and is honorary consultant radiologist at Addenbrooke’s Hospital and chair of radiology at the University of Cambridge School of Clinical Medicine.
Indeed, according to the report, the team believes they have provided “a powerful proof-of-concept of the feasibility of using FL for fast and collaborative development of needed AI models in healthcare. Our study involved multiple sites across four continents and under the oversight of different regulatory bodies, and thus holds the promise of being provided to different regulated markets in an expedited way. The global FL model, EXAM, proved to be more robust and achieved better results at individual sites than any model trained on only local data.”
Bringing together collaborators across North and South America, Europe and Asia, the EXAM study took just two weeks of AI ‘learning’ to achieve high-quality predictions.
“Federated Learning allowed researchers to collaborate and set a new standard for what we can do globally, using the power of AI,'' said Dr Mona G Flores, Global Head for Medical AI at NVIDIA, which also participated in the study. “This will advance AI not just for healthcare but across all industries looking to build robust models without sacrificing privacy.”
The outcomes of around 10,000 COVID patients from across the world were analyzed in the study.
Photo by Rouzes/Getty Images