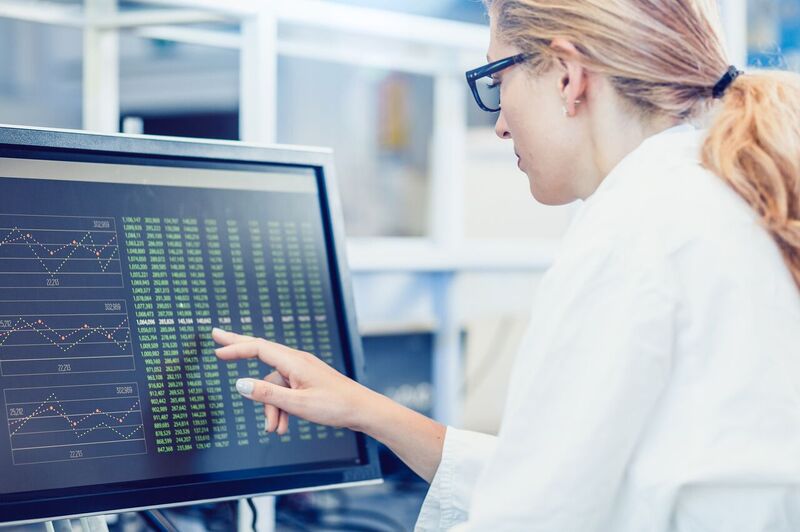
EHRs are a potential treasure trove of information beyond the needs and circumstances of specific patients, but who has time to pore through them to find it?
It turns out, perhaps, another machine. That’s the thinking behind a study published recently at npj Digital Medicine that specifically explored using deep machine learning methods to extract information regarding the post-surgical safety performance of implanted medical devices, in this case using hip replacements as a test case.
To be sure, any medical device has to meet safety standards set by the FDA, but as Nigam Shah, PhD, associate professor of medicine and biomedical data science at Stanford and the study’s lead author, pointed out, “The safety standards required by the FDA are for initial approval of the device’s use. What we need is a scalable way — beyond self-reporting — to see how safe and effective these devices are in a population after years of use.”
The primary source of the answer to that question lie in EHRs that detail the experiences of real-world patients, so Shah and colleagues worked out a way to mine them. Part of the challenge stems from the fact that patient data is spread across multiple databases, making it difficult to discern patterns of use for any specific device. Shah and his team addressed that challenge by accessing patient data stripped of personal identifying information.
While this study focused on hip replacements, there are several other widely used devices for which this approach should be appropriate, including pacemakers, joint replacements, breast implants, and more modern devices, such as insulin pumps and spinal cord stimulators.
According to the report, the algorithm is designed to link up certain medical characteristics — things like infection rates, how long an implant lasts before it needs to be replaced, and levels of patient pain — with specific implantable devices. Shah and his team chose to follow hip implants as they’re one of the most common medical devices.
Another team member, Stanford research scientist Alison Callahan, noted that the algorithm accurately and efficiently identified complication events with each patient’s implant while also explained pointing to a powerful predictor of complication: pain.
“Previous studies have likewise shown that pain is a useful predictor for more serious complications later on,” she said. By tracking patient pain levels over time, the model helped reveal a patient’s quality of life with a specific implant, while by compiling data from many patients, the algorithm also provided a broader picture of complications and pain levels associated with each device.