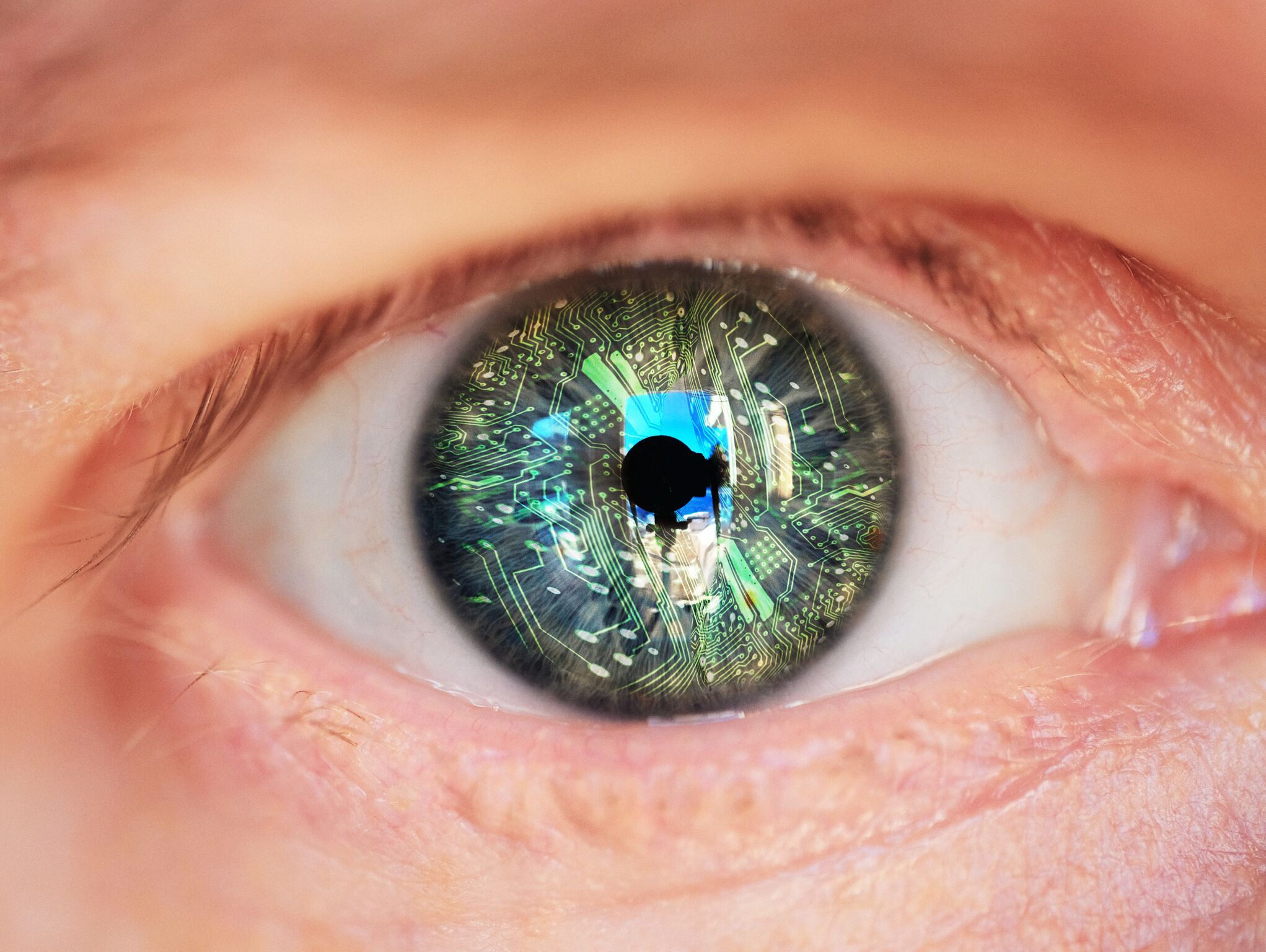
To what extent can brain diseases be detected by analyzing the movement of a person’s eyes?
According to scientists, to quite a significant extent, actually, and to that end scientists at the Kavli Institute for Systems Neuroscience at the Norwegian University of Science and Technology (NTNU) in Trondheim have used AI to develop an eye tracker that analyzes images from MRI brain scans to recognize patterns that are shared across people.
“Our viewing behaviour is an expression of our thoughts, memories and goals at any given moment in time,” said Matthias Nau, one of the scientists involved in the project. “Brain diseases manifest themselves as characteristic eye movement patterns and disturbances in viewing behaviour. Almost every cognitive or neural disorder, such as working memory deficits, amnesia, Parkinson’s disease and Alzheimer’s disease will affect your viewing behaviour.”
The tool, dubbed DeepMReye, was trained using MRI datasets that enabled it to figure out the complicated task of generalizing patterns of gaze across people, the team reported in a statement. “In a process involving convolutions that extract features of the data through dimensionality reduction, the artificial intelligence had learned how to use the vast pool of knowledge it had been fed, to extract and interpret the hidden meaning from any person’s viewing behaviour.”
The scientists noted that AI’s ability to generalize knowledge to new individuals, combined with the fact that all the data the AI requires for analysis is already found in most MRI maps, brings new life and interest to old brain scanning data.
“Researchers can use this model to analyse retrospectively from 20 years of existing fMRI data, from thousands of thousands of subjects, and ask completely new questions,” Nau said.
DeepMReye can also be used for patient groups and in categories of research that previouis camera-based eye tracking did not support. While camera eye tracking could only collect information from subjects when their eyes were open, the MR signal locates eyeball movement patterns even when the eyelid is closed. This makes the DeepMReye model relevant for sleep labs, for instance by studying eye movements to classify sleep stages.
Another opportunity, said the scientists, is clinical health screenings of congenitally blind people, who previously have been excluded from eye tracking studies because the camera calibration process requires a seeing and focusing eye. DeepMReye does not discriminate between seeing and blind eyes when it comes to health screening.
While there is general agreement between scientists and clinicians that eye tracking is an important measure for investigating cognition and diseases, Nau said the process hasn’t been widely used because “the existing camera eye-tracking procedure is quite expensive, it is tricky to use, and way too time consuming. We don't have the time, the clinical routine, or the expertise to use these cameras for every single patient or research subject.”
The scientists hope their new AI-based approach will change that.
Photo by Daniel Haug/Getty Images