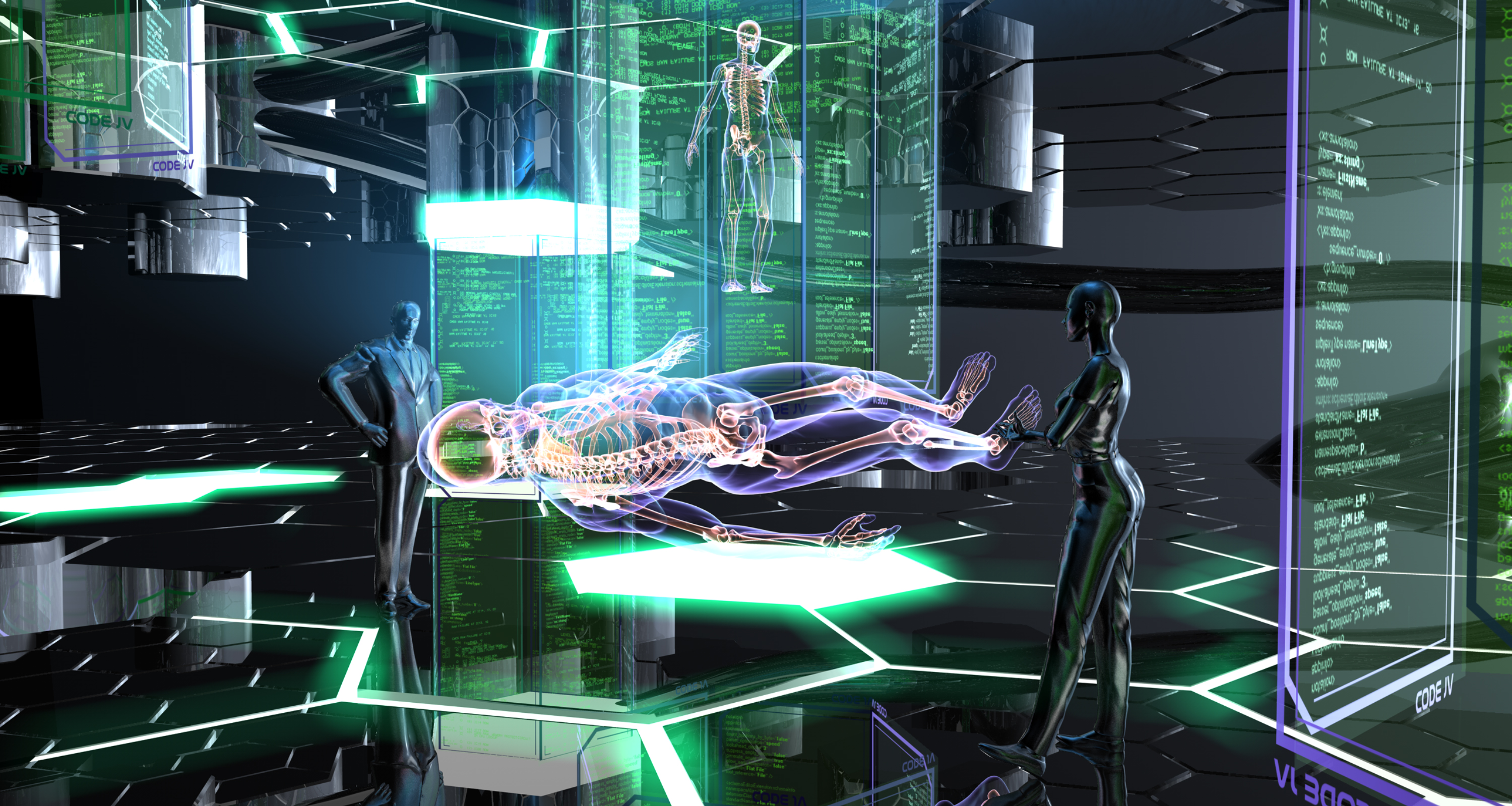
One of the many challenges of the COVID-19 pandemic has been determining how best to determine whether patients with the virus are going to need hospitalization, and at what level.
But a recent study by researchers at the NYU Grossman School of Medicine, among other affiliations, has applied AI to EHR data to help doctors and hospitals more accurately manage patients who have tested positive.
“Telling a provider that someone might need an ICU bed isn’t useful, because ICU beds are already limited to patients in immediate need of higher levels of care. Predicting future needs wouldn’t change clinical management,” Yindalon Aphinyanaphongs, MD, PhD, assistant professor in the Department of Medicine at NYU Langone Health, explained in an interview with HealthITAnalytics.
“We had to figure out what would be useful for clinicians, and we finally settled down on favorable outcomes with COVID-19. We wanted to build something that would give providers a little bit of confidence that their patients will be okay.”
By analyzing over 3000 retrospective cases, the researchers developed a model that can identify hospitalized patients likely to have good outcomes with 90 percent accuracy. The model was also developed with an eye toward a straightforward integration with existing data infrastructures.
“We purposely built the model so that it could be easily deployed to every other Epic installation,” Aphinyanaphongs explained. “So rather than using all features and variables that are available to us, we took the opposite approach and considered what would be the minimum amount of data needed to make a prediction. We also had to think about how a partner organization could implement this model with minimal effort. That was probably the biggest barrier: Some things weren't designed for this use case yet, so we had to invent some new stuff to make it work.”
In their report, the researchers noted that “(t)o be effective, solutions involving machine learning must (1) address a clearly defined use case that clinical leaders will champion and (2) motivate changes in clinical management based on model predictions.”
Moreover, Aphinyanaphongs said, providers need to remain vigilant when using tools like these.
“You have to understand your patient populations and decide whether the outputs make sense for certain individuals. You see examples where the physician has some knowledge that the machine doesn't have. That's why it's all about augmentation. It's all about bringing together the AI with the knowledge that the physician has to help them make better decisions,” he said.