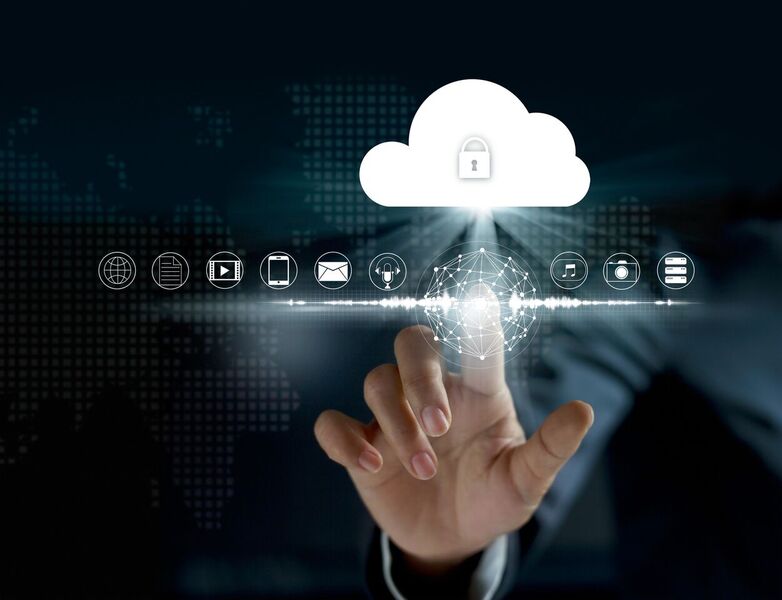
Even as AI spreads steadily across the healthcare sector, researchers continue to look for ways to address one of the most vexing problems with the emerging technology: how best to protect patient data privacy while thoroughly mining that data for critical insights.
To that end, a team of researchers from UCLA have released a study discussing their work – in conjunction with stakeholders from the State University of New York (SUNY) Upstate Medical University, and the National Cancer Institute (NCI) – on an alternative method of training AI algorithms that doesn’t rely on direct data sharing.
Dubbed “federated learning,” the method taps data from a range of institutions, then distributes training operations across all the sites.
“In federated learning (FL), models are trained simultaneously at each site and then periodically aggregated and redistributed. This approach requires only the transfer of learned model weights between institutions, thus eliminating the requirement to directly share data,” the team explained in the study.
For the study, the research team trained deep learning models at each participating institution using local clinical data, while they trained an additional model using FL across all of the institutions, thus enabling the algorithms to learn from patient data located at each of the study’s participating institutions without sharing that data.
“Because successful medical AI algorithm development requires exposure to a large quantity of data that is representative of patients across the globe, it was traditionally believed that the only way to be successful was to acquire and transfer to your local institution data originating from a wide variety of healthcare providers — a barrier that was considered insurmountable for any but the largest AI developers,” Corey Arnold, PhD, director of the Computational Diagnostics Lab at UCLA, noted in a discussion of the project.
“However, our findings demonstrate that instead, institutions can team up into AI federations and collaboratively develop innovative and valuable medical AI models that can perform just as well as those developed through the creation of massive, siloed datasets, with less risk to privacy. This could enable a significantly faster pace of innovation within the medical AI space, enabling life-saving innovations to be developed and used for patients faster.”
Moving forward, the team will aim to add an additional private fine-tuning step at each institution in order to ensure the FL model performs well at each institution in a large federation.
“The FL model that we trained performed well across all of the private datasets, yielding an overall performance level that was significantly better than that of any of the private models alone,” the team explained. “This suggests that the FL model was able to benefit from the advantage of learning important institution-specific knowledge through the FL aggregation paradigm, without requiring any individual training site to ‘see’ the full breadth of inputs.
“Additionally, our results showed that the FL model performed significantly better than any of the individual private models on the held-out challenge dataset, suggesting that the model also attained the expected advantages inherent in training with more data through the FL aggregation method, even though the full dataset was not seen at any single training site.”