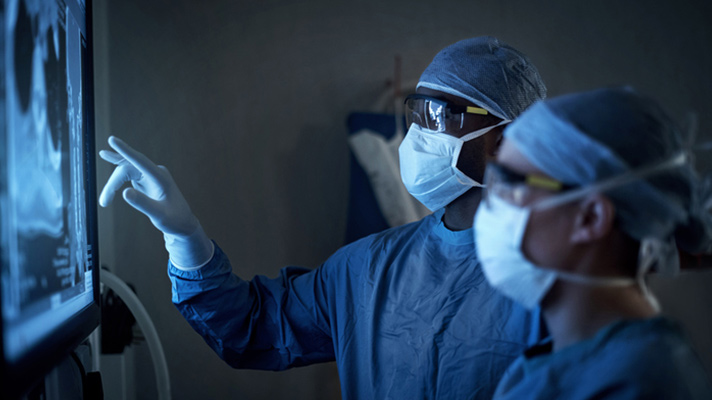
What good is the efficient use of radiology in patient diagnosis if patients aren’t able to get follow-up care in a timely fashion?
That’s the question the University of Rochester Medical Center recently tackled when it implemented an AI-driven tracking system the goal of which, Bill Siwicki recently reported at HealthcareIT News, was “to serve as a safety net for patients for whom clinicians had identified a potential malignancy or aneurysm and offered an actionable recommendation.”
As Dr. Ben Wandtke, medical staff president and chief of diagnostic imaging at UR Medicine Thompson Health and an associate professor in the department of imaging sciences at the University of Rochester Medical Center, explained, “While a majority of incidental radiology findings are, thankfully, benign nodules, others turn out to be treatable forms of cancer. “Unfortunately, due to gaps in communication, patients can fall through the cracks and not get into the proper cancer treatment right away.”
The good news for Rochester’s patients was how quickly the pilot program yielded results.
“In the first 13 months, we tracked 589 recommendations, 86% of which were satisfactorily closed through the program, reducing the risk of delayed diagnosis by 74%,” Wandtke reported. “We subsequently expanded (the program) to include six hospitals and 75 radiologists.”
Interestingly, the program didn’t initially include an AI element, but the Center’s staff soon recognized that a largely manual process of identifying recommendations was going to impede widespread adoption of the program.
“Our facilities conduct more than 800,000 diagnostic imaging exams annually, and our radiologists were too busy to consistently remember to add all of their recommendations to the database,” Wandtke explained. “We needed a way to automate this process to improve the effectiveness of our safety net.”
The Center then incorporated a Natural Language Processing-based clinical analytics solution into their existing program, enabling them to tap AI algorithms to help identify and track more of the recommendations coming out of the department.
Moreover, said Wandtke, “the analytics is used to provide a report detailing the frequency and quality of recommendations generated to individual radiologists and leadership to improve the quality of recommendations radiologists include in their reports.”
The result of the changes, Wandtke reported, is that the “University of Rochester now tracks more than 500 recommendations per month (0.8% of diagnostic volume), and 91% of these recommendations are satisfactorily closed.”
More tellingly, he said, 55% of those recommendations tracked have been identified only by the NLP analytics.