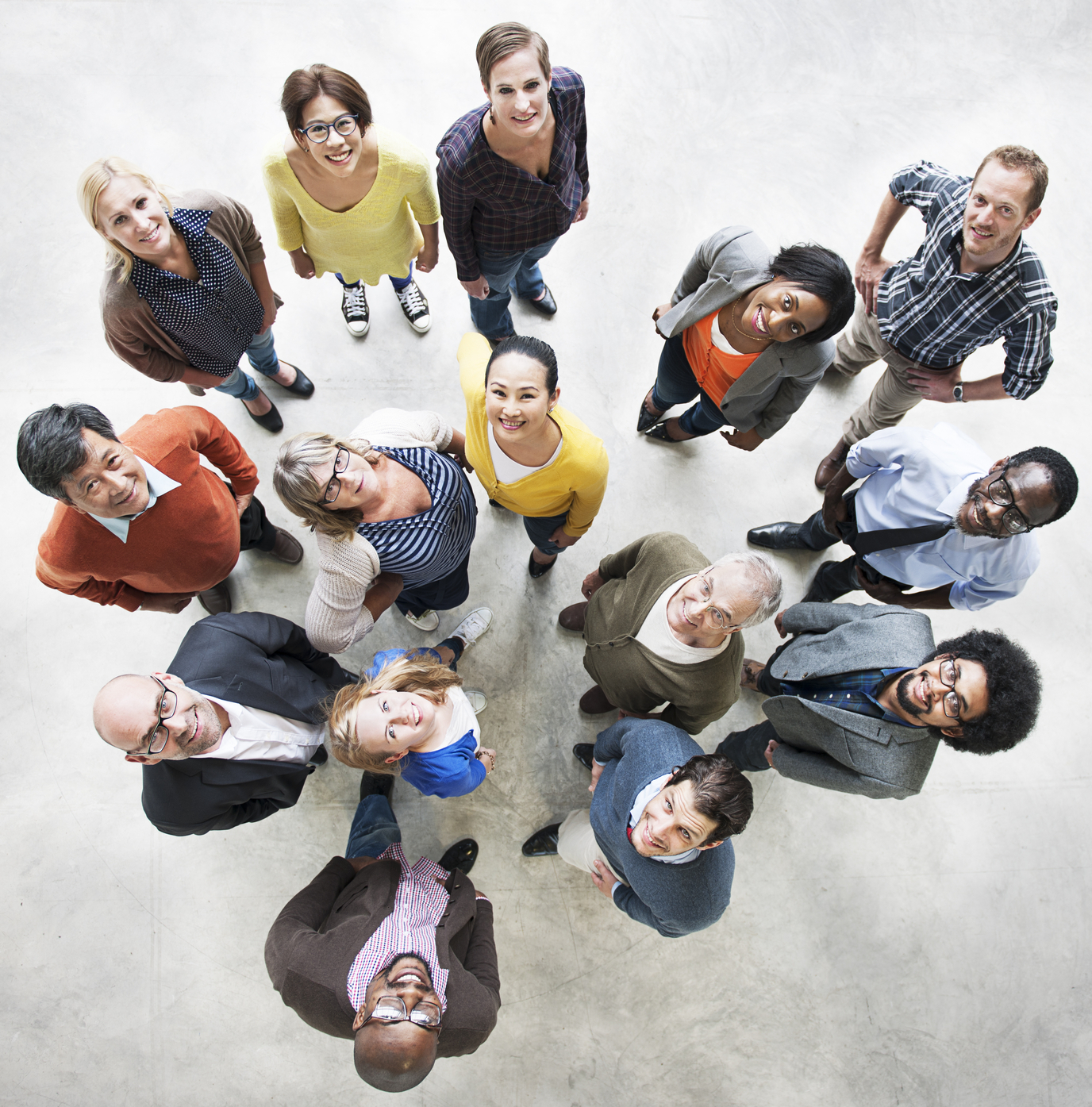
Coughing and sneezing are common sounds in any cold-weather season, but in recent months they’ve taken on new significance as the coronavirus has raced around the world.
A new portable surveillance device powered by machine learning may be just in time, therefore, to help healthcare providers step up efforts to detect coughs and analyze data to monitor flu-like illnesses and influenza trends more closely.
Developed by two UMass Amherst researchers, the FluSense platform is intended to be used in hospitals, healthcare waiting rooms and larger public spaces with an eye toward helping determine the timing for flu vaccine campaigns, potential travel restrictions and the allocation of medical supplies, among other things.
“This may allow us to predict flu trends in a much more accurate manner,” said Tauhidur Rahman, assistant professor of computer and information sciences, in a press release.
The FluSense platform processes a low-cost microphone array and thermal imaging data with a Raspberry Pi and neural computing engine. It stores no personally identifiable information, such as speech data or distinguishing images. In Rahman’s UMass lab, where computer scientists develop sensors to observe human health and behavior, the researchers first developed a lab-based cough model. Then they trained the deep neural network classifier to draw bounding boxes on thermal images representing people, and then to count them.
“Our main goal was to build predictive models at the population level, not the individual level,” Rahman said.
From December 2018 to July 2019, the FluSense devices, which were encased in a rectangular box about the size of a large dictionary and placed four healthcare waiting rooms at UMass’s University Health Services clinic, collected and analyzed more than 350,000 thermal images and 21 million non-speech audio samples from the public waiting areas.
The researchers found that FluSense was able to accurately predict daily illness rates at the university clinic. Multiple and complementary sets of FluSense signals “strongly correlated” with laboratory-based testing for flu-like illnesses and influenza itself.
“I’ve been interested in non-speech body sounds for a long time,” Rahman explained. “I thought if we could capture coughing or sneezing sounds from public spaces where a lot of people naturally congregate, we could utilize this information as a new source of data for predicting epidemiologic trends.”
According to Ph.D. student and lead author Forsad Al Hossai, FluSense is an example of the power of combining artificial intelligence with edge computing, the frontier-pushing trend that enables data to be gathered and analyzed right at the data’s source.
“We are trying to bring machine-learning systems to the edge,” Al Hossain said, pointing to the compact components inside the FluSense device. “All of the processing happens right here. These systems are becoming cheaper and more powerful.”
The next step is to test FluSense in other public areas and geographic locations.