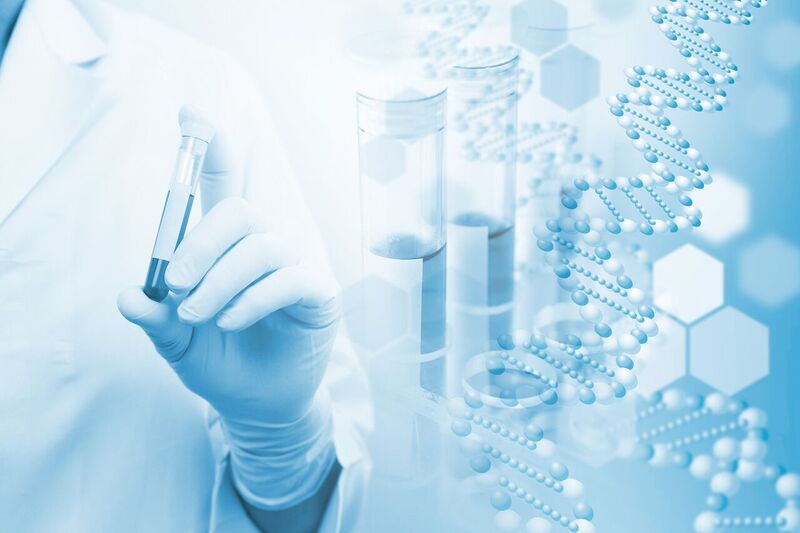
Developments in AI present exciting possibilities across the healthcare sector, but given the cost of developing new drugs, one could argue that the pharmaceutical industry has the most the gain from potential AI efficiencies.
At the same time, like every other healthcare stakeholder, Big Pharma decision makers have to determine what’s possible with AI from what is simply hype.
As Gabe Musso, Chief Scientific Officer of BioSymetrics, put it in a recent industry interview,
“every executive in the pharmaceutical industry has seen the landscape change to the point where they know that AI and ML (Machine Learning) approaches are going to impact their future business. However, there is still much noise; identifying what is likely to be a successful implementation of AI/ML and how it will impact your business practices is undeniably tricky.”
Not surprisingly, Musso pointed out that most executives approach AI trials by starting “with small projects, either on the commercialization side or research side, trying to understand, in a very measured way, how AI or ML can impact the products they are seeking to get to market.”
At the same time, that ostensibly sensible approach might be counter-productive, Musso suggests, given that the true value of machine learning often “isn't seen until applied on much larger and more encompassing datasets. It does require multiple data sets and cross-disciplinary engagements to see the benefit in ML.”
As to the best place for pharma firms to plug into AI, Musso says “pre-clinical stages appear to provide the best business value. The resources required to screen through a large library of compounds or diagnostics get compounded if you get it wrong based on an informed hunch. ML provides the opportunity to remove our human perspective and allow a more unbiased decision-making process.”
And then there’s the need to strike the inevitable balance between machine and human, Musso says, as companies will never be able to depend solely on AI and ML. For example, he notes, “There will always be a human element, particularly around data collection and patient care. Healthcare is an intimately human experience; we cannot discount human intervention and oversight.”
In short, as with pretty much every other part of healthcare, Musso assumes the next few years are going to be about “finding that balance between how much we want the AI to play a role in the decision-making process and what remains human regulated. Just as the automotive industry is evolving from adaptive cruise control and lane assist to the prospect of self-driving cars, the healthcare community will need to have a similar progression. However, we sense that there will always be a driver behind the wheel.”