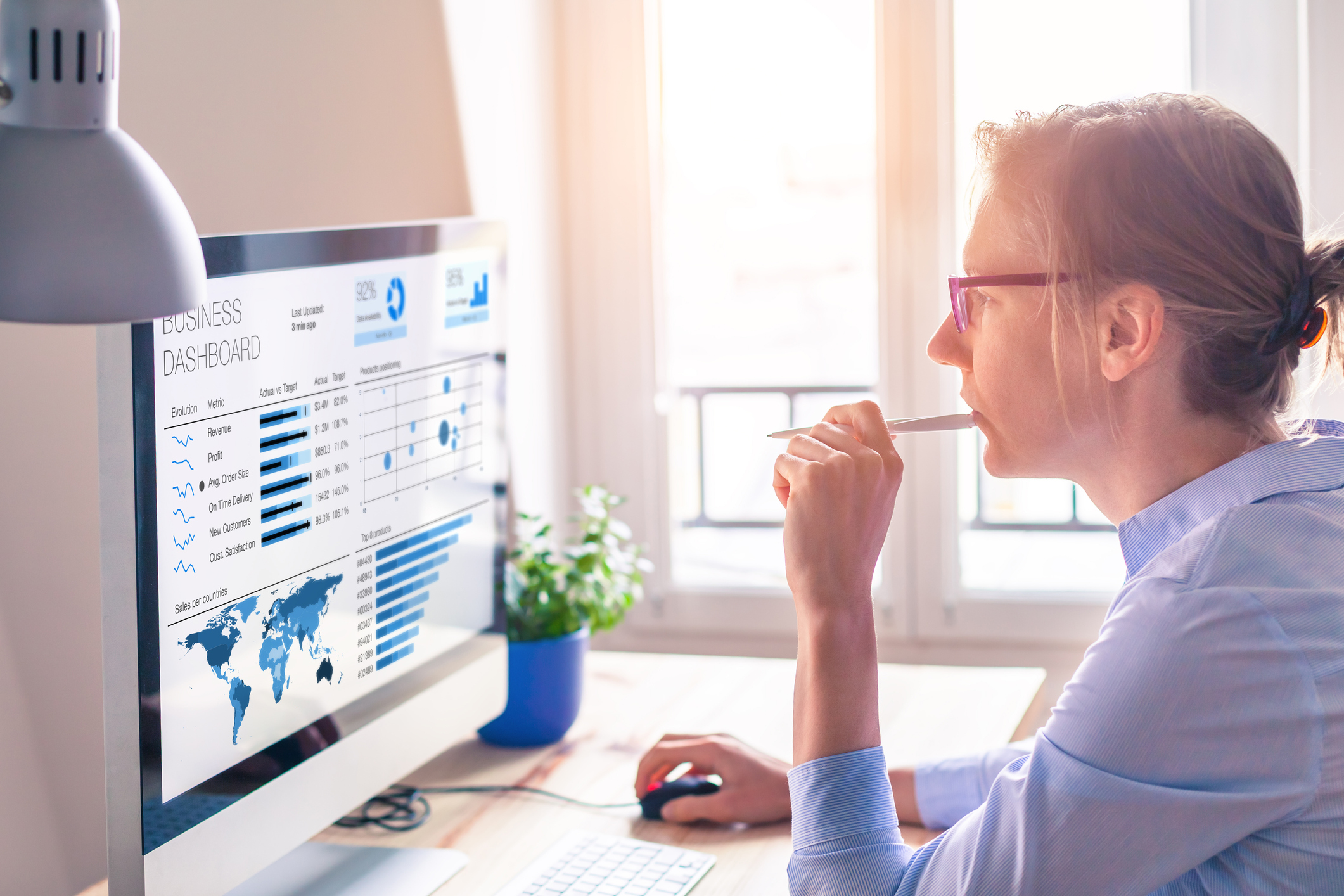
The Penn Medicine Institute for Biomedical Informatics recently announced the launch of a free, open-source automated machine learning system designed to simplify data analysis.
The tool, dubbed PennAI, was developed with funding from the National Institutes of Health to constantly learn the best approaches for analyzing data and to provide recommendations to users.
The artificial intelligence engine behind the platform can work out different analyses with different variables and methods on its own, and by making Penn AI’s analysis open source, it allows researchers to see the mechanisms behind each analysis.
“The problem with machine learning tools is that machine learning people build them, so they’re usually only usable by those with high levels of training,” Jason Moore, director of the Institute for Biomedical Informatics, said in a statement.
As Penn AI is used more and more, it will continually learn the best methods for analyzing data and will provide recommendations for its users based on what they are looking to find out.
Moore explained the development team’s goal was to make a free and simple system that was still robust enough to transform the way the industry approaches biomedical research, and over the three-year development period the system was built to be approachable by anyone, regardless of training or experience. As a self-service, clinical platform, it will be possible for a doctor to query associations between sex, age, smoking and different diseases, and then have the platform answer their questions.
“I think this is really going to accelerate biomedical research,” Moore noted. “We’ll be able to do almost instantly what it takes weeks and months—and thousands or millions of dollars—to do now.”
Moreover, Moore said that by making this kind of analysis open source, Penn AI addresses the “black box” phenomenon by enabling physicians to understand internal workings of the tool and how it arrived at the results.
Future versions of the platform could include more complex features for advanced users, like the addition of “ensemble approaches”, a technique that allows multiple machine learning apparatuses to work on the same dataset at the same time in order to develop a more robust analysis.