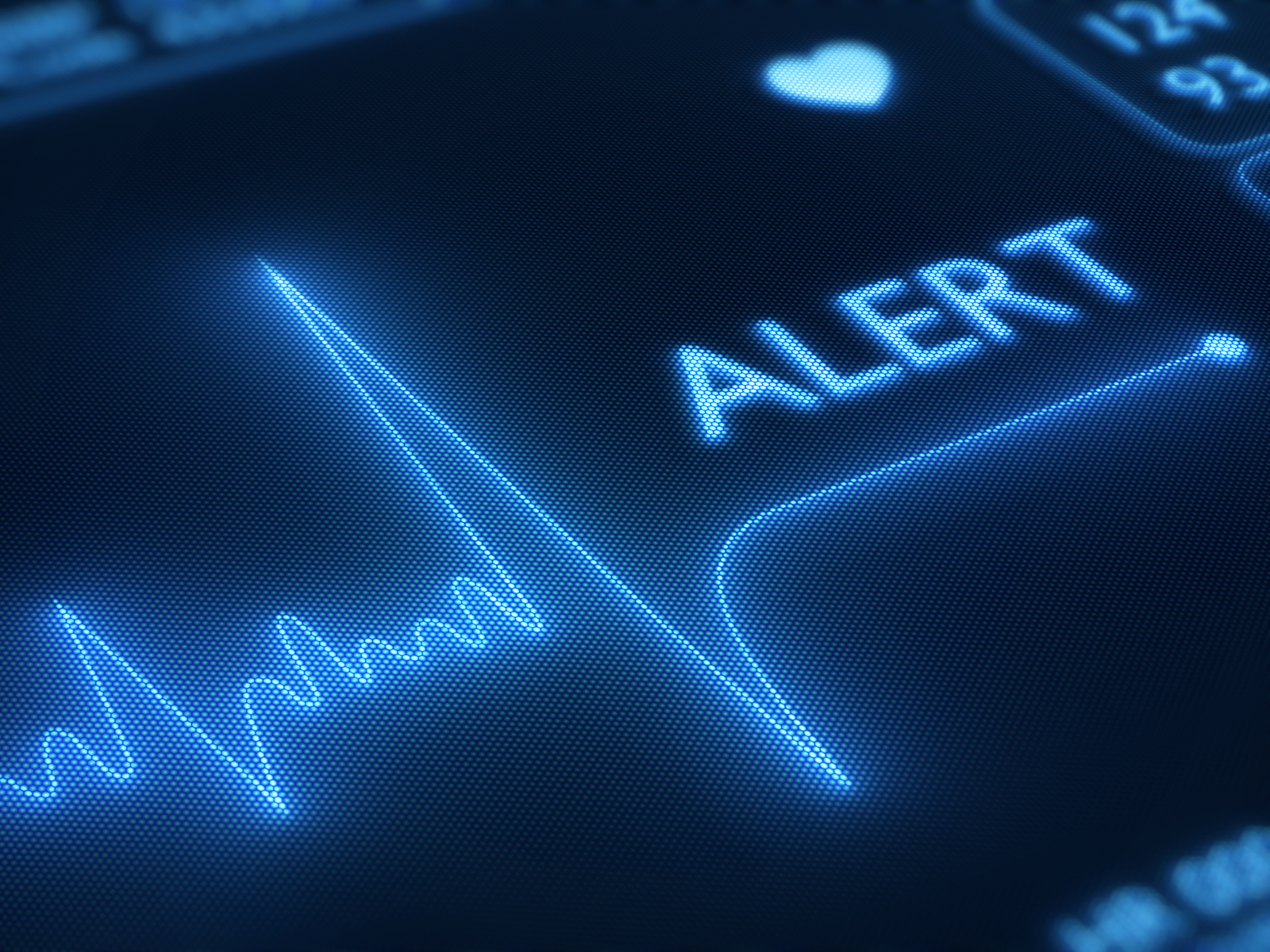
Atrial fibrillation (AF) is a common heart condition that can lead to stroke-causing blood clots, and researchers at MIT and Mass General Hospital have turned to AI to help develop a new method of identifying patients who are at risk for the condition.
“Identification of individuals at elevated AF risk is a clinical imperative since modifying lifestyle and behavioral factors may prevent AF, and since cardiac rhythm monitoring may identify individuals with undiagnosed AF thereby enabling prevention of strokes,” the researchers wrote in their report recently published in Circulation, a journal of the American Heart Association.
According to reports, the investigators developed the AI-based method to predict the risk of AF within the next five years based on results from electrocardiograms (noninvasive tests that record the electrical signals of the heart) in 45,770 patients receiving primary care at Mass General.
“Baseline age, sex, race, height, weight, and blood pressure values were obtained from the EHR,” the researchers wrote in their report. Anti- hypertensive use was determined using medication lists. Tobacco use was categorized as present or absent. Race was classified as white or non-white . . . The presence of heart failure, diabetes, and myocardial infarction were ascertained using previously published sets of diagnostic codes and medications . . .”
Next, the scientists applied their method to three large data sets from studies including a total of 83,162 individuals. The AI-based method predicted atrial fibrillation risk on its own and was synergistic when combined with known clinical risk factors for predicting atrial fibrillation. The method was also highly predictive in subsets of individuals such as those with prior heart failure or stroke.
“We see a role for electrocardiogram-based artificial intelligence algorithms to assist with the identification of individuals at greatest risk for atrial fibrillation,” said senior author Steven A. Lubitz, a cardiac electrophysiologist at MGH, an associate member at the Broad Institute of MIT and Harvard, and associate professor of medicine at Harvard Medical School.
“Our findings demonstrate that deep learning models utilizing ECG to estimate AF risk are robust and valid across contrasting populations when assessed using rigorous epidemiologic metrics,” the researchers noted in their report, while Lubitz explained that the algorithm could serve as a form of pre-screening tool for patients who may currently be experiencing undetected atrial fibrillation.
Added co-author Anthony Philippakis, chief data officer at the Broad and co-director of the institute’s Eric and Wendy Schmidt Center, “With the explosion of data science technologies and the vast amounts of clinical data now available, machine learning is poised to help clinicians and researchers make great strides in enhancing cardiology care. As a data scientist and former cardiologist, I’m excited to see how machine learning–based methods can work with the tests and clinical approaches we use every day to help us improve risk prediction and take care of patients with atrial fibrillation.”
Photo by johan63/Getty Images