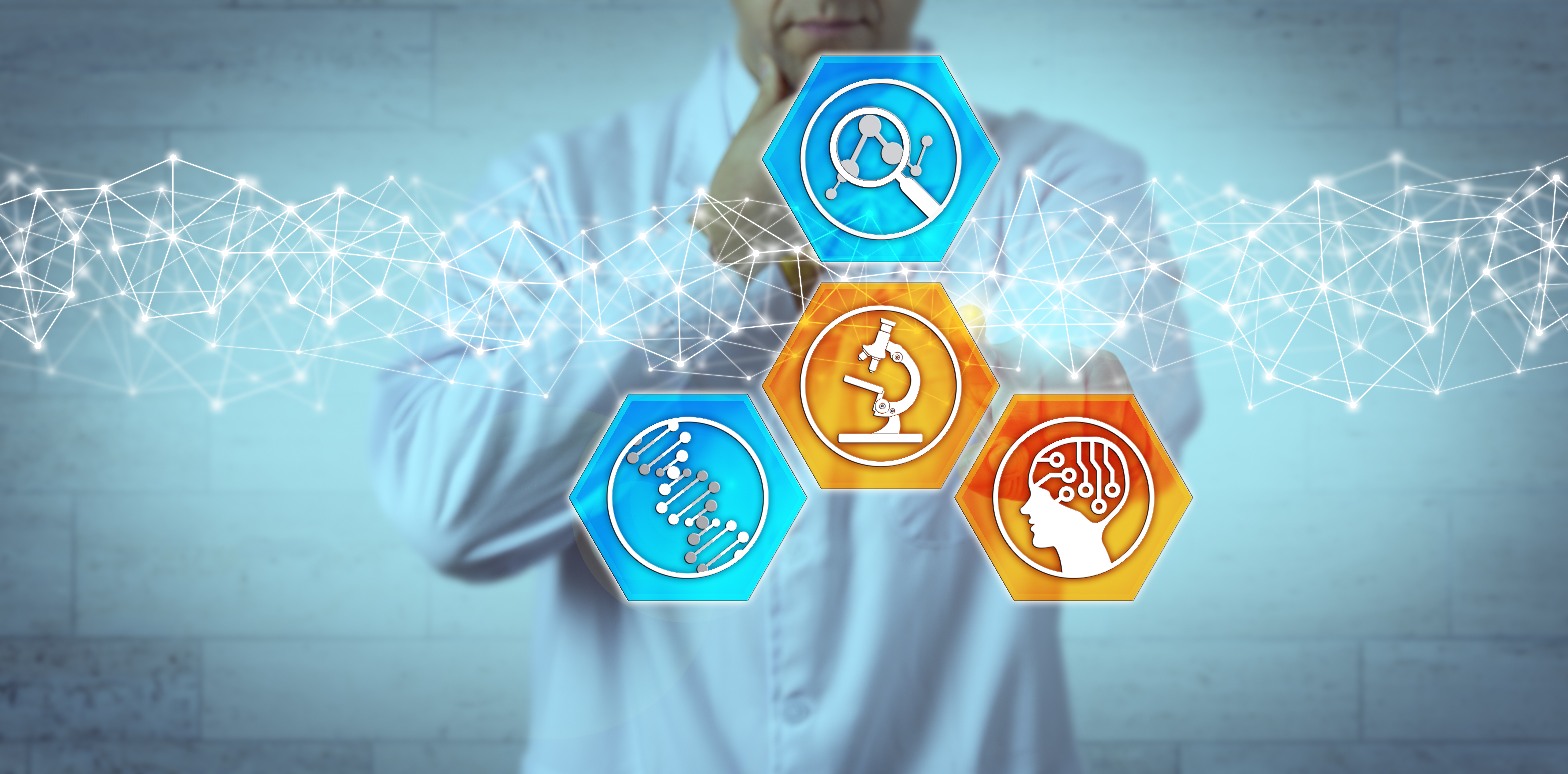
One of the many vexing attributes of COVID-19 is the fact that it manifests in patients in variable and unpredictable ways.
But healthcare teams may soon be able to better predict whether a patient’s condition is going to improve or deteriorate with a new algorithm developed by a team of researchers from Michigan Medicine and published in British Journal of Anaesthesia.
According to the study, “Machine learning techniques can be leveraged to improve the ability to predict which patients with COVID-19 are likely to require mechanical ventilation, identifying unrecognized bellwethers and providing insight into the constellation of accompanying signs of respiratory failure in COVID-19.’’
As Nicholas J. Douville, M.D., Ph.D., of Michigan Medicine’s Department of Anesthesiology and one of the study’s lead authors, explained the problem, “You can see large variability in how different patients with COVID-19 do, even among close relatives with similar environments and genetic risk. At the peak of the surge, it was very difficult for clinicians to know how to plan and allocate resources.”
To develop the algorithm, researchers looked at a set of patients with COVID-19 hospitalized during the first pandemic surge from March to May 2020 and modeled their clinical course using inputs such as a patient’s age, whether they had underlying medical conditions and what medications they were on when entering the hospital. They also factored in variables that changed while hospitalized, such as vital signs like blood pressure, heart rate,and oxygenation ratio. The goal was to discover which of these data points would help best predict which patients would require a mechanical ventilator or die within 24 hours.
The model was able to predict mechanical ventilation most accurately based on key vital signs, including oxygen saturation ratio, respiratory rate, heart rate, blood pressure, and blood glucose level. The team examined data points at four-, eight-, 24-, and 48-hour increments to identify the optimal amount of time necessary to predict and intervene before a patient deteriorates.
While the algorithm worked best at shorter increments, researchers noted that the model maintained accuracy even two days before an adverse event
“The closer we were to the event, the higher our ability to predict, which we expected. But we were still able to predict the outcomes with good discrimination at 48 hours, giving providers time to make alterations to the patient’s care or to mobilize resources,” said Douville.
Going forward, the team expect that the algorithm can be integrated into existing clinical decision support tool already used in the ICU. In the short term, the study highlights patient characteristics that clinicians should consider when treating patients with COVID-19.