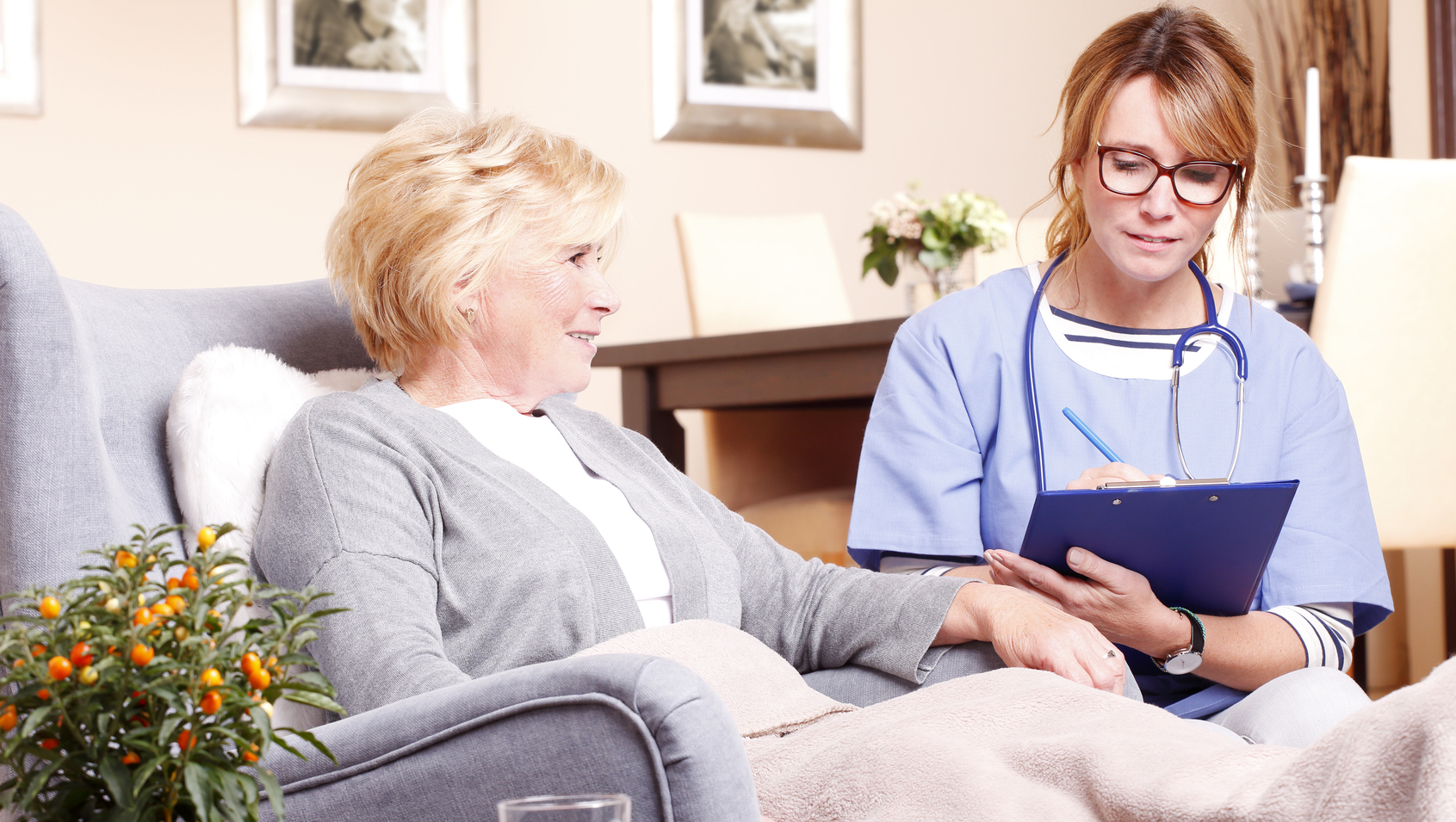
New AI helps improve the efficiency and accuracy of an advanced imaging technology used to screen for breast cancer.
That’s according to a new study published in the journal Radiology: Artificial Intelligence, for which a model was developed that read and interpreted the findings of digital breast tomosynthesis (DBT) images.
DBT is an advanced method for cancer detection in which an X-ray arm sweeps over the breast, taking multiple images in a matter of seconds. Previous research has shown that DBT improves cancer detection and reduces false-positive recalls compared to screening with digital mammography (DM) alone.
For the study, researchers developed a deep learning system to help mine vast amounts of data to find subtle patterns beyond human recognition. They trained the AI system on large DBT data sets to identify suspicious findings in the DBT images.
After developing and training the system, the researchers tested its performance by having 24 radiologists, including 13 breast subspecialists, each read 260 DBT examinations with and without AI assistance. The examinations included 65 cancer cases.
"Overall, readers were able to increase their sensitivity by 8 percent, lower their recall rate by 7 percent and cut their reading time in half when using AI concurrently while reading DBT cases compared to reading without using AI," said study lead author Emily F. Conant, M.D., professor and chief of breast imaging from the Department of Radiology at the Perelman School of Medicine at the University of Pennsylvania in Philadelphia, in a statement.
The accuracy of the deep learning system depends on the size of the datasets it is fed, so improved efficacy will increase the technology’s usefulness in clinical practice by continuing to cut down reading times and false-positive rates. Moreover, despite both the improved accuracy and decreased false-positive readings, DBT tests are not widely adopted as a part of the standard of care primarily because the results take longer to interpret. The multiple images captured in a DBT screening test require radiologists to spend more time looking at each picture before drawing conclusions.
“The results of this study suggest that both improved efficiency and accuracy could be achieved in clinical practice using an effective AI system,” said Conant.
Employing AI strategies that cut down reading time and improve the tests accuracy could help support the argument of DBT’s adoption as a part of the standard of care.
“We know that DBT imaging increases cancer detection and lowers recall rate when added to 2-D mammography and even further improvement in these key metrics is clinically very important,” Conant said. “Since adding DBT to the 2-D mammogram approximately doubles radiologist reading time, the concurrent use of AI with DBT increases cancer detection and may bring reading times back to about the time it takes to read DM-alone exams.”