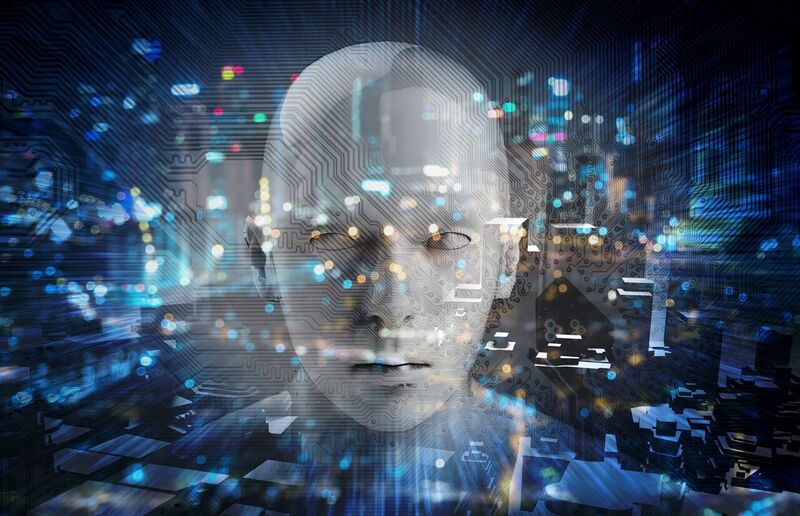
As AI is implemented across the healthcare sector, stakeholders and researchers are taking increasing steps to ensure that the data used in the algorithms that are the engine for AI are as “clean” as possible when it comes to possible bias.
To that end, researchers at MIT have have worked to develop a new deep learning system that is able to predict a patient’s cancer risk leveraging only the person’s mammograms, that reportedly works equally effectively regardless of race or ethnicity.
Dubbed “Mirai,” a recent announcement from MIT noted that the algorithm is able to model “a patient’s risk across multiple future time points,” while taking into account minor variances, down to the brand of mammogram machine the clinic uses. Its predictions can be further optimized if other clinical risk factors -- such as age or family history -- are available.
The CSAIL team initially trained Mirai, which is now installed at Massachusetts General Hospital (MGH), on a 200,000-exam dataset from that organizations= before validating its predictive results on additional sets from the Karolinska Institute in Sweden, and Chang Gung Memorial Hospital in Taiwan.
According to MIT, “Mirai was significantly more accurate than prior methods in predicting cancer risk and identifying high-risk groups across all three datasets.” It is also able to identify nearly twice as many potential cancer cases among high risk groups as the currently employed diagnostic Tyrer-Cuzick model.
“Improved breast cancer risk models enable targeted screening strategies that achieve earlier detection, and less screening harm than existing guidelines,” Adam Yala, CSAIL lead author of the paper concerning Mirai that has been published in Science Translational Medicine. “Our goal is to make these advances part of the standard of care.”
Despite the wide adoption of breast cancer screening, the practice has been not been without controversy. According to the researchers, “more-aggressive screening strategies aim to maximize the benefits of early detection, whereas less-frequent screenings aim to reduce false positives, anxiety, and costs for those who will never even develop breast cancer . . . . Despite decades of effort, the accuracy of risk models used in clinical practice remains modest.”
To ensure that Mirai’s recommendations were consistent, the CSAIL team de-biased the algorithm by running it through an “adversarial” network to differentiate between aspects of the mammogram which are important and those that may be caused by minor random environmental variances.
The team also analyzed the model’s performance across races, ages, and breast density categories in the MGH test set, and across cancer subtypes on the Karolinska dataset, and found it performed similarly across all subgroups.