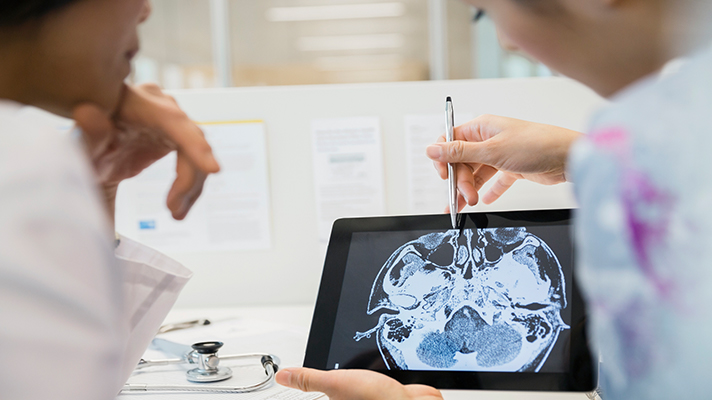
Ultrasound, first used for clinical purposes in 1956, is witnessing major advancements for obstetrics care. These advances range from finely grained imaging to show blood moving through a fetal heart to 3D and 4D visualizations to help surgeons plan prenatal surgeries. Now, the addition of AI and machine learning is promising to assist every radiologist with the early detection of medical issues inside the womb.
Boston Children’s Hospital, the primary pediatric teaching affiliate of Harvard Medical School, is creating an AI tool to see a side-by-side comparison of age-matched normal childhood brain images along with patients’ scans. The collaboration with GE Healthcare seeks to develop and commercialize digital solutions to advance the diagnosis and treatment of specific childhood diseases, starting with brain diseases.
Boston Children’s conducts nearly 1,000 imaging studies each day, making it an ideal setting to implement a decision-support tool that can distinguish the large variability in brain MRI scans. In the case of developing prenatal and young brains, abnormal but symmetrical conditions can be missed; conversely, normal, expected changes can be misinterpreted as pathologic, leading to unnecessary follow-up imaging or other diagnostic tests.
To start, the model will answer a binary question, “normal or abnormal,” said Richard Robertson, MD, radiologist-in-chief at Boston Children’s. In operation, a future GE Healthcare ultrasound machine will be pre-loaded with normative reference scans from young children of different ages for doctors worldwide to use as a benchmark when reading scans of pediatric patients. It will recognize which scans are possibly abnormal and need to be viewed by a radiologist for further consideration. “But one can imagine down the line an assessment of a condition,” Robertson said.
An important goal of the project, which was described[1] at the 102nd annual meeting of the Radiological Society of North America in Chicago, is to bring insight to locations lacking expertise or access to data. Most pediatric imaging is not performed in children’s hospitals by specialists, so “providing decision support at the time of interpretation to non-specialists will improve both the confidence and performance of the interpreting radiologist," he noted.
Much of the work at Boston Children’s focuses on building the data set, added Robertson, who described the project as still in-process. “There are a lot of steps before we get to the exciting stuff, the building of the model,” he said.
Normalizing data
That preparatory work will require a great deal of data cleaning and tagging, not only of the image scans but of other clinical and patient data, according to experts.
“There are a lot of implications with regards to looking not only looking at the fetus but also the mother” to predict, for example, women at higher risk for preterm delivery, said Safwan S. Halabi, MD, a clinical assistant professor at the Stanford University School of Medicine and medical director for radiology informatics at Stanford Children’s Health.
While bone-age, lung nodule and cranial bleed decision-support tools have made their way into clinical practice, “ultrasound is tough because there isn’t a lot of labeled data, and because the image acquisition is very operator dependent,” Halibi said. In other words, ultrasound image data isn’t nearly as normalized (in the form of curated data sets) as other radiological modalities.
Nevertheless, Halabi sees enormous potential for AI to augment what obstetricians do daily. “A pregnant woman, instead of having to drive four hours to a tertiary care center, could take a scan nearby, or even at home,” he said. Since many of the AI models will be hosted on the cloud, “there’s going to be this connection between modality or device, to data transfer, to the cloud.”
In order for such systems to enter clinical practice, both Halabi and Robertson said physician trust in the models will be paramount. “It’s been shown that images from different systems react differently with the same [AI] model,” Halabi said. “There is a huge training process that requires supervised learning or some type of annotated data.”
GE Healthcare is stepping up to that challenge, especially when it comes to leveraging AI and deep learning in Ultrasound, to enable better care of mothers and their unborn babies. “Take SonoCNS on GE Healthcare’s Voluson Ultrasound, for example. Here is an application that leverages deep learning to help detect brain abnormalities early in the second trimester.” said Roland Rott, GM of Women's Health Ultrasound at GE Healthcare.
Rott went on to say, “what is equally exciting is we are already hearing that the benefits can go beyond a single doctor to a single patient interaction. Customers are embracing the technology in amazing ways to expand access to their services to do more with less, while also potentially improving quality, especially in areas of the world that present geographical challenges. We are still in early days with AI in Ultrasound, but with AI now embedded across our Ultrasound portfolio, we are really excited to see the impact for our customers and their patients at a global scale.”
[1] https://www3.gehealthcare.com/~/media/rsna-2016-press-kit-assets/press releases/press release_bch and gehc working together to create smart imaging technology to better detect pediatric brain disorders.pdf?Parent={1FFF8A39-2AC6-4EA6-9119-7458819CB4F7}