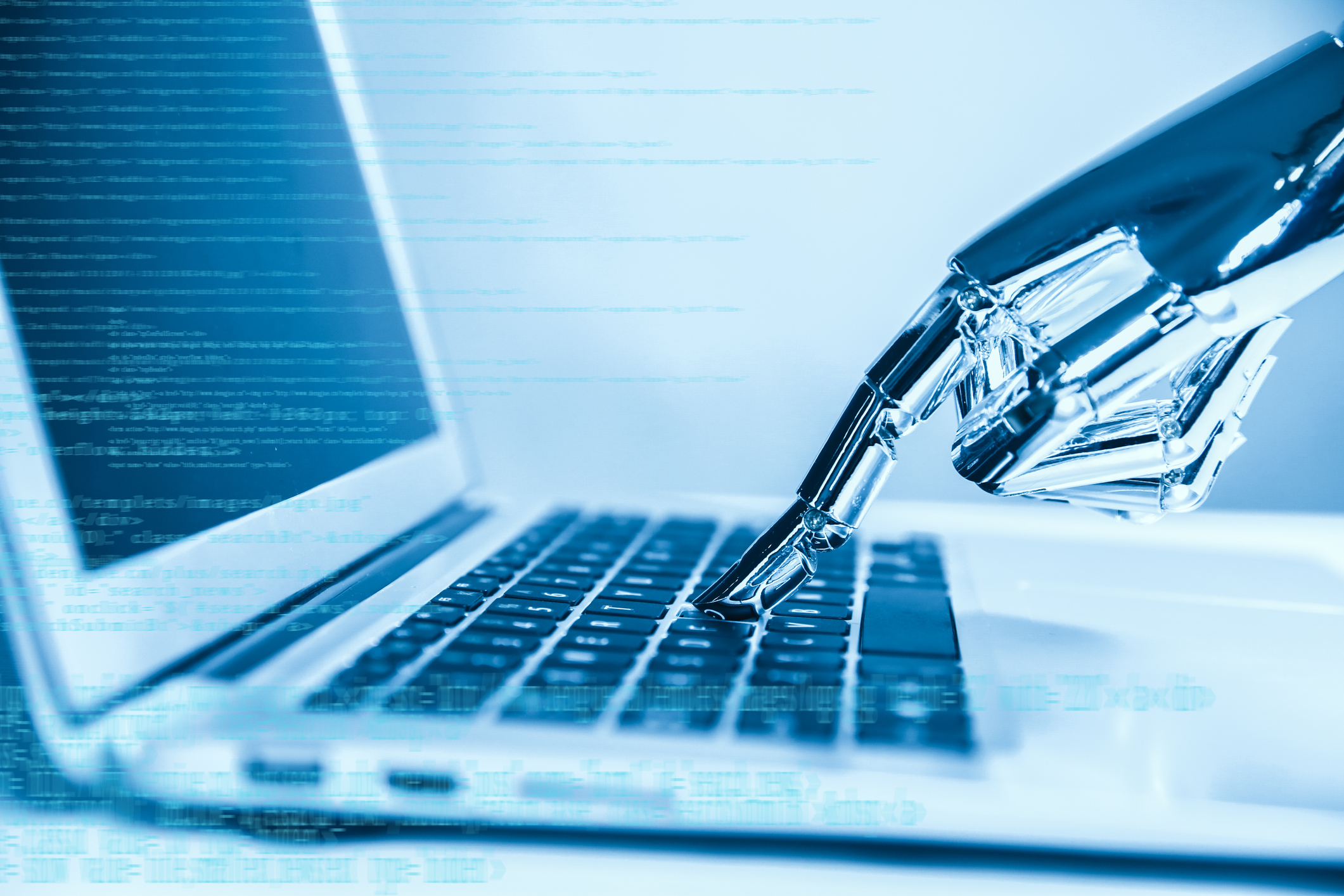
When does a problem become an opportunity? When there’s a better way of organizing it.
That’s one way of looking at the emergence of Artificial Intelligence (AI) in healthcare, tech writer Nick Ismail recently suggested in a piece at Information Age.
“Of all the industries set to be impacted by artificial intelligence and machine learning,” he argued, “the healthcare sector stands to gain the most. Often hindered by legacy infrastructure and disparate data sets from hundreds, or thousands of different sources, healthcare needs AI.”
Indeed, according to Ian Roberts, CTO at Healx, a UK-based bio-tech and research firm, “AI is set to integrate the data collected and provide ever improving treatments for patients from the process of drug discovery to providing personalized therapeutics, while considering the individual’s diagnostic data. This is particularly exciting due to the fact that the costs and time associated in all parts of the process can be cut down dramatically, due to the relatively cheap nature of modern computation (when compared to current model of healthcare systems). With the current model, healthcare systems around the world are not sustainable and AI has the potential to change that to democratize medicine once again.”
As Ismail sees the near term future, “AI systems will appear at an increasing rate throughout healthcare processes — whether it is in diagnosis diabetic eye disease at the Moorlands Eye Clinic, or assisting surgery with Robots like Da Vinci Surgical System.”
Going even further, Ian Roberts predicts AI will be fully integrated into the UK’s healthcare system in ten years, and “once this happens, preventative medicine will become more prevalent in protective treatments before the onset of life-threatening conditions. This will also cut costs in the long term."
In addition to the cost-cutting potential, Roberts points to the numerous ways AI will support medical research.
“There are several layers of data which no one can integrate without AI,” he said. “We have academic papers covering basic research, diagnostic omics data, immunology markers, compounds structures, as well as patients’ personal reports about their condition and the treatments they tried. Using machine learning algorithms, the technology can be used to read, analyze and correlate and integrate data at a much faster rate, with ever improving accuracy. Patterns and relationships are identified to discover new targets, drug entities or repurposing of drugs already on the market in order to treat rare diseases in unexpected ways.”
To be sure, there remain some big challenges, particularly since most of the currently available data is unstructured, and needs to be optimized for AI mining.
But as Roberts sees it, “Yes, these challenges might slow us down or make our work harder but knowing that rare disease patients don’t have ANY treatment available to ease their suffering makes it easy for us to keep on going despite the difficulties.”