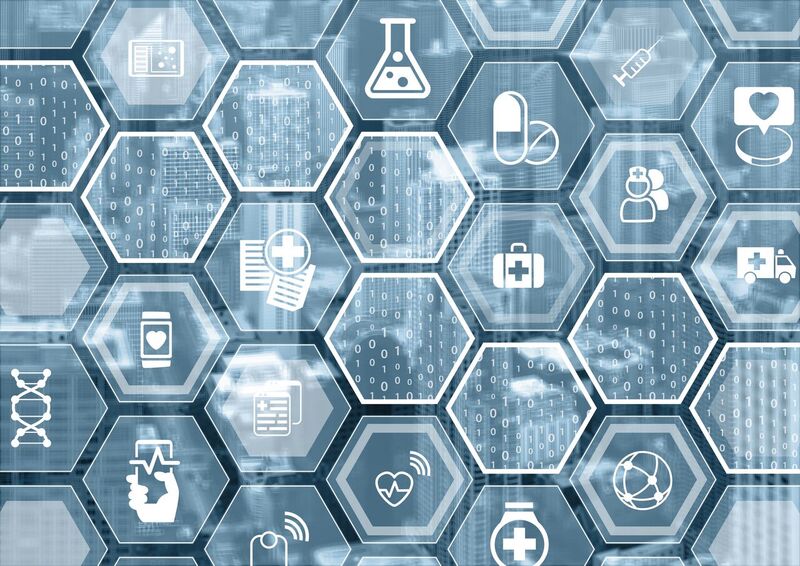
Use of AI in healthcare around the globe is expected to grow exponentially in the next few years, but healthcare stakeholders still struggle with how best to use it.
That sums up the problem three stakeholders with data science and healthcare research giant IQVIA aim to help solve in a recent commentary that offers five ways AI can contribute right now to clinical operational efficiency.
Interestingly, they look first behind the scenes of healthcare, so to speak, by observing that successful clinical trials rely on effective study design. To that end, they note, “(l)everaging vast healthcare data sets, AI, ML and natural language processing tools can be used to assess and select optimal primary and secondary endpoints during study design to ensure the most relevant protocols are defined for regulators, payers and patients. This helps to optimize the study design by informing ideal strategies for host countries and sites, enrollment models, patient recruitment and start-up plans.”
On a related note, the next opportunity where AI can help is in identifying sites and patients – meaning the right ones and a big enough number – also to help ensure successful trials. “Identifying trial sites that have access to enough patients who meet inclusion/exclusion criteria is an ongoing challenge,” they observe, but “AI and ML can mitigate these risks by identifying and suggesting the sites with the highest recruitment potential and suggesting appropriate recruitment strategies. This involves mapping patient populations and proactively targeting sites with high predicted potential to deliver the most patients — before a single site is opened — and identifying the best avenues to recruit them.”
Next on their list for areas AI can help is what they dub “pharmacovigilance.” Specifically, AI and ML tools “can automate manual processing tasks and translate and digitize case safety reporting and adverse drug reaction documents. They can also monitor digital conversations on social media and other platforms to ensure that adverse events are promptly identified.”
Another challenge they point to in clinical tests involves analyzing and working to mitigate site risks, and “AL and ML concepts can alleviate these pressures by assessing the risk environment and delivering predictive analytics to generate more effective clinical monitoring insights. . . Evaluations can also be used to proactively identify which sites are more likely to have recruitment and performance issues, or which patients are at higher risk for potential AEs. These insights facilitate faster action and avoid potential problems.”
Finally, there’s the patient care that is an integral part of clinical tests. “Disease detection algorithms are now being designed to leverage medical information, such as symptoms and procedures that typically precede a diagnosis, to identify patients who are very likely to develop diseases,” the writers explain. “This allows for proactive care, as well as new recruiting insights for prodromal or early-stage disease studies and those that require treatment-naive patients.”
The bottom line for these stakeholders is that fact that, as they sum it up, “(t)he clinical development landscape is changing quickly, and sponsors need to have the best insights to make the right decisions to increase predictability, reduce time to market and improve efficiencies.”
With that goal in mind, AI and related technologies aren’t going to replace the need for human oversight, but “they are going to accelerate the ability to analyze the data and take meaningful action in response to create a safer, more streamlined research environment.”